Unsupervised Dimension Reduction Methods for Protein Sequence Classification.
Studies in Classification Data Analysis and Knowledge Organization(2014)
摘要
Feature extraction methods are widely applied in order to reduce the dimensionality of data for subsequent classification, thus decreasing the risk of noise fitting. Principal Component Analysis (PCA) is a popular linear method for transforming high-dimensional data into a low-dimensional representation. Non-linear and non-parametric methods for dimension reduction, such as Isomap, Stochastic Neighbor Embedding (SNE) and Interpol are also used. In this study, we compare the performance of PCA, Isomap, t-SNE and Interpol as preprocessing steps for classification of protein sequences. Using random forests, we compared the classification performance on two artificial and eighteen real-world protein data sets, including HIV drug resistance, HIV-1 co-receptor usage and protein functional class prediction, preprocessed with PCA, Isomap, t-SNE and Interpol. Significant differences between these feature extraction methods were observed. The prediction performance of Interpol converges towards a stable and significantly higher value compared to PCA, Isomap and t-SNE. This is probably due to the nature of protein sequences, where amino acid are often dependent from and affect each other to achieve, for instance, conformational stability. However, visualization of data reduced with Interpol is rather unintuitive, compared to the other methods. We conclude that Interpol is superior to PCA, Isomap and t-SNE for feature extraction previous to classification, but is of limited use for visualization.
更多查看译文
关键词
Principal Component Analysis, Random Forest, Prediction Performance, Feature Extraction Method, High Prediction Performance
AI 理解论文
溯源树
样例
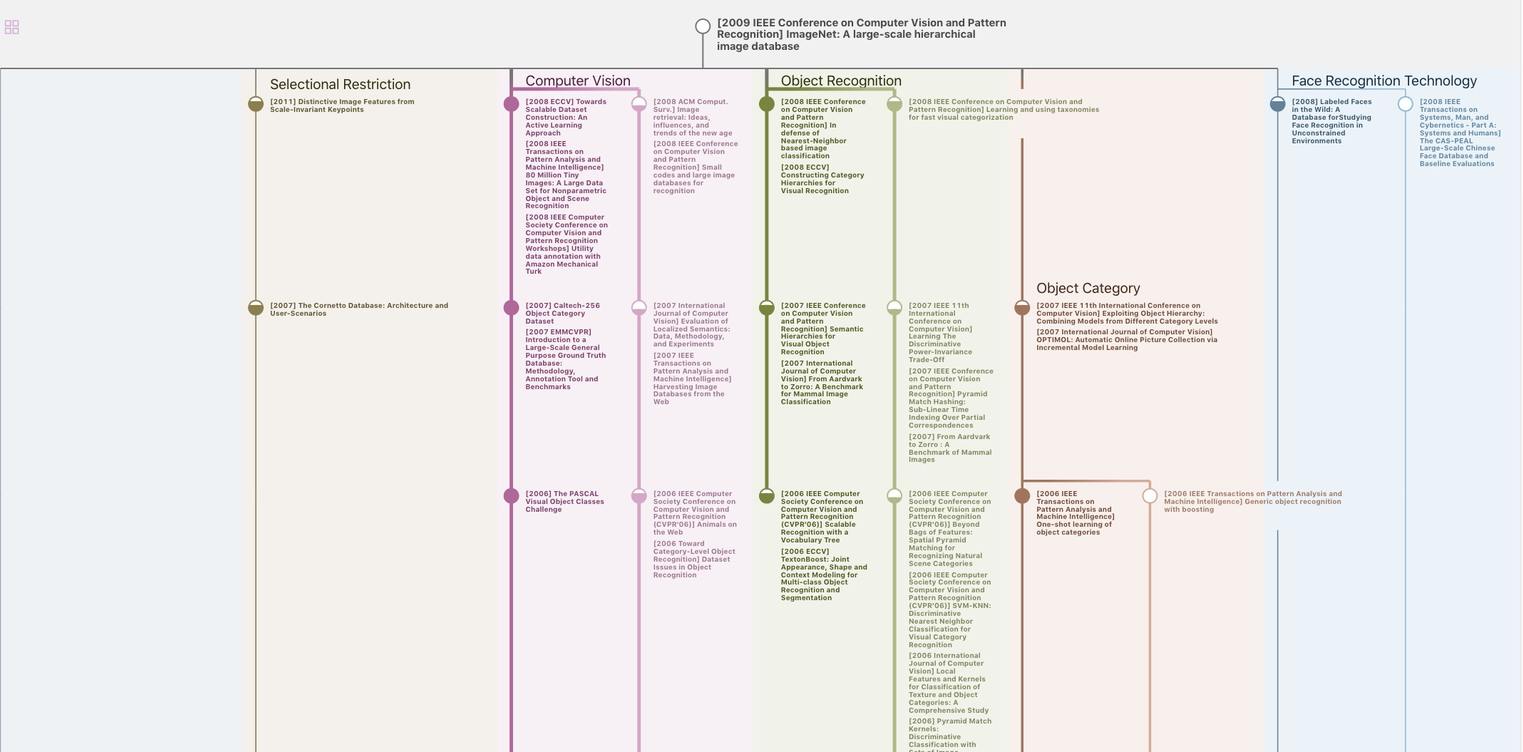
生成溯源树,研究论文发展脉络
Chat Paper
正在生成论文摘要