Stabilizing Sparse Cox Model Using Statistic and Semantic Structures in Electronic Medical Records.
ADVANCES IN KNOWLEDGE DISCOVERY AND DATA MINING, PART II(2015)
摘要
Stability in clinical prediction models is crucial for transferability between studies, yet has received little attention. The problem is paramount in high dimensional data, which invites sparse models with feature selection capability. We introduce an effective method to stabilize sparse Cox model of time-to-events using statistical and semantic structures inherent in Electronic Medical Records (EMR). Model estimation is stabilized using three feature graphs built from (i) Jaccard similarity among features (ii) aggregation of Jaccard similarity graph and a recently introduced semantic EMR graph (iii) Jaccard similarity among features transferred from a related cohort. Our experiments are conducted on two real world hospital datasets: a heart failure cohort and a diabetes cohort. On two stability measures - the Consistency index and signal-to-noise ratio (SNR) - the use of our proposed methods significantly increased feature stability when compared with the baselines.
更多查看译文
关键词
Electronic Medical Record, Consistency Index, Transfer Learning, Jaccard Index, Semantic Structure
AI 理解论文
溯源树
样例
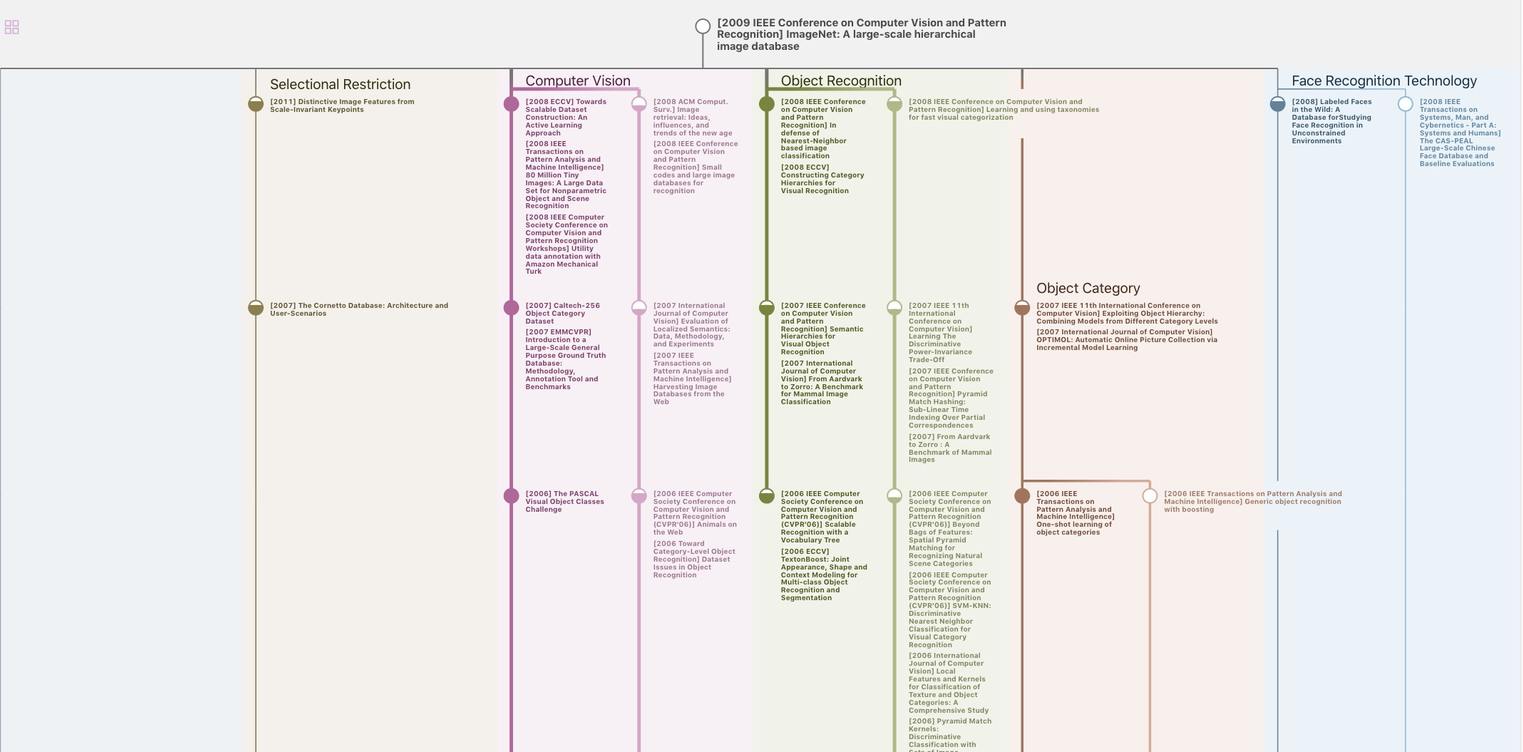
生成溯源树,研究论文发展脉络
Chat Paper
正在生成论文摘要