Structure Learning Constrained By Node-Specific Degree Distribution
UAI'15: Proceedings of the Thirty-First Conference on Uncertainty in Artificial Intelligence(2015)
摘要
We consider the problem of learning the structure of a Markov Random Field (MRF) when a node-specific degree distribution is provided. The problem setting is inspired by protein contact map (i.e., graph) prediction in which the contact number (i.e., degree) of an individual residue (i.e., node) can be predicted without knowing the contact graph. We formulate this problem using maximum pseudo-likelihood plus a node-specific l(1) regularization derived from the predicted degree distribution. Intuitively, when a node have.. predicted edges, we dynamically reduce the regularization coefficients of the.. most possible edges to promote their occurrence. We then optimize the objective function using ADMM and an Iterative Maximum Cost Bipartite Matching algorithm. Our experimental results show that using degree distribution as a constraint may lead to significant performance gain when the predicted degree has good accuracy.
更多查看译文
AI 理解论文
溯源树
样例
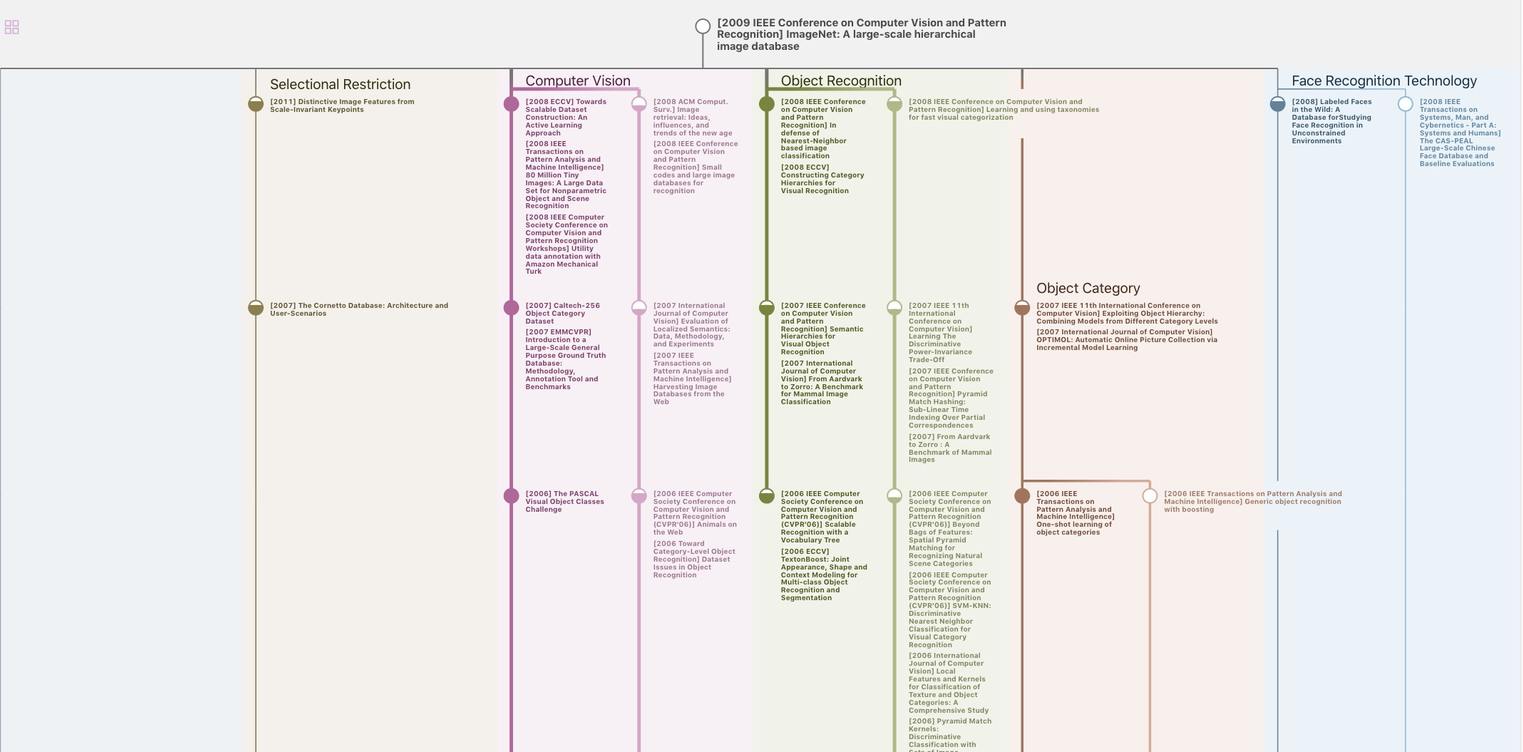
生成溯源树,研究论文发展脉络
Chat Paper
正在生成论文摘要