A 640M pixel/s 3.65mW sparse event-driven neuromorphic object recognition processor with on-chip learning
Symposium on VLSI Circuits-Digest of Papers(2015)
摘要
A 1.82mm(2) 65nm neuromorphic object recognition processor is designed using a sparse feature extraction inference module (IM) and a task-driven dictionary classifier. To achieve a high throughput, the 256-neuron IM is organized in four parallel neural networks to process four image patches and generate sparse neuron spikes. The on-chip classifier is activated by sparse neuron spikes to infer the object class, reducing its power by 88% and simplifying its implementation by removing all multiplications. A light-weight co-processor performs efficient on-chip learning by taking advantage of sparse neuron activity to save 84% of its workload and power. The test chip processes 10.16G pixel/s, dissipating 268mW. Integrated IM and classifier provides extra error tolerance for voltage scaling, lowering power to 3.65mW at a throughput of 640M pixel/s.
更多查看译文
关键词
sparse event-driven neuromorphic object recognition processor,on-chip learning,sparse feature extraction inference module,task-driven dictionary classifier,parallel neural networks,image patches,sparse neuron spikes,on-chip classifier,voltage scaling,power 3.65 mW,size 1.82 mm,size 65 nm,power 268 mW
AI 理解论文
溯源树
样例
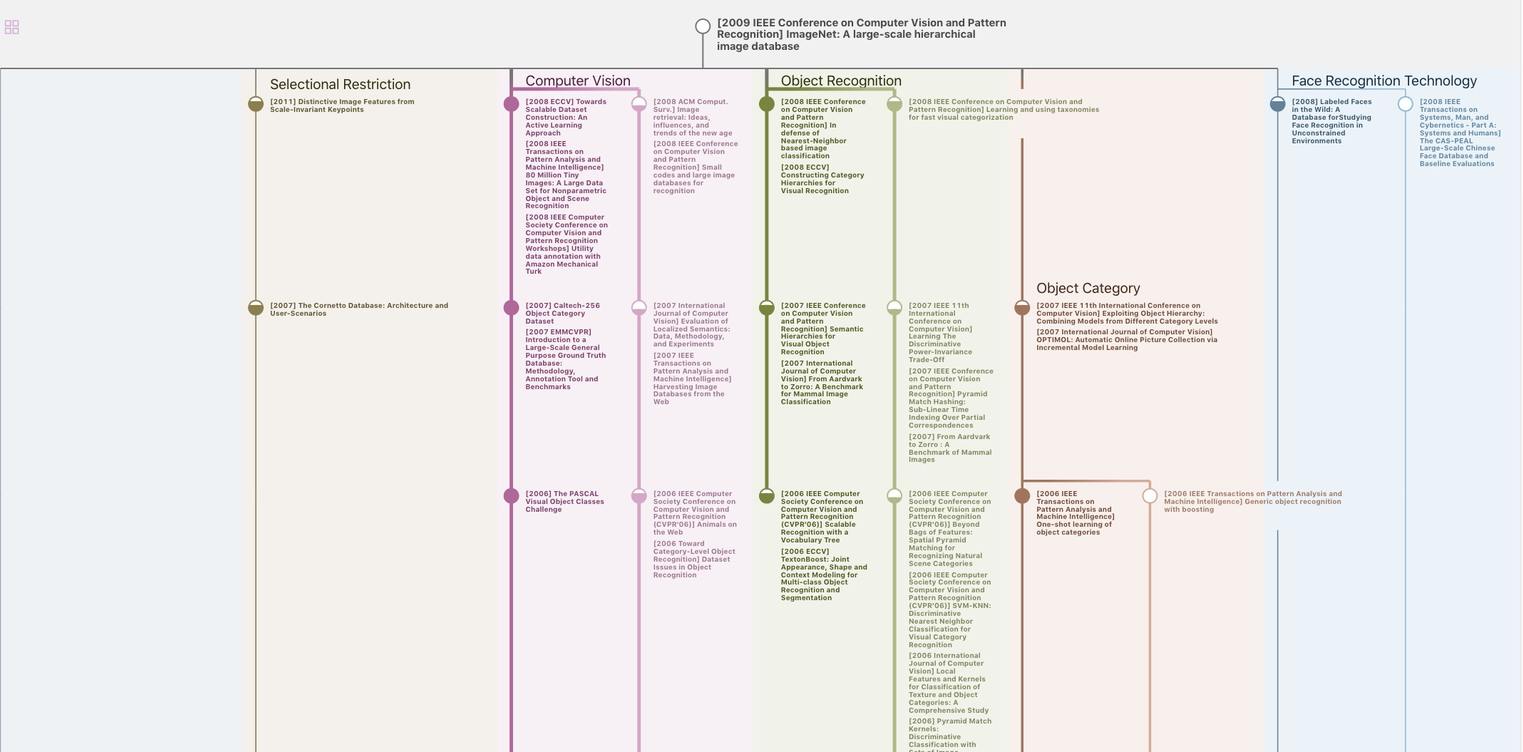
生成溯源树,研究论文发展脉络
Chat Paper
正在生成论文摘要