Finding a Path for Segmentation Through Sequential Learning.
IPMI(2015)
摘要
Sequential learning techniques, such as auto-context, that applies the output of an intermediate classifier as contextual features for its subsequent classifier has shown impressive performance for semantic segmentation. We show that these methods can be interpreted as an approximation technique derived from a Bayesian formulation. To improve the effectiveness of applying this approximation technique, we propose a new sequential learning approach for semantic segmentation that solves a segmentation problem by breaking it into a series of simplified segmentation problems. Sequentially solving each of the simplified problems along the path leads to a more effective way for solving the original segmentation problem. To achieve this goal, we also propose a learning-based method to generate simplified segmentation problems by explicitly controlling the complexities of the modeling classifiers. We report promising results on the 2013 SATA canine leg muscle segmentation dataset.
更多查看译文
关键词
Segmentation Problem, Simplified Segmentation, Joint Label Fusion (JLF), Trained AdaBoost Classifier, Gold Standard Segmentation
AI 理解论文
溯源树
样例
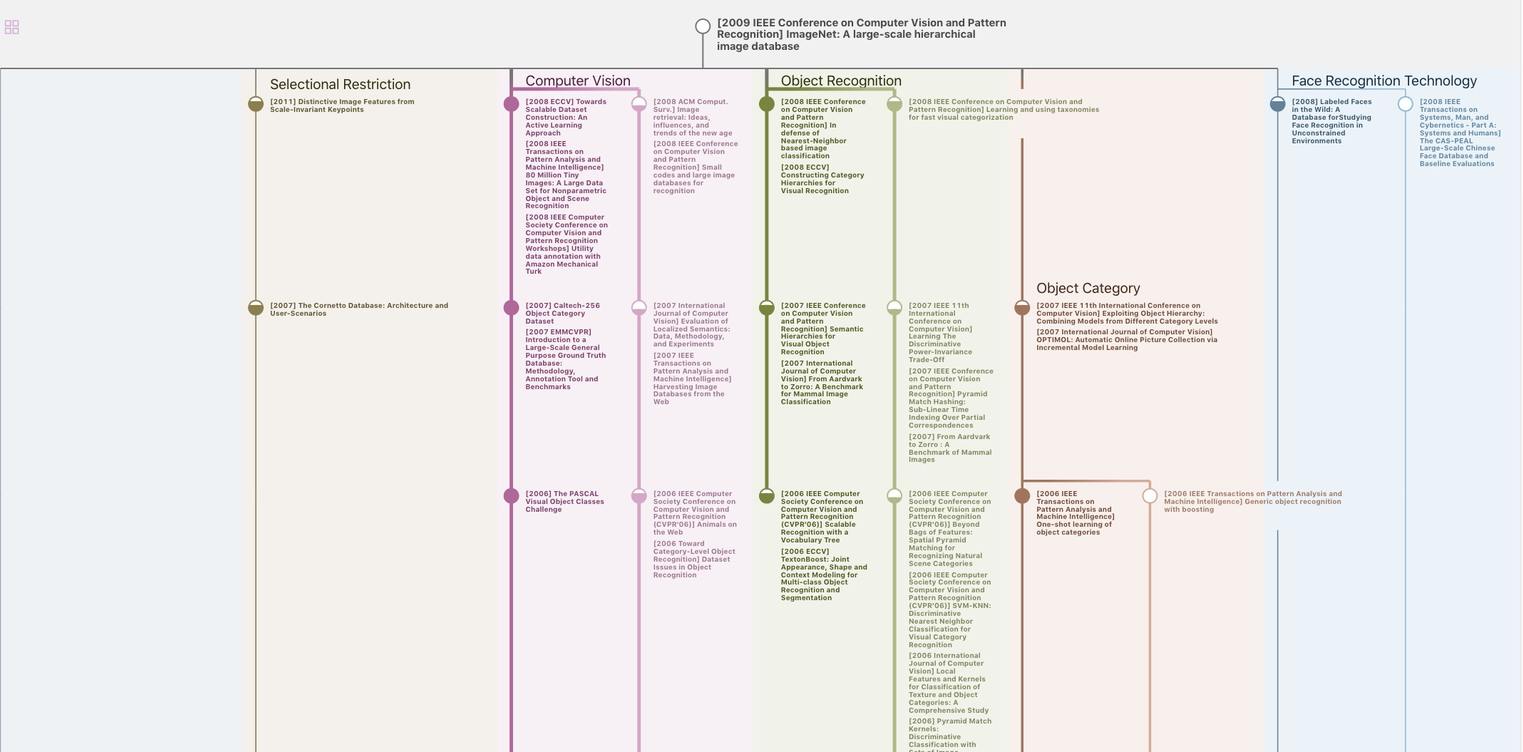
生成溯源树,研究论文发展脉络
Chat Paper
正在生成论文摘要