Context-Adaptive Matrix Factorization for Multi-Context Recommendation
ACM International Conference on Information and Knowledge Management(2015)
摘要
Data sparsity is a long-standing challenge for recommender systems based on collaborative filtering. A promising solution for this problem is multi-context recommendation, i.e., leveraging users' explicit or implicit feedback from multiple contexts. In multi-context recommendation, various types of interactions between entities (users and items) are combined to alleviate data sparsity of a single context in a collective manner. Two issues are crucial for multi-context recommendation: (1) How to differentiate context-specific factors from entity-intrinsic factors shared across contexts? (2) How to capture the salient phenomenon that some entities are insensitive to contexts while others are remarkably context-dependent? Previous methods either do not consider context-specific factors, or assume that a context imposes equal influence on different entities, limiting their capability of combating data sparsity problem by taking full advantage of multiple contexts.
In this paper, we propose a context-adaptive matrix factorization method for multi-context recommendation by simultaneously modeling context-specific factors and entity-intrinsic factors in a unified model. We learn an entity-intrinsic latent factor for every entity, and a context-specific latent factor for every entity in each context. Meanwhile, using a context-entity mixture parameter matrix we explicitly model the extent to which each context imposes influence on each entity. Experiments on two real scenarios demonstrate that our method consistently outperforms previous multi-context recommendation methods on all different sparsity levels.Such a consistent performance promotion forms the unique superiority of our method, enabling it to be a reliable model for multi-context recommendation.
更多查看译文
AI 理解论文
溯源树
样例
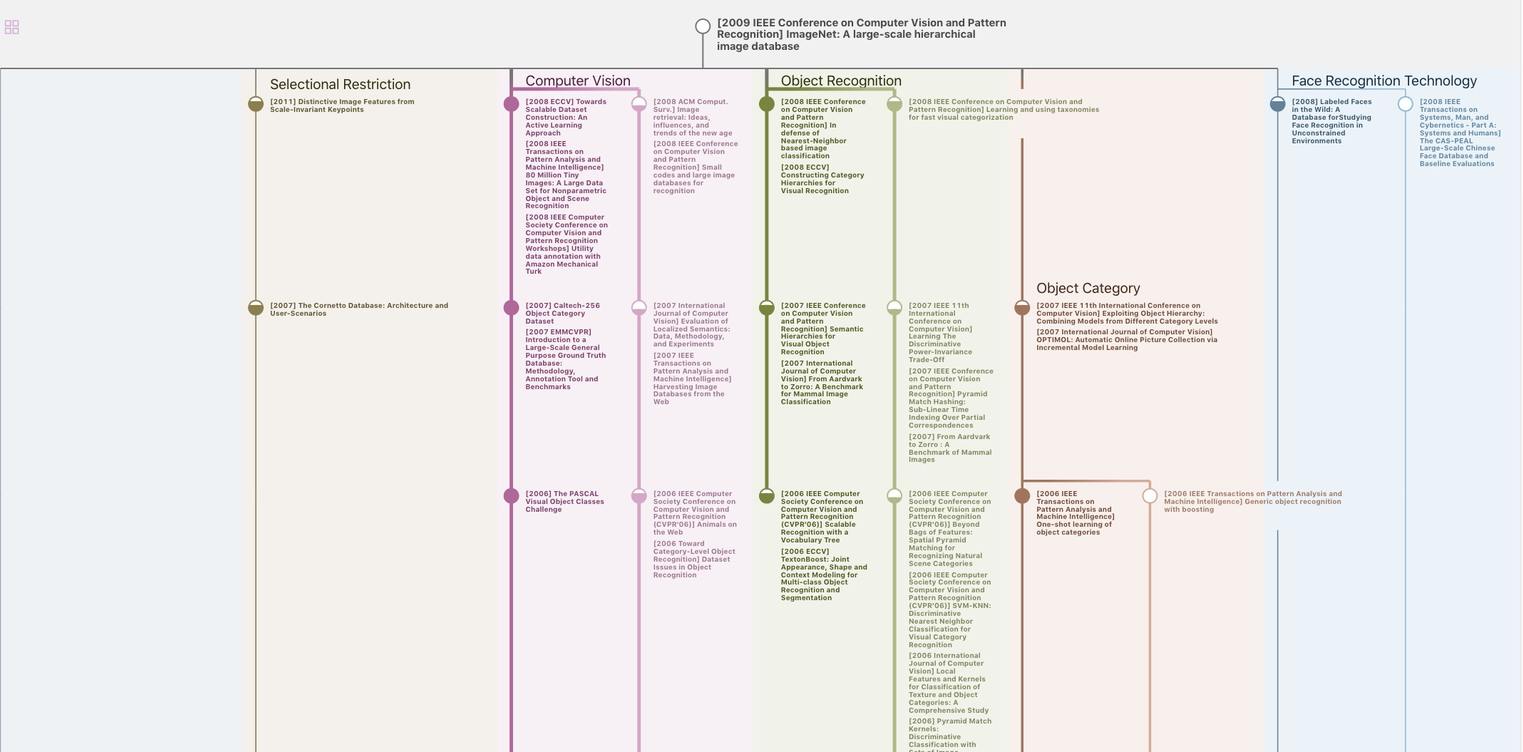
生成溯源树,研究论文发展脉络
Chat Paper
正在生成论文摘要