Explaining And Aggregating Anomalies To Detect Insider Threats
2016 49th Hawaii International Conference on System Sciences (HICSS)(2016)
摘要
Anomalies in computer usage data may be indicative of insider threats. Distinguishing actual malicious activities from unusual but justifiable activities requires not only a sophisticated anomaly detection system but also the expertise of human analysts with access to additional data sources. Because any anomaly detection system for extremely rare events will generate many false positives, human analysts must decide which anomalies are worth their time and effort for follow-up investigations. Providing a ranked or scored list of users the typical output of an anomaly detection system is necessary but far from sufficient for this purpose. Anomalies indicative of insider threats can be distinguished from those that arise from legitimate activity by explaining why they are anomalous, and high-risk users may be identified by their repeated appearance near the top of the ranked and scored lists. This paper describes results of experiments that show the utility of these techniques of explaining and aggregating anomalies to detect insider threats with greater accuracy than is achieved solely with anomaly detection methods.
更多查看译文
关键词
Anomaly Detection,Outlier Detection,Explanation,Temporal Aggregation
AI 理解论文
溯源树
样例
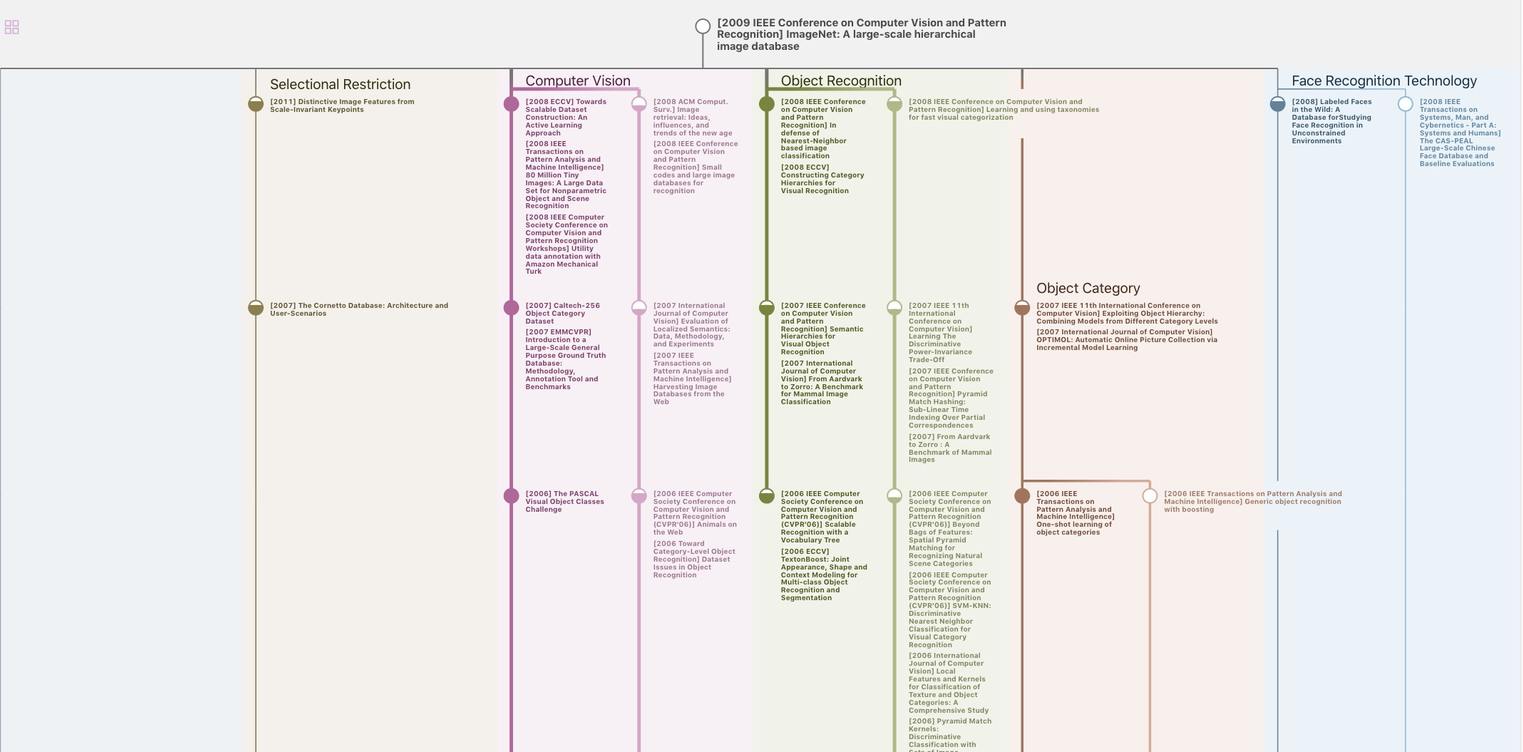
生成溯源树,研究论文发展脉络
Chat Paper
正在生成论文摘要