Performance Characterization of High-Level Programming Models for GPU Graph Analytics
IEEE International Symposium on Workload Characterization(2015)
摘要
We identify several factors that are critical to high-performance GPU graph analytics: efficient building block operators, synchronization and data movement, workload distribution and load balancing, and memory access patterns. We analyze the impact of these critical factors through three GPU graph analytic frameworks, Gun rock, Map Graph, and VertexAPI2. We also examine their effect on different workloads: four common graph primitives from multiple graph application domains, evaluated through real-world and synthetic graphs. We show that efficient building block operators enable more powerful operations for fast information propagation and result in fewer device kernel invocations, less data movement, and fewer global synchronizations, and thus are key focus areas for efficient large-scale graph analytics on the GPU.
更多查看译文
关键词
high-level programming models,identify several factors,high-performance GPU graph analytics,building block operators,synchronization,data movement,workload distribution,load balancing,memory access patterns,gun rock,map graph,VertexAPI2
AI 理解论文
溯源树
样例
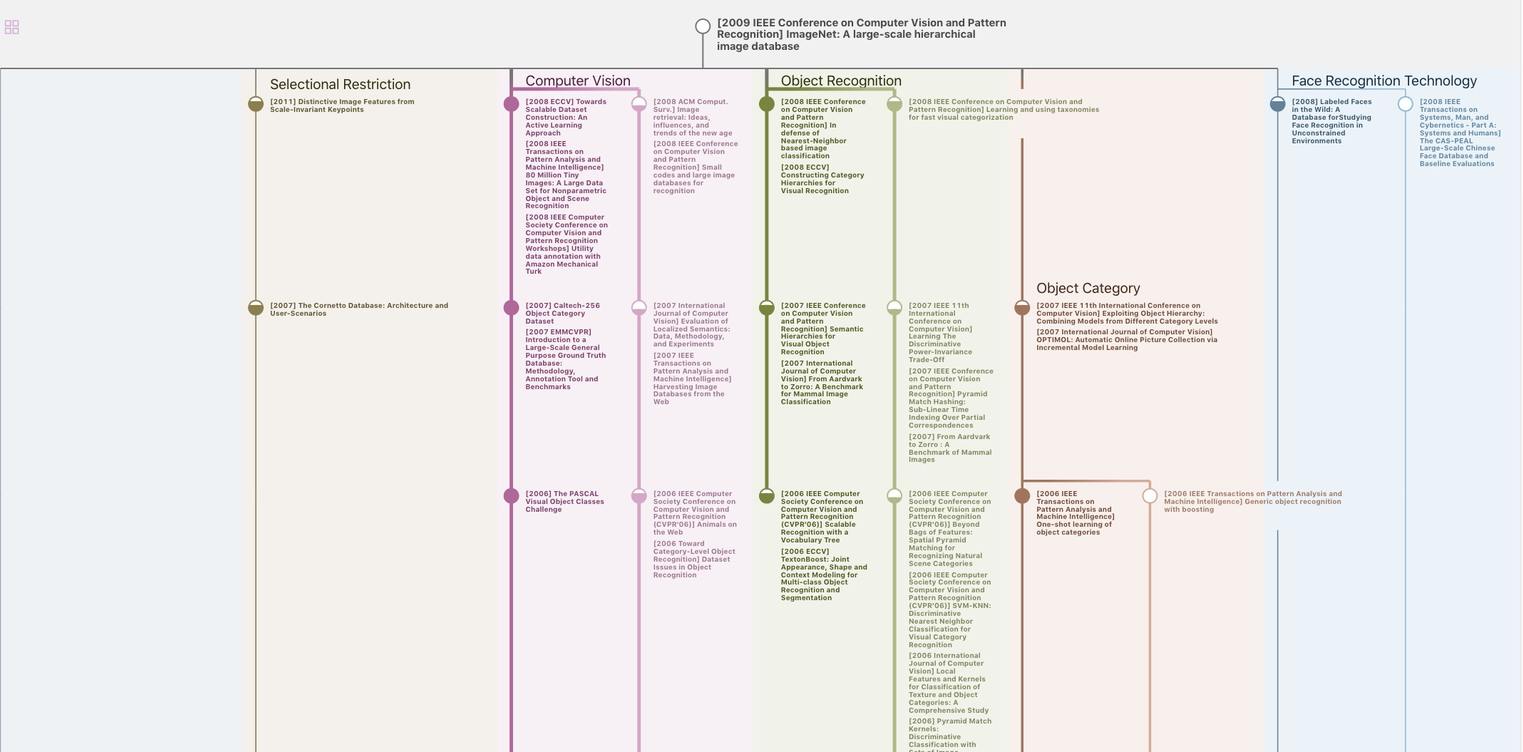
生成溯源树,研究论文发展脉络
Chat Paper
正在生成论文摘要