Fire Detection from Social Media Images by Means of Instance-Based Learning.
Lecture Notes in Business Information Processing(2015)
摘要
Social media can provide valuable information to support decision making in crisis management, such as in accidents, explosions, and fires. However, much of the data from social media are images, which are uploaded at a rate that makes it impossible for human beings to analyze them. To cope with that problem, we design and implement a database-driven architecture for fast and accurate fire detection named FFireDt. The design of FFireDt uses the instance-based learning through indexed similarity queries expressed as an extension of the relational Structured Query Language. Our contributions are: (i) the design of the Fast-Fire Detection (FFireDt), which achieves efficiency and efficacy rates that rival to the state-of-the-art techniques; (ii) the sound evaluation of 36 image descriptors, for the task of image classification in social media; (iii) the evaluation of content-based indexing with respect to the construction of instance-based classification systems; and (iv) the curation of a ground-truth annotated dataset of fire images from social media. Using real data from Flickr, the experiments showed that system FFireDt was able to achieve a precision for fire detection comparable to that of human annotators. Our results are promising for the engineering of systems to monitor images uploaded to social media services.
更多查看译文
关键词
Fire detection,Image descriptors,Social media,Extended-SQL
AI 理解论文
溯源树
样例
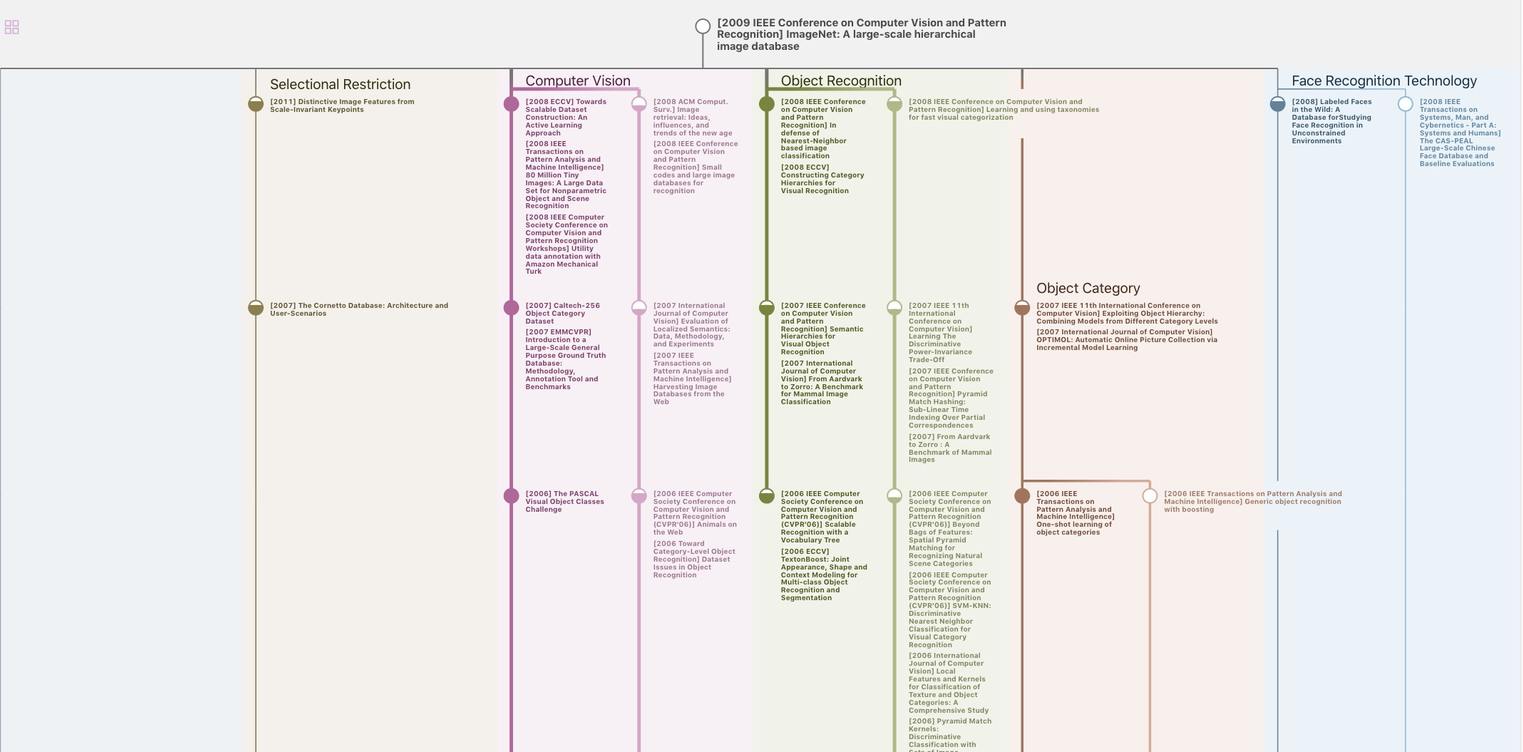
生成溯源树,研究论文发展脉络
Chat Paper
正在生成论文摘要