Notes On Image Processing With Partial Differential Equations
2015 IEEE International Conference on Image Processing (ICIP)(2015)
摘要
We discuss the underlying physics of transport coefficients in the PDE image processing approach and present a more principled method for their construction. Transport coefficients in PDEs play important roles, e.g., the diffusion coefficient c in the Perona-Malik equation partial derivative u/partial derivative t = del(inverted perpendicular) (c del u). Hence different models for c have been proposed in the literature. We present a physics-based method for constructing c and compare its performance with existing models. We also clarify an issue that arises in implementing the PDE approach. It is well-known that the product rule for derivatives in continuous spaces, namely, partial derivative(f g) = g partial derivative f + f partial derivative g, does not hold in discrete spaces. Thus computing such quantities as del(inverted perpendicular)(c del u) in spatially discretized images becomes ambiguous depending on which of the two alternatives we use, namely, whether to treat c del u as a single function or treat c and del u as two functions and use the product rule. We examine this ambiguity and show which alternative is better in the sense of yielding approximations that are closer to the continuous space results.
更多查看译文
关键词
Anisotropic diffusion,image processing,PDE,Perona-Malik equation,transport coefficient
AI 理解论文
溯源树
样例
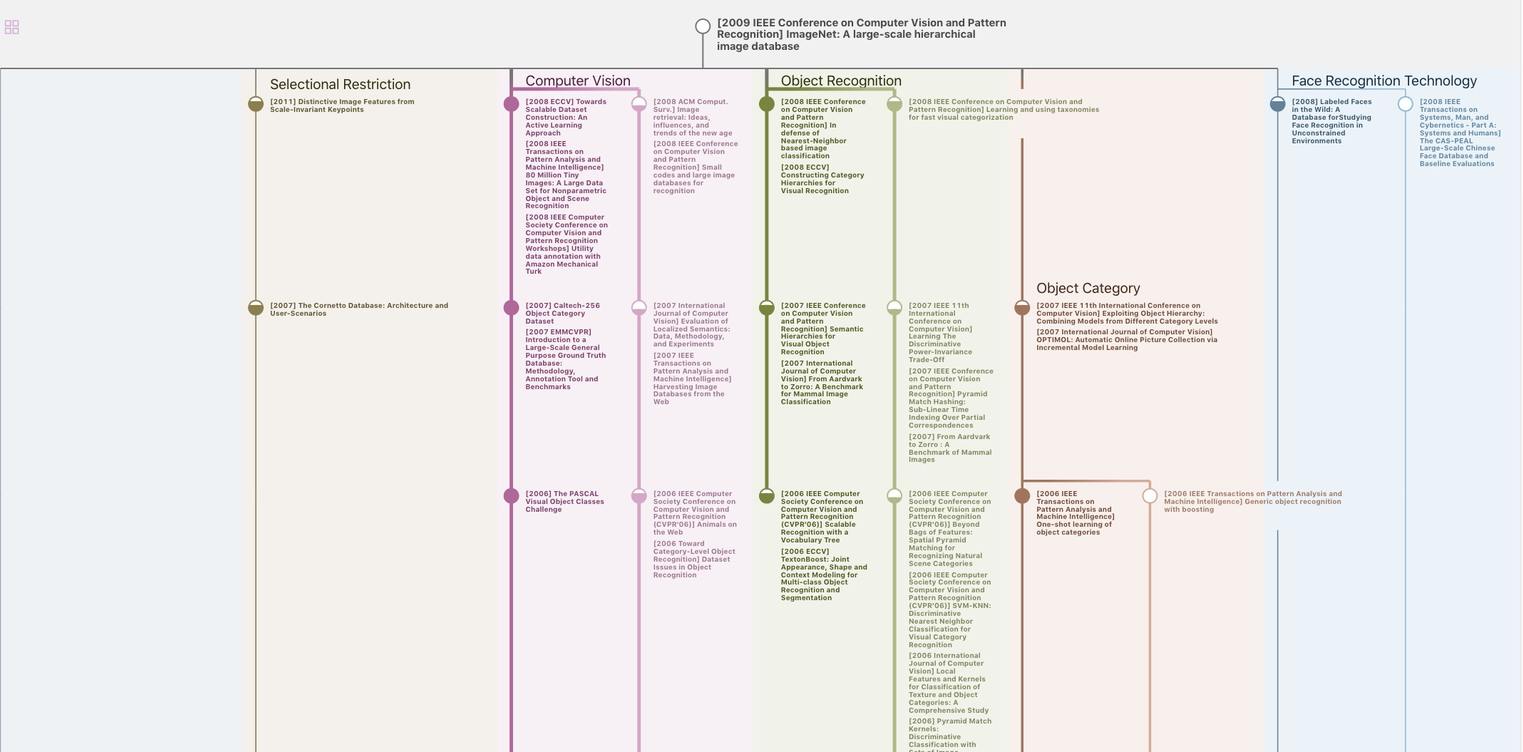
生成溯源树,研究论文发展脉络
Chat Paper
正在生成论文摘要