The Importance Of Being Expert: Efficient Max-Finding In Crowdsourcing
SIGMOD/PODS'15: International Conference on Management of Data Melbourne Victoria Australia May, 2015(2015)
摘要
Crowdsourcing is a computational paradigm whose distinctive feature is the involvement of human workers in key steps of the computation. It is used successfully to address problems that would be hard or impossible to solve for machines. As we highlight in this work, the exclusive use of nonexpert individuals may prove ineffective in some cases, especially when the task at hand or the need for accurate solutions demand some degree of specialization to avoid excessive uncertainty and inconsistency in the answers. We address this limitation by proposing an approach that combines the wisdom of the crowd with the educated opinion of experts. We present a computational model for crowdsourcing that envisions two classes of workers with different expertise levels. One of its distinctive features is the adoption of the threshold error model, whose roots are in psychometrics and which we extend from previous theoretical work. Our computational model allows to evaluate the performance of crowdsourcing algorithms with respect to accuracy and cost. We use our model to develop and analyze an algorithm for approximating the best, in a broad sense, of a set of elements. The algorithm uses naive and expert workers to find an element that is a constant- factor approximation to the best. We prove upper and lower bounds on the number of comparisons needed to solve this problem, showing that our algorithm uses expert and naive workers optimally up to a constant factor. Finally, we evaluate our algorithm on real and synthetic datasets using the CrowdFlower crowdsourcing platform, showing that our approach is also effective in practice.
更多查看译文
关键词
Crowdsourcing,human computation,max algorithm,worker models
AI 理解论文
溯源树
样例
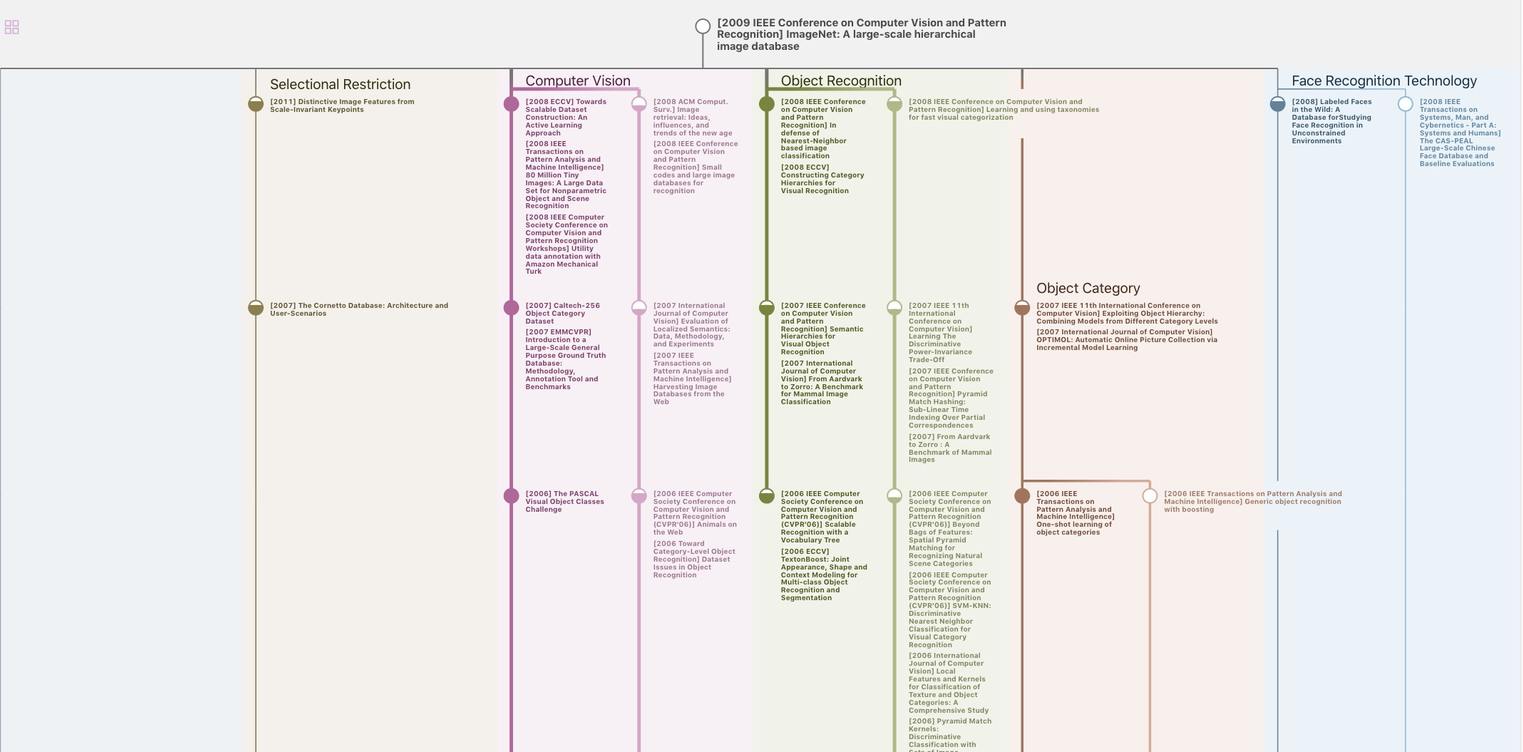
生成溯源树,研究论文发展脉络
Chat Paper
正在生成论文摘要