Battling against DDoS in SIP: Is Machine Learning-based detection an effective weapon?
2015 12th International Joint Conference on e-Business and Telecommunications (ICETE)(2015)
摘要
This paper focuses on network anomaly-detection and especially the effectiveness of Machine Learning (ML) techniques in detecting Denial of Service (DoS) in SIP-based VoIP ecosystems. It is true that until now several works in the literature have been devoted to this topic, but only a small fraction of them have done so in an elaborate way. Even more, none of them takes into account high and low-rate Distributed DoS (DDoS) when assessing the efficacy of such techniques in SIP intrusion detection. To provide a more complete estimation of this potential, we conduct extensive experimentations involving 5 different classifiers and a plethora of realistically simulated attack scenarios representing a variety of (D)DoS incidents. Moreover, for DDoS ones, we compare our results with those produced by two other anomaly-based detection methods, namely Entropy and Hellinger Distance. Our results show that ML-powered detection scores a promising false alarm rate in the general case, and seems to outperform similar methods when it comes to DDoS.
更多查看译文
关键词
Session Initiation Protocol,Machine Learning,DDoS,Anomaly-detection,Intrusion Detection Systems
AI 理解论文
溯源树
样例
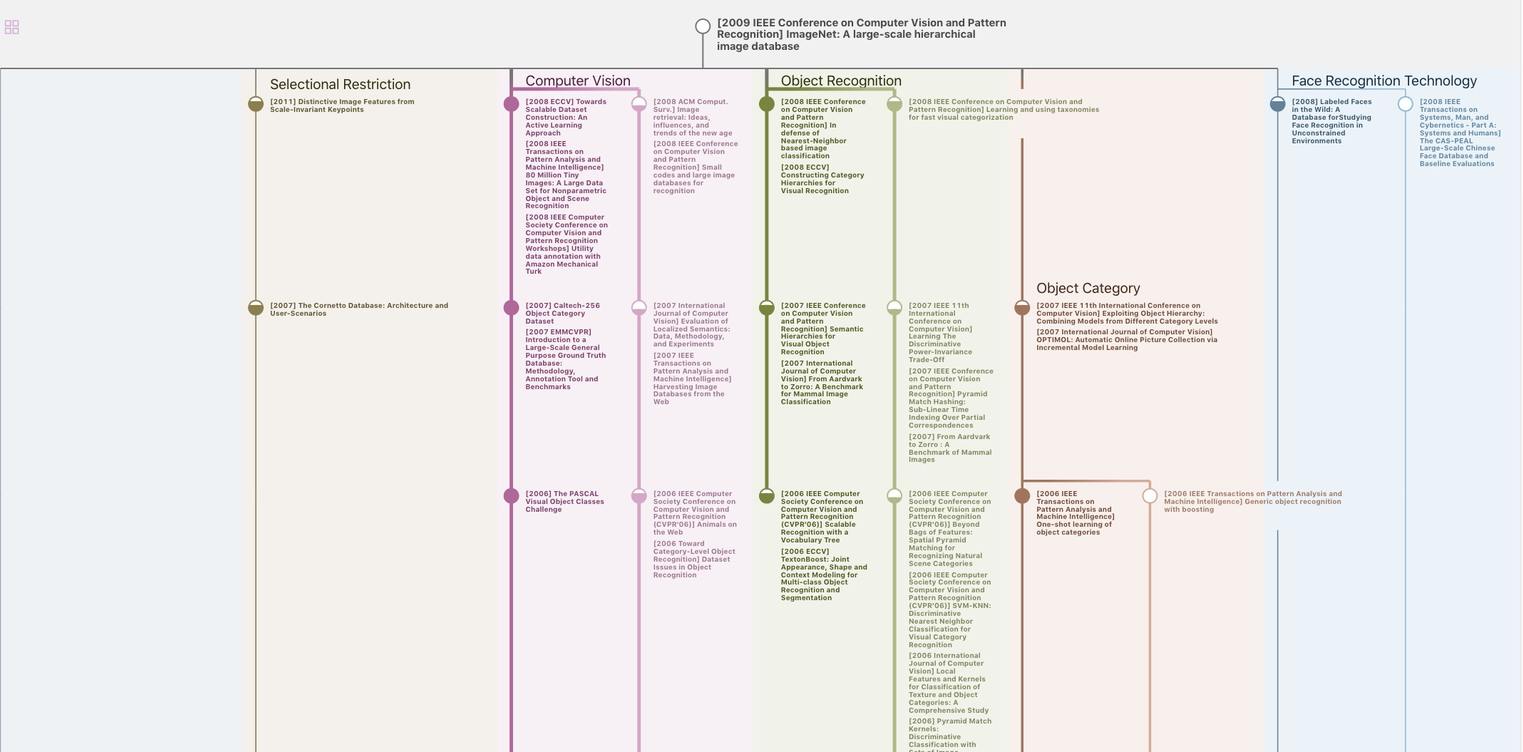
生成溯源树,研究论文发展脉络
Chat Paper
正在生成论文摘要