LabelRankT: Incremental Community Detection in Dynamic Networks via Label Propagation
SIGMOD/PODS'13: International Conference on Management of Data New York New York June, 2013(2013)
摘要
An increasingly important challenge in network analysis is efficient detection and tracking of communities in dynamic networks for which changes arrive as a stream. There is a need for algorithms that can incrementally update and monitor communities whose evolution generates huge realtime data streams, such as the Internet or on-line social networks. In this paper, we propose LabelRankT, an online distributed algorithm for detection of communities in large-scale dynamic networks through stabilized label propagation. Results of tests on real-world networks demonstrate that LabelRankT has much lower computational costs than other algorithms. It also improves the quality of the detected communities compared to dynamic detection methods and matches the quality achieved by static detection approaches. Unlike most of other algorithms which apply only to binary networks, LabelRankT works on weighted and directed networks, which provides a flexible and promising solution for real-world applications.
更多查看译文
AI 理解论文
溯源树
样例
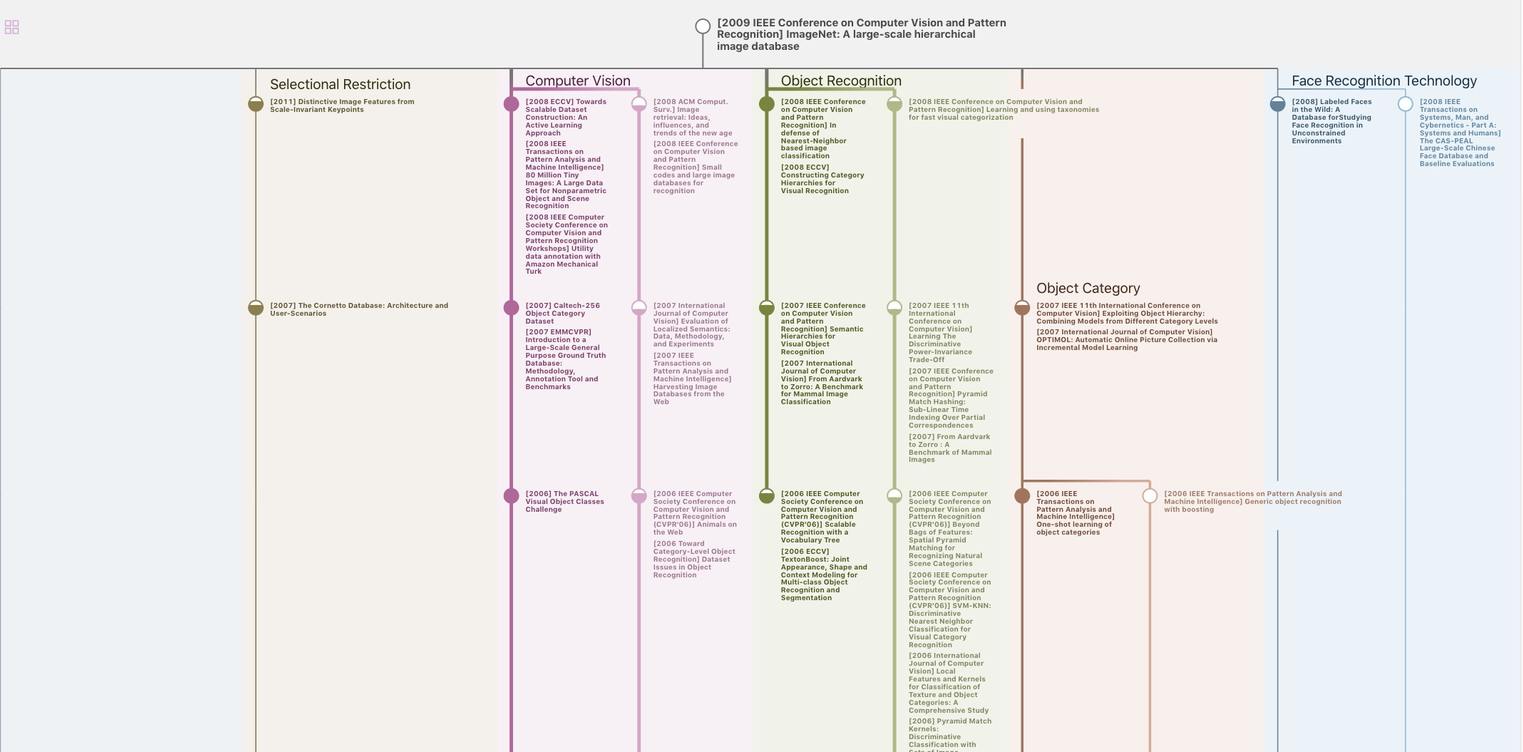
生成溯源树,研究论文发展脉络
Chat Paper
正在生成论文摘要