Learning Asymmetric Co-Relevance
ICTIR(2015)
摘要
Several applications in information retrieval rely on asymmetric co-relevance estimation; that is, estimating the relevance of a document to a query under the assumption that another document is relevant. We present a supervised model for learning an asymmetric co-relevance estimate. The model uses different types of similarities with the assumed relevant document and the query, as well as document-quality measures. Empirical evaluation demonstrates the merits of using the co-relevance estimate in various applications, including cluster-based and graph-based document retrieval. Specifically, the resultant performance transcends that of using a wide variety of alternative estimates, mostly symmetric inter-document similarity measures that dominate past work.
更多查看译文
AI 理解论文
溯源树
样例
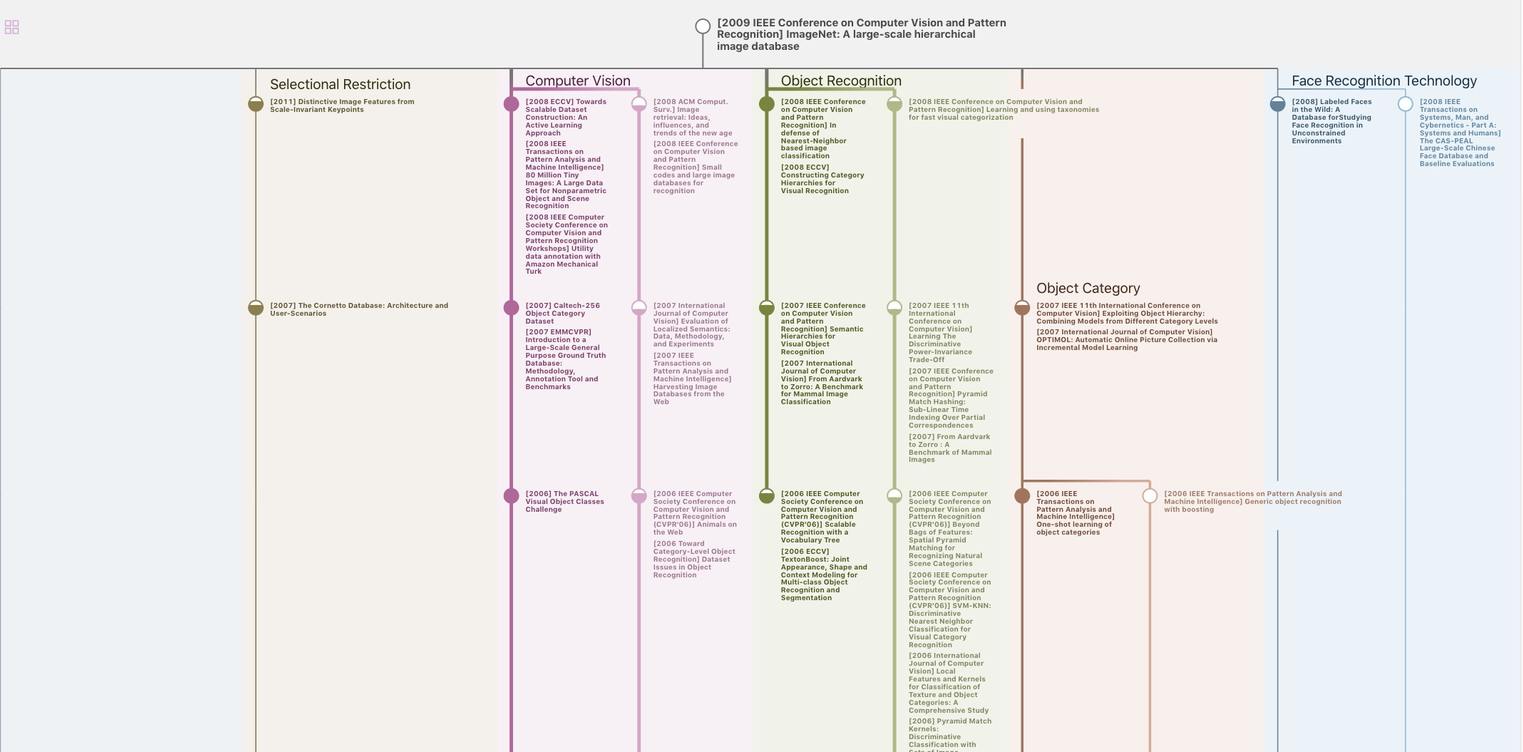
生成溯源树,研究论文发展脉络
Chat Paper
正在生成论文摘要