Gpu-Based Fast Parameter Optimization For Phenomenological Spiking Neural Models
2015 International Joint Conference on Neural Networks (IJCNN)(2015)
摘要
A significant obstacle in using phenomenological models of spiking neurons for large-scale simulations is the approximation of the optimal parameters for a type of neuron, given the available experimental data. Here we show a method for optimizing the parameters of such models, based on a combination of different frequency-current and voltage-current relations of a neuron as well as known physiological properties. We also present a python toolbox which uses NeMo spiking neural network simulator and provides a fast GPU-based implementation of our method. As a benchmark, our toolbox was used to fit Izhikevich equations to neurological data obtained from a eat's thalamic relay cell. Our resulting model was able to predict the firing patterns of known membrane potential traces of this neuron, although they were not explicitly defined during training. A further comparison between this neuron model and a previous approach, when both models are used in the simulation of a generic thalamic nucleus, revealed that the distribution of neuronal avalanches is significantly different and conforms better to power law-like distributions, thus increasing the likelihood of a critical regime and the biological plausibility of the simulation.
更多查看译文
关键词
GPU,fast parameter optimization,phenomenological spiking neural models,optimal parameter approximation,frequency-current relation,voltage-current relation,physiological properties,Python toolbox,NeMo spiking neural network simulator,Izhikevich equations,cat thalamic relay cell,membrane potential traces,neuronal avalanche distribution
AI 理解论文
溯源树
样例
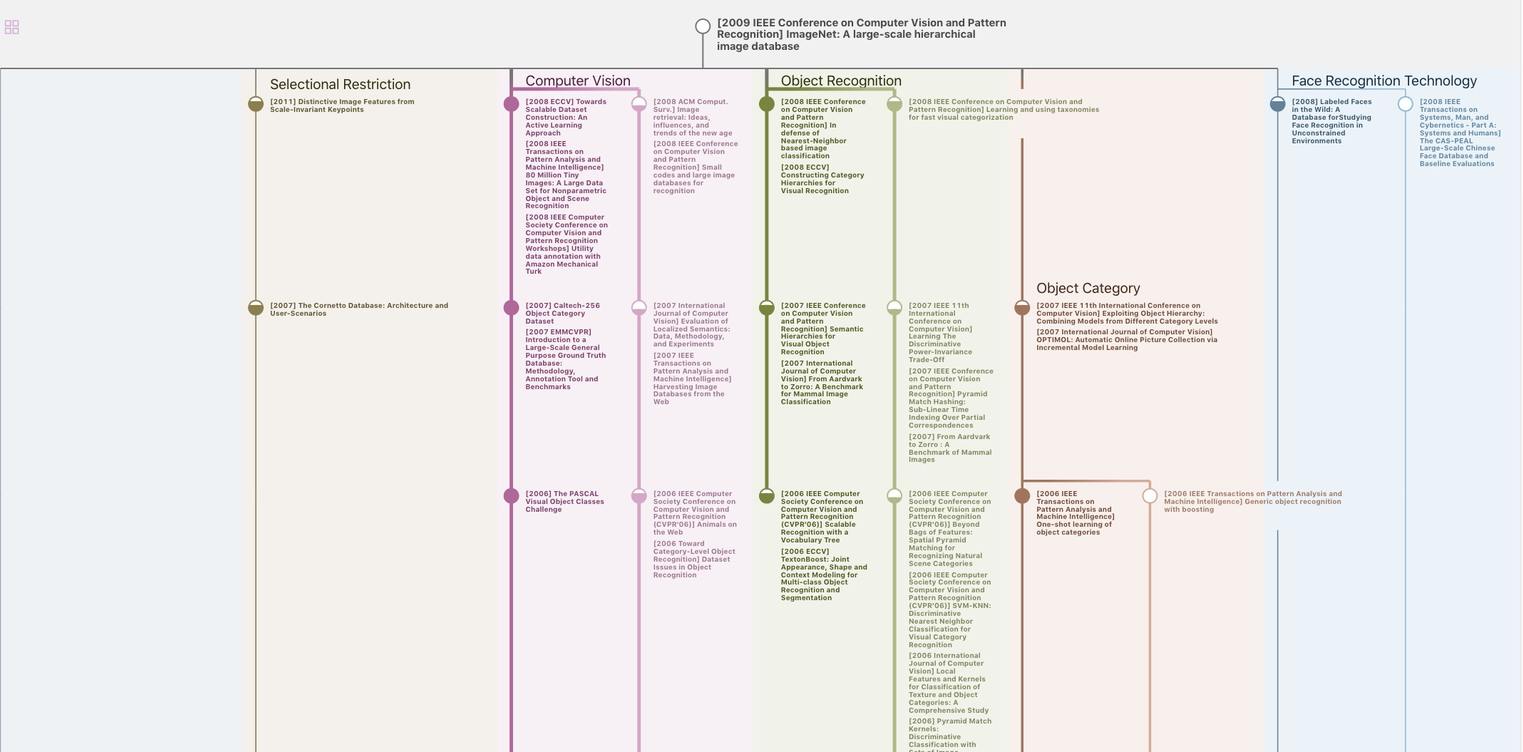
生成溯源树,研究论文发展脉络
Chat Paper
正在生成论文摘要