Detection Of Localization Failure Using Logistic Regression
2015 IEEE/RSJ International Conference on Intelligent Robots and Systems (IROS)(2015)
摘要
Monte Carlo localization (MCL) is a sample-based approach for representing probability density for the pose of a robot. MCL is widely used for mobile robots because of its robustness with respect to sensor noise. On the other hand, MCL can fail to estimate a pose of a robot if objects block measuring range of a laser sensor of the robot. Even though MCL fails to estimate the pose of the robot, MCL does not stop to estimate the pose because MCL does not have a self-diagnostic function. Therefore, detection of localization failure is essential for a mobile robot application. In the present paper, we propose a novel approach that detects the localization failure of MCL through logistic regression. The proposed approach has two advantages. First, it can detect whether position errors is larger than 0.15 m with a high accuracy. Second, the proposed method can detect localization failure by means of a statistically modeled equation. Moreover, as an example of an application using the probability of localization failure, we have proposed a hybrid localization scheme with MCL and laser odometry. The practical effectiveness of the proposed scheme is verified through experiments.
更多查看译文
关键词
statistically modeled equation,hybrid localization scheme,laser odometry,position errors,logistic regression,MCL localization failure detection,laser sensor,pose estimation,sensor noise robustness,mobile robots,probability density representation,sample-based approach,Monte Carlo localization
AI 理解论文
溯源树
样例
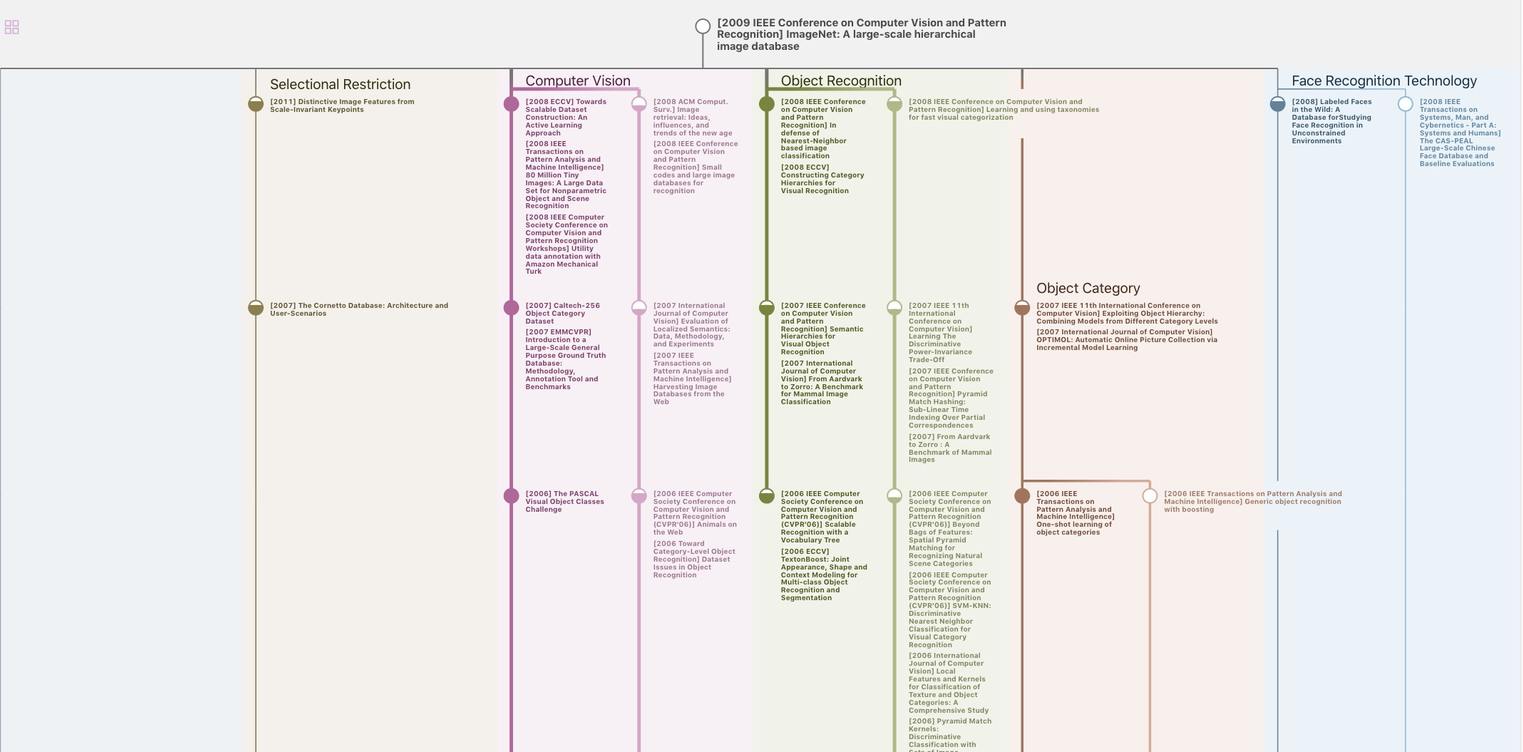
生成溯源树,研究论文发展脉络
Chat Paper
正在生成论文摘要