Progressive Blind Deconvolution.
CAIP(2015)
摘要
We present a novel progressive framework for blind image restoration. Common blind restoration schemes first estimate the blur kernel, then employ non-blind deblurring. However, despite recent progress, the accuracy of PSF estimation is limited. Furthermore, the outcome of non-blind deblurring is highly sensitive to errors in the assumed PSF. Therefore, high quality blind deblurring has remained a major challenge. In this work, we combine state of the art regularizers for the image and the PSF, namely the Mumford & Shah piecewise-smooth image model and the sparse PSF prior. Previous works that used Mumford & Shah image regularization were either limited to non-blind deblurring or semi-blind deblurring assuming a parametric kernel known up to an unknown parameter. We suggest an iterative progressive restoration scheme, in which the imperfectly deblurred output of the current iteration is fed back as input to the next iteration. The kernel representing the residual blur is then estimated, and used to drive the non-blind restoration component, leading to finer deblurring. Experimental results demonstrate rapid convergence, and excellent performance on a wide variety of blurred images.
更多查看译文
关键词
Progressive blind restoration, Residual blur removal, Image deblurring, Piecewise-smooth image model, Mumford & Shah regularization, Sparse PSF prior
AI 理解论文
溯源树
样例
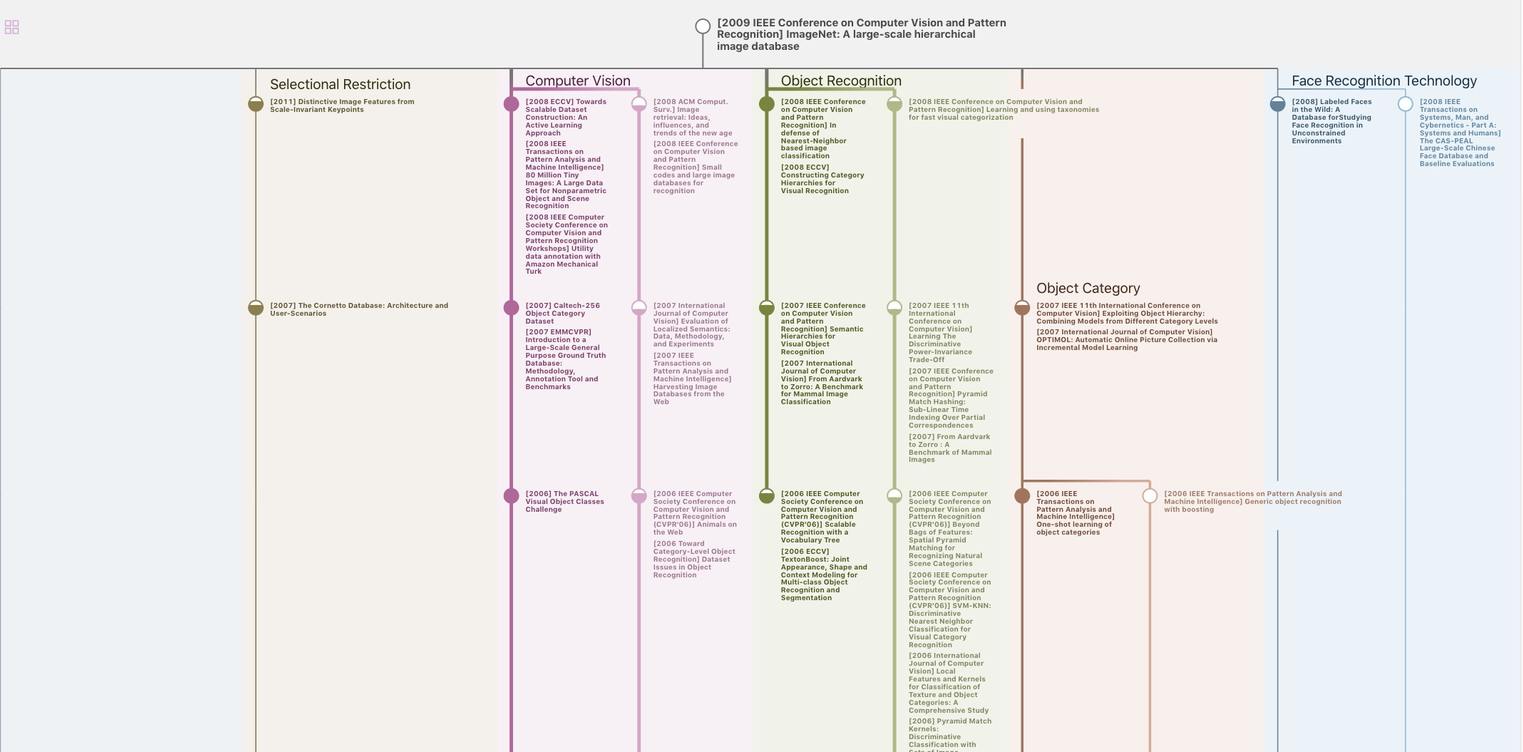
生成溯源树,研究论文发展脉络
Chat Paper
正在生成论文摘要