Fast Sparse Recovery Via Non-Convex Optimization
2015 IEEE Global Conference on Signal and Information Processing (GlobalSIP)(2015)
摘要
Recovering sparse signals from noisy underdetermined linear measurements has been a concerning problem in the signal processing community. Lasso has been put forward to handle this problem well, yet recent research reveals that replacing l(1) norm with some non-convex functions leads to better recovery performance. In this paper, based on majorization-minimization and proximal operator, we propose a fast algorithm for the non-convex function regularized least squares problem. Theoretical analysis shows that any limit point of the iterative sequence is a stationary point of the problem, and if the non-convexity is below a threshold, the iterative sequence converges to a neighborhood of the sparse signal with superlinear convergence rate. Simulation results verify such theoretical rate of convergence, and demonstrate that the algorithm outperforms its convex counterpart in various aspects including more nonzero entries allowed, less running time required, and better denoising performance exhibited.
更多查看译文
关键词
Sparse recovery,non-convex optimization,iterative regularization,convergence,rate of convergence
AI 理解论文
溯源树
样例
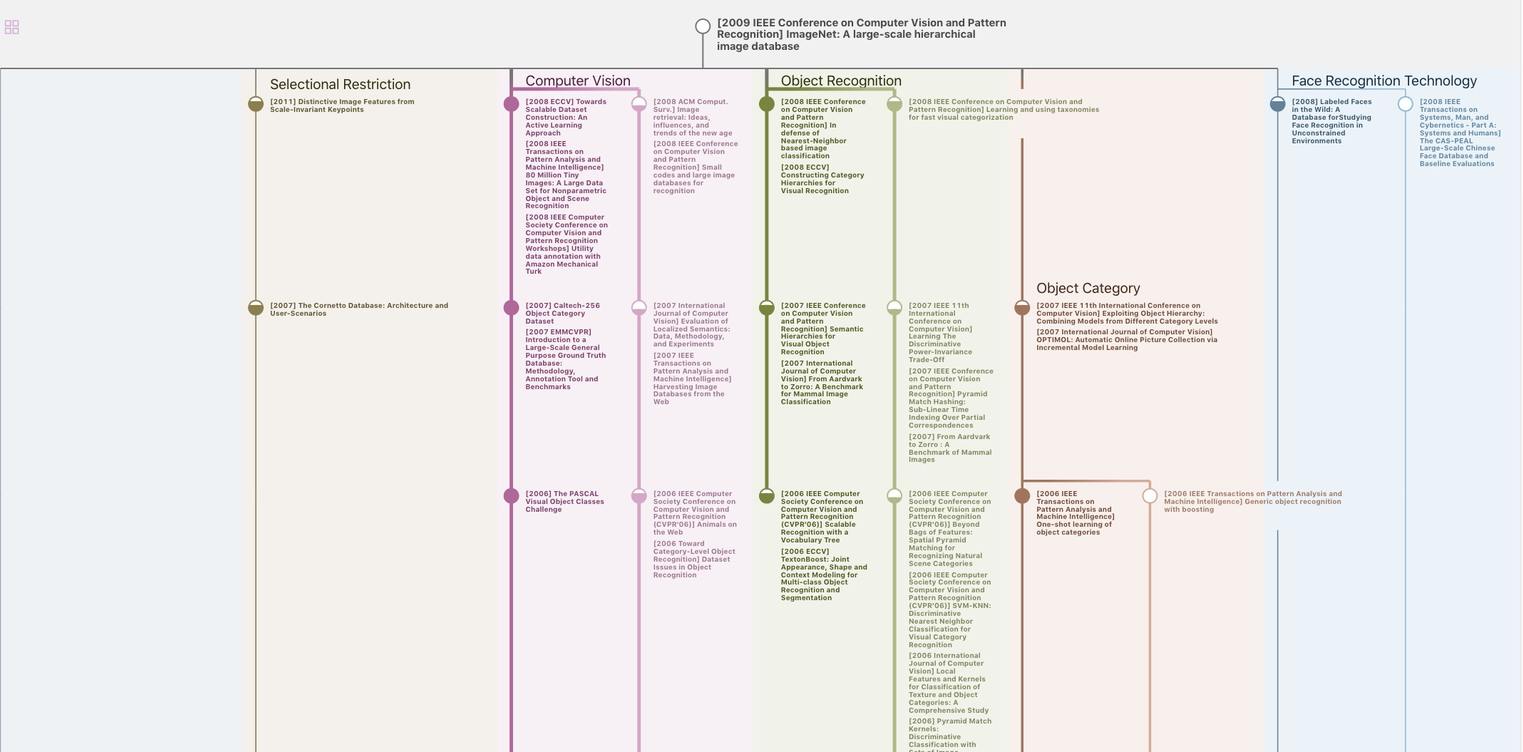
生成溯源树,研究论文发展脉络
Chat Paper
正在生成论文摘要