Combining Online Learning And Equilibrium Computation In Security Games
DECISION AND GAME THEORY FOR SECURITY, GAMESEC 2015(2015)
摘要
Game-theoretic analysis has emerged as an important method for making resource allocation decisions in both infrastructure protection and cyber security domains. However, static equilibrium models defined based on inputs from domain experts have weaknesses; they can be inaccurate, and they do not adapt over time as the situation (and adversary) evolves. In cases where there are frequent interactions with an attacker, using learning to adapt to an adversary revealed behavior may lead to better solutions in the long run. However, learning approaches need a lot of data, may perform poorly at the start, and may not be able to take advantage of expert analysis. We explore ways to combine equilibrium analysis with online learning methods with the goal of gaining the advantages of both approaches. We present several hybrid methods that combine these techniques in different ways, and empirically evaluated the performance of these methods in a game that models a border patrolling scenario.
更多查看译文
关键词
Game theory, Security games, Online learning, Stackelberg game, Stackelberg equilibrium, Nash equilibrium, Border patrol, Multi-armed bandit problem
AI 理解论文
溯源树
样例
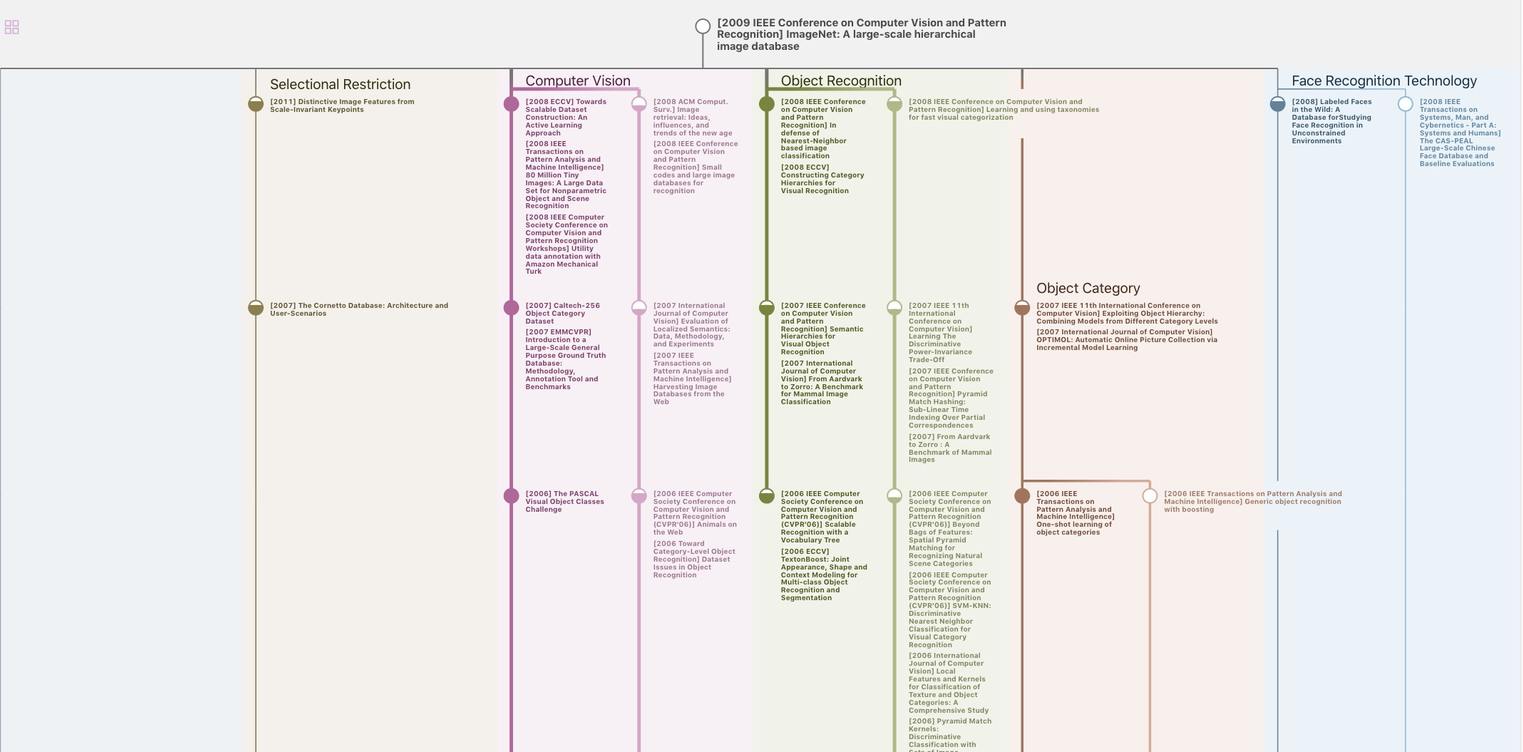
生成溯源树,研究论文发展脉络
Chat Paper
正在生成论文摘要