Concept-Based Relevance Models for Medical and Semantic Information Retrieval.
CIKM'15: 24th ACM International Conference on Information and Knowledge Management Melbourne Australia October, 2015(2015)
摘要
Relevance models provide an important approach for estimating probabilities of words in the relevant class. However, the associated bag-of-words assumption breaks dependencies between words, especially between those within a phrase. If such dependencies could be preserved, it would permit matching the query terms with document terms having the same dependencies. Additionally, during the estimation of relevance, relevance models are unable to distinguish relevant and non-relevant information in a feedback document, and hence take the entire document into account, which potentially hurts the accuracy of estimation. In this paper, we define the notion of "concept", and design a concept-based information retrieval framework. Using this framework, we transform documents and queries from term space into concept space, and propose a concept-based relevance model for improved estimation of relevance. Our approach has three advantages. First, this approach only assumes independence between concepts, so is able to keep the strong dependencies between the words of a concept. Second, it unifies synonyms or different surface forms of a concept, leading to reduced dimensionality of the space, increased sample size of a concept, and consequently more accurate and reliable estimates of the relevance. Third, when knowledge bases are available, our approach enables the semantic analysis of query concepts, and thus identifies concepts related to the query, from which a more accurate distribution of relevance can be estimated. This work is aligned with semantic search methods.
We apply our concept-based relevance model to information retrieval in the medical domain, where concepts are abundant and their variations are numerous. We compare with relevance models, BM25 with pseudo relevance feedback, and the state of the art conceptual language models, on several data collections. The proposed model demonstrates consistent and statistically significant improvements across collections, outperforming top benchmark conceptual language models by at least 9% and up to 20% on a number of metrics.
更多查看译文
AI 理解论文
溯源树
样例
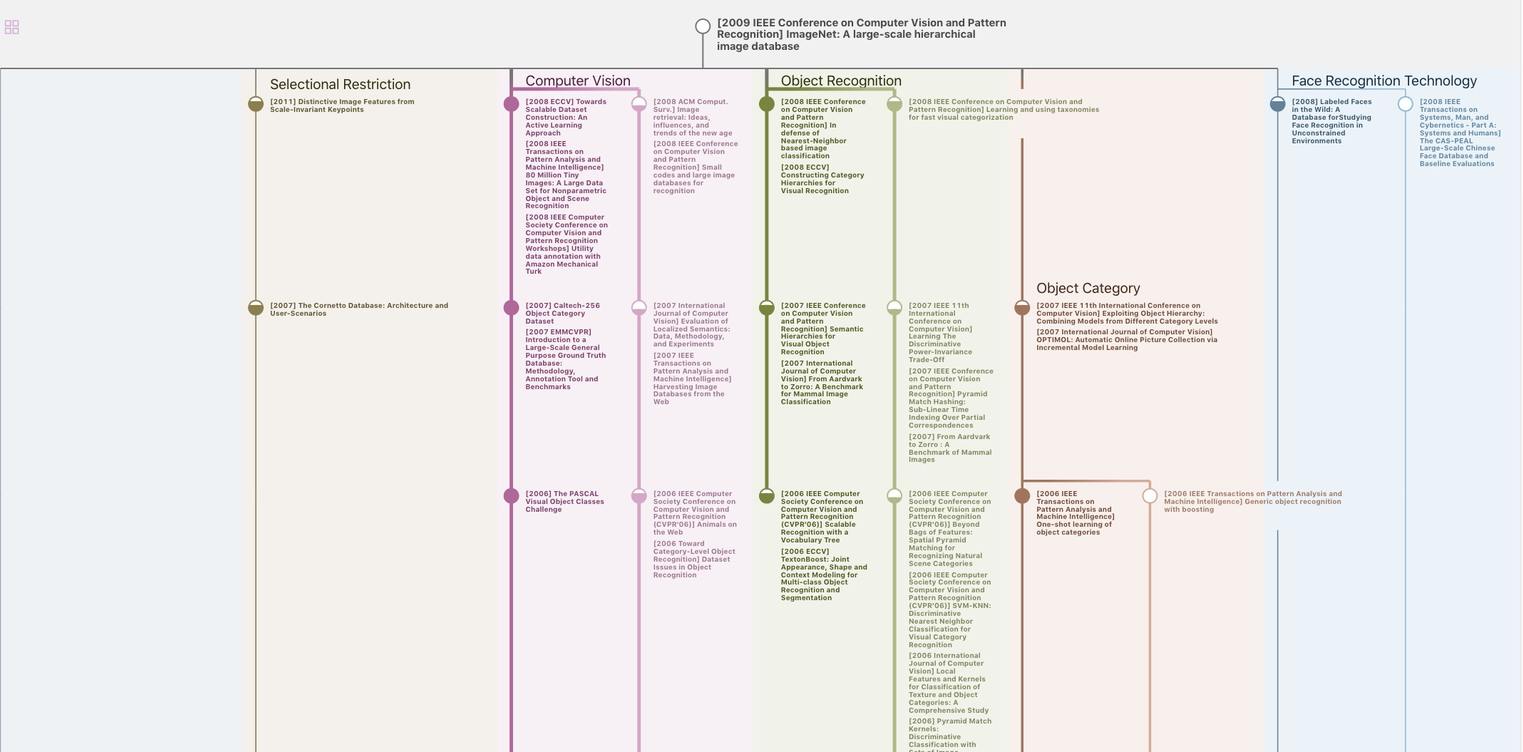
生成溯源树,研究论文发展脉络
Chat Paper
正在生成论文摘要