Surface Oriented Traverse For Robust Instance Detection In Rgb-D
2015 IEEE/RSJ International Conference on Intelligent Robots and Systems (IROS)(2015)
摘要
We address the problem of robust instance detection in RGB-D image in the presence of noisy data, cluttering, partial occlusion and large pose variation. We extract contour points from the depth image, construct a Surface Oriented Traverse (SOT) feature for each contour point and further classify it as either belonging or not belonging to the instance of interest. Starting from each contour point, its SOT feature is constructed by traversing and uniformly sampling along an oriented geodesic path on the object surface. After classification, all contour points vote for an instance-specific saliency map, from which the instance of interest is finally localized. Compared with the holistic template-based and learning-based methods, our method inherits advantages of the feature-based methods in dealing with cluttering, partial occlusion, and large pose variation. Furthermore, our method does not require accurate 3D models or high quality laser scan data as input and takes noisy data from commodity 3D sensors. Experimental results on the public RGB-D Object Dataset and our FindMe RGBD Dataset demonstrate the effectiveness and robustness of our proposed instance detection algorithm.
更多查看译文
关键词
robotic operation,image classification,geodesic path,contour point extraction,RGB-D image,instance detection,SOT,surface oriented traverse
AI 理解论文
溯源树
样例
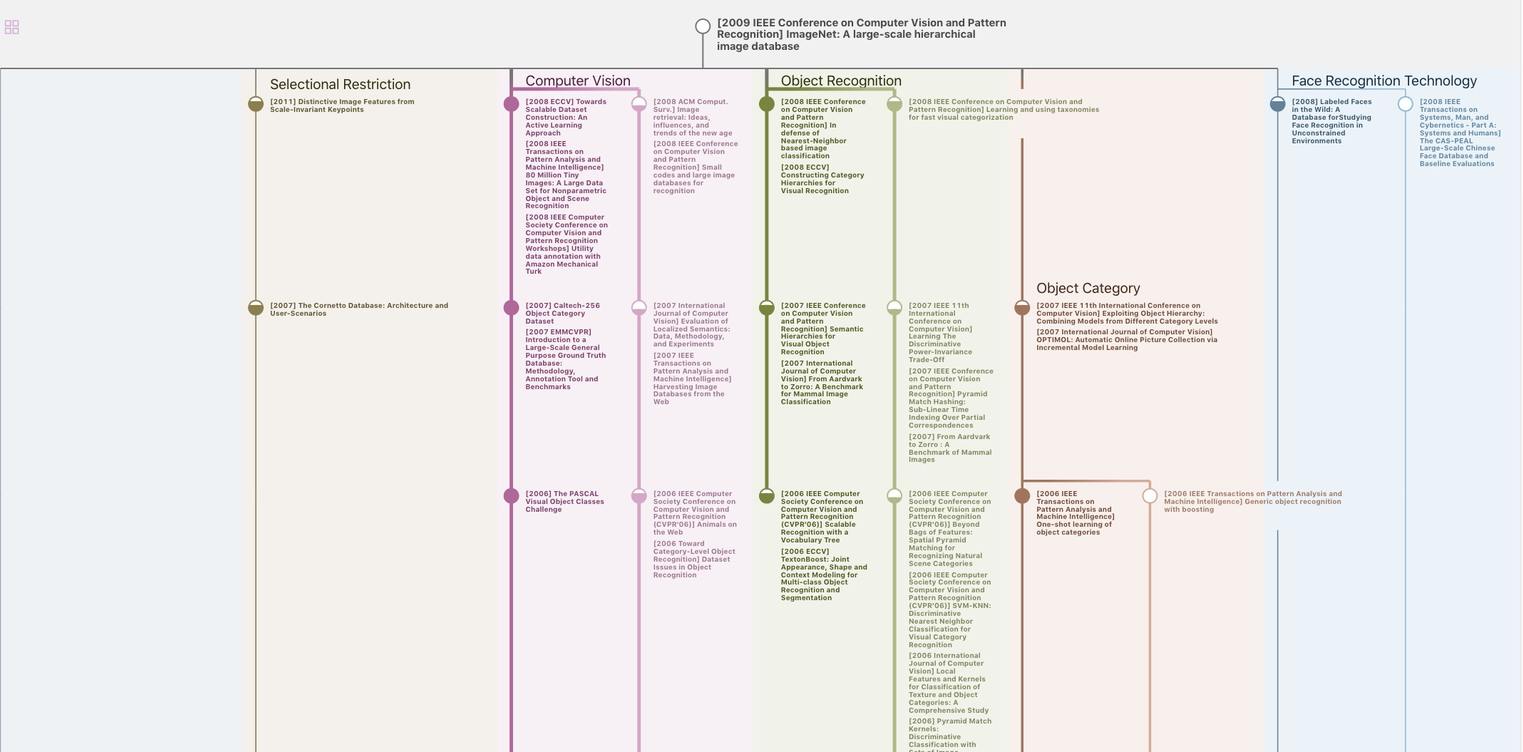
生成溯源树,研究论文发展脉络
Chat Paper
正在生成论文摘要