Improving Latent Factor Models via Personalized Feature Projection for One Class Recommendation.
CIKM'15: 24th ACM International Conference on Information and Knowledge Management Melbourne Australia October, 2015(2015)
摘要
Latent Factor models, which transform both users and items into the same latent feature space, are one of the most successful and ubiquitous models in recommender systems. Most existing models in this paradigm define both users' and items' latent factors to be of the same size and use an inner product to represent a user's "compatibility" with an item. Intuitively, users' factors encode "preferences" while item factors encode "properties", so that the inner product encodes how well an item matches a user's preferences. However, a user's opinion of an item may be more complex, for example each dimension of each user's opinion may depend on a combination of multiple item factors simultaneously. Thus it may be better to view each dimension of a user's preference as a personalized projection of an item's properties so that the preference model can capture complex relationships between items' properties and users' preferences.
Therefore, in this paper we propose a novel personalized feature projection method to model users' preferences over items. Specifically, for each user, we define a personalized projection matrix, which takes the place of user-specific factors from existing models. This matrix describes a mapping between items' factors and users' preferences in order to build personalized preference models for each user and item. The proposed personalized feature projection method is quite general and existing latent factor models, for example, can be cast as a special case. We present three objective functions to optimize predictions in the form of ranked lists of users' preferences over items, and demonstrate how each can be used to improve one-class recommendation performance. Experiments are conducted on four real-world datasets and our results show that our personalized feature projection method outperforms several state-of-the-art methods on various evaluation metrics.
更多查看译文
AI 理解论文
溯源树
样例
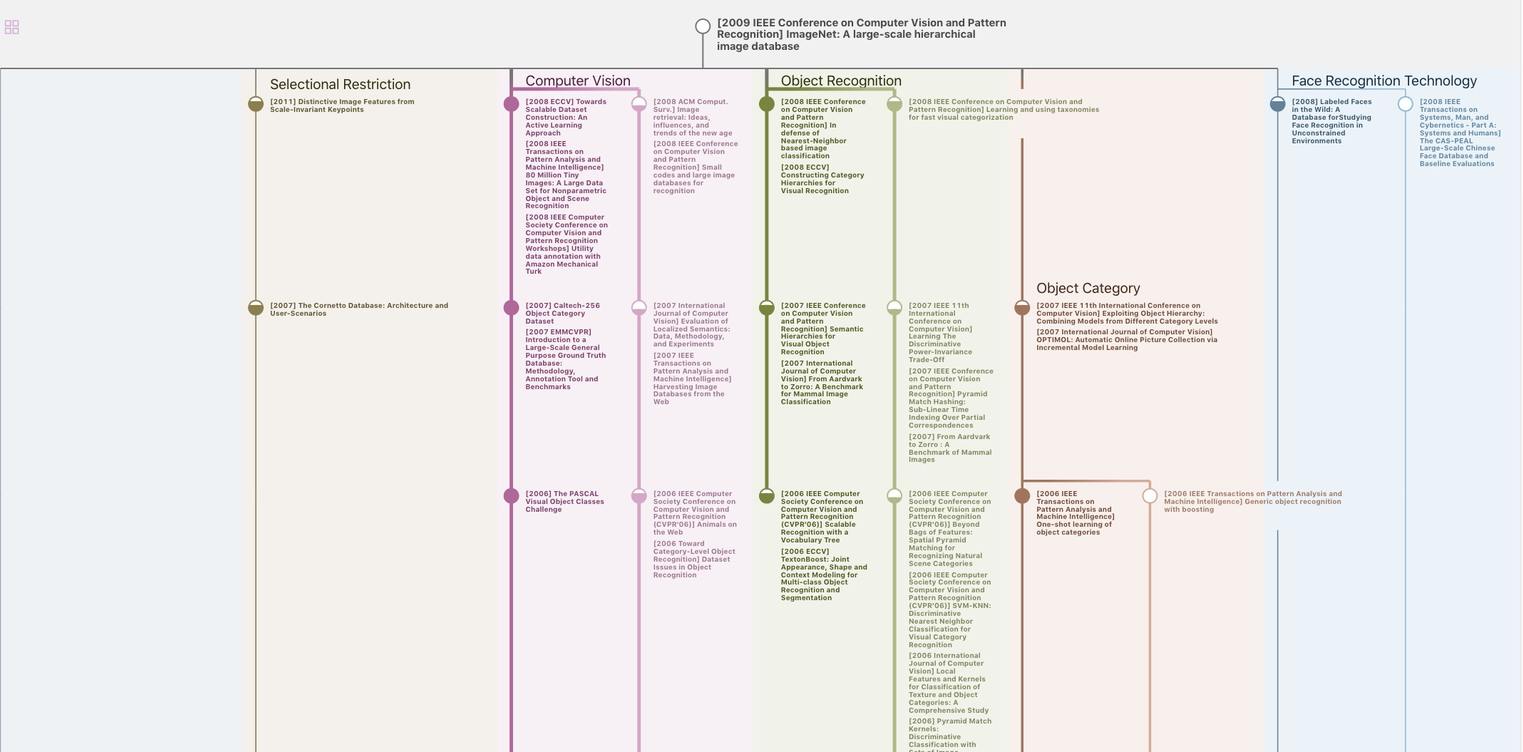
生成溯源树,研究论文发展脉络
Chat Paper
正在生成论文摘要