Stochastic Discriminant Analysis
2015 International Joint Conference on Neural Networks (IJCNN)(2015)
摘要
In this paper, we consider a linear supervised dimension reduction method for classification settings: Stochastic Discriminant Analysis. This method matches point similarities in the projection space with those in a response space. These similarities are represented by t-distributed joint pairwise probabilities. The matching is done by minimizing the Kullback-Leibler divergence between the two probability distributions. The performance of the algorithm is compared against state-of-the-art methods in supervised dimension reduction. We found that the performance of SDA is comparable to (and sometimes better than) state-of-the-art methods in supervised linear dimension reduction. In the presence of multiple classes, low-dimensional SDA projections led to higher classification accuracies.
更多查看译文
关键词
stochastic discriminant analysis,linear supervised dimension reduction method,classification settings,response space,t-distributed joint pairwise probabilities,Kullback-Leibler divergence,probability distributions,supervised linear dimension reduction,low-dimensional SDA projections
AI 理解论文
溯源树
样例
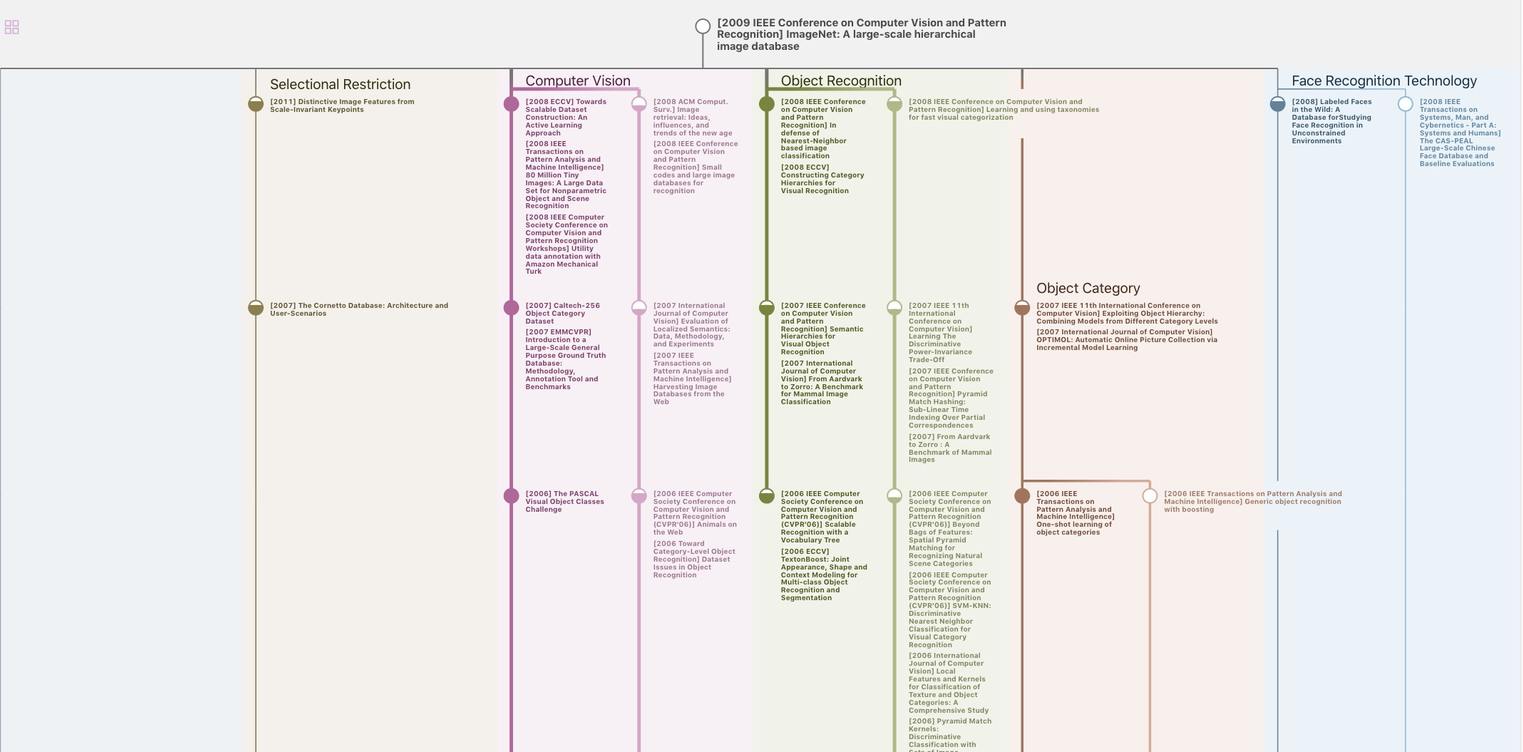
生成溯源树,研究论文发展脉络
Chat Paper
正在生成论文摘要