No-Reference Image Quality Assessment Using Shearlet Transform And Stacked Autoencoders
2015 IEEE International Symposium on Circuits and Systems (ISCAS)(2015)
摘要
In this work, we describe an efficient general-purpose no-reference (NR) image quality assessment (IQA) algorithm that is based on a new multiscale directional transform (shearlet transform) with a strong ability to localize distributed discontinuities. The algorithm relies on utilizing the sum of subband coefficient amplitudes (SSCA) as primary features to describe the behavior of natural images and distorted images. Then, stacked autoencoders are applied to exaggerate the discriminative parts of the primary features. Finally, by translating the NR-IQA problem into classification problem, the differences of evolved features are identified by softmax classifier. The resulting algorithm, which we name SESANIA (ShEarlet and Stacked Autoencoders based No-reference Image quality Assessment), is tested on several databases (LIVE, Multiply Distorted LIVE and TID2008) and shown to be suitable to many common distortions, consistent with subjective assessment and comparable to full-reference IQA methods and state-of-the-art general purpose NR-IQA algorithms.
更多查看译文
关键词
No-reference image quality assessment,Shearlet transform,Stacked autoencoders,Softmax classification
AI 理解论文
溯源树
样例
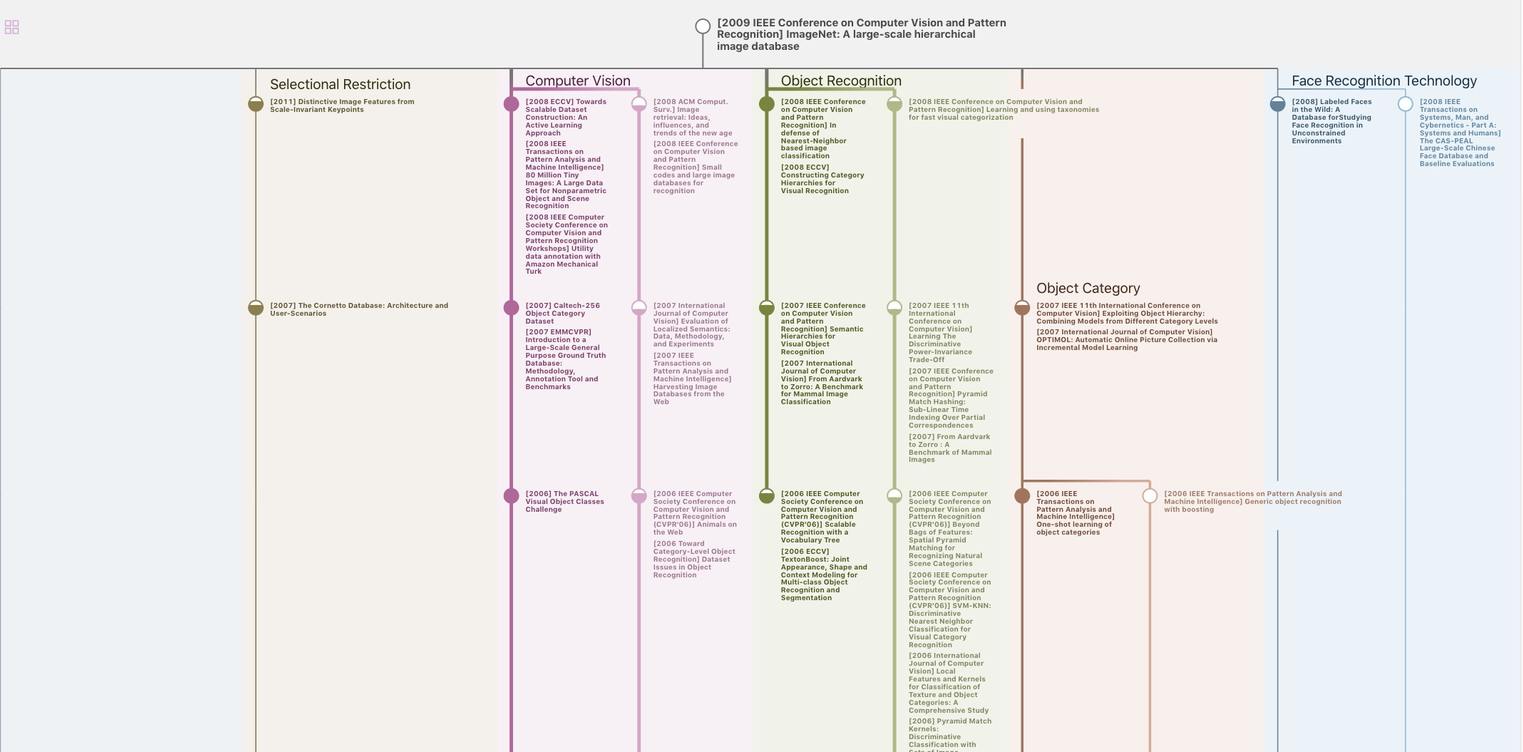
生成溯源树,研究论文发展脉络
Chat Paper
正在生成论文摘要