Low Area Overhead In-Situ Training Approach For Memristor-Based Classifier
PROCEEDINGS OF THE 2015 IEEE/ACM INTERNATIONAL SYMPOSIUM ON NANOSCALE ARCHITECTURES (NANOARCH 15)(2015)
摘要
We propose combination of "dropout" and "Manhattan Rule" training algorithms for memristive crossbar neural networks to reduce circuit area overhead of in -situ training. Using accurate phenomenological model of memristive devices, we show that such combination allows achieving 0.7% misclassification rate on the MNIST benchmark, which is comparable to the best reported results. At the same time, the considered training approach allows reducing the size of memory circuits, the largest area overhead component, which is required to store intermediate weight adjustments during training, by as much as 40% at 16% longer training time as compared to the baseline crossbar circuit compatible "Manhattan Rule" training. The further reduction of the memory circuit area overhead is possible but at the expense of inferior classification performance.
更多查看译文
关键词
Memristor,Crossbar,Multilayer Perceptron,Manhattan Rule,Dropout training,Pattern Classification
AI 理解论文
溯源树
样例
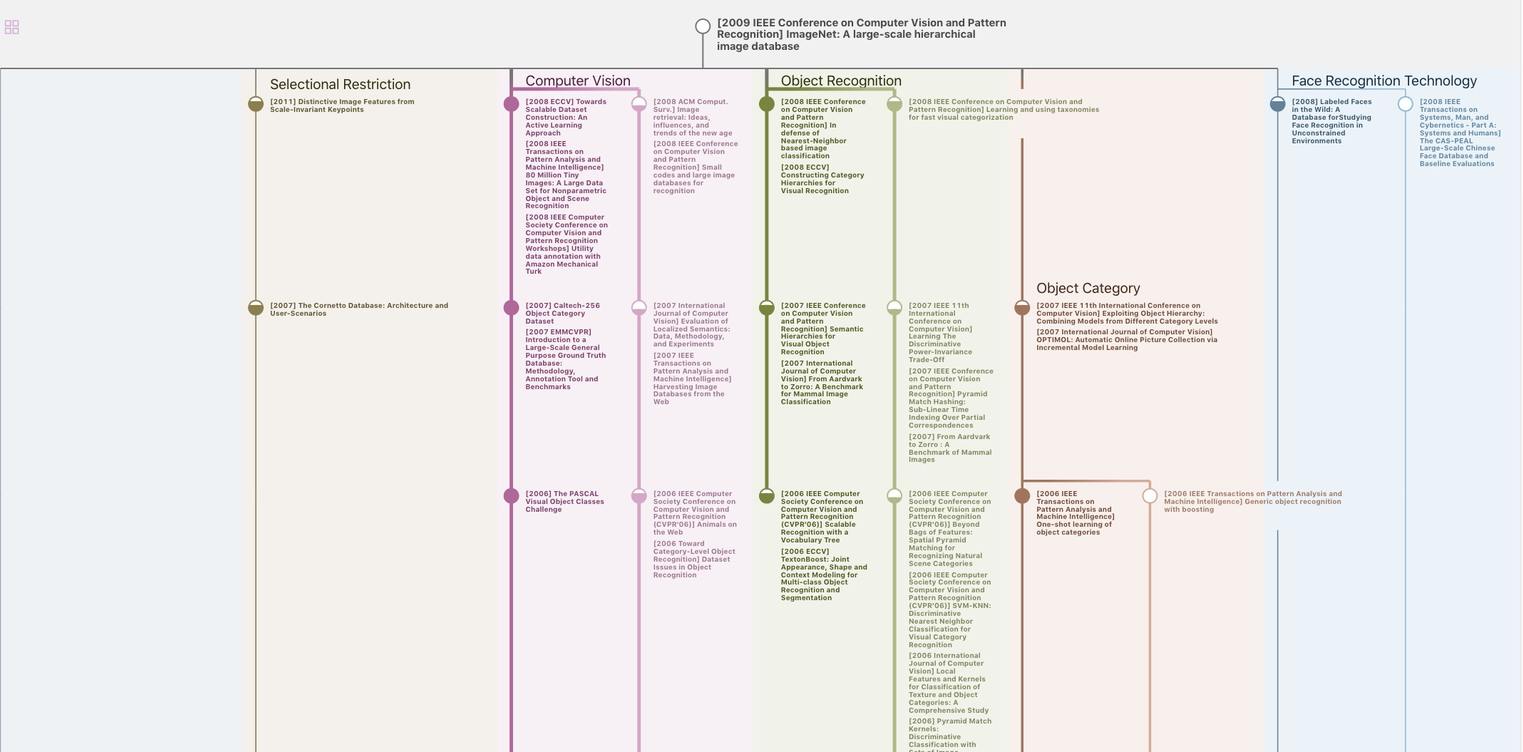
生成溯源树,研究论文发展脉络
Chat Paper
正在生成论文摘要