A 6.67mW sparse coding ASIC enabling on-chip learning and inference
VLSIC(2014)
摘要
A sparse coding ASIC is designed to learn visual receptive fields and infer the sparse representation of images for encoding, feature detection and recognition. 256 leaky integrate-and-fire neurons are connected in a 2-layer network of 2D local grids linked in a 4-stage systolic ring to reduce the communication latency. Spike collisions are kept sparse enough to be tolerated to save power. Memory is divided into a core section to support inference, and an auxiliary section that is only powered on for learning. An approximate learning tracks only significant neuron activities to save memory and power. The 3.06mm2 65nm CMOS ASIC achieves an inference throughput of 1.24Gpixel/s at 1.0V and 310MHz, and on-chip learning can be completed in seconds. Memory supply voltage can be reduced to 440mV to exploit the soft algorithm that tolerates errors, reducing the inference power to 6.67mW for a 140Mpixel/s throughput at 35MHz.
更多查看译文
关键词
CMOS integrated circuits,application specific integrated circuits,feature extraction,image coding,image representation,integrated circuit design,learning (artificial intelligence),neural chips,systolic arrays,2-layer network,2D local grids,4-stage systolic ring,CMOS ASIC,communication latency reduction,encoding,feature detection,feature recognition,frequency 310 MHz,frequency 35 MHz,leaky integrate-and-fire neurons,memory supply voltage,on-chip inference,on-chip learning,power 6.67 mW,size 65 nm,soft algorithm,sparse coding ASIC,sparse image representation,spike collisions,visual receptive field learning,voltage 1.0 V,voltage 440 mV,
AI 理解论文
溯源树
样例
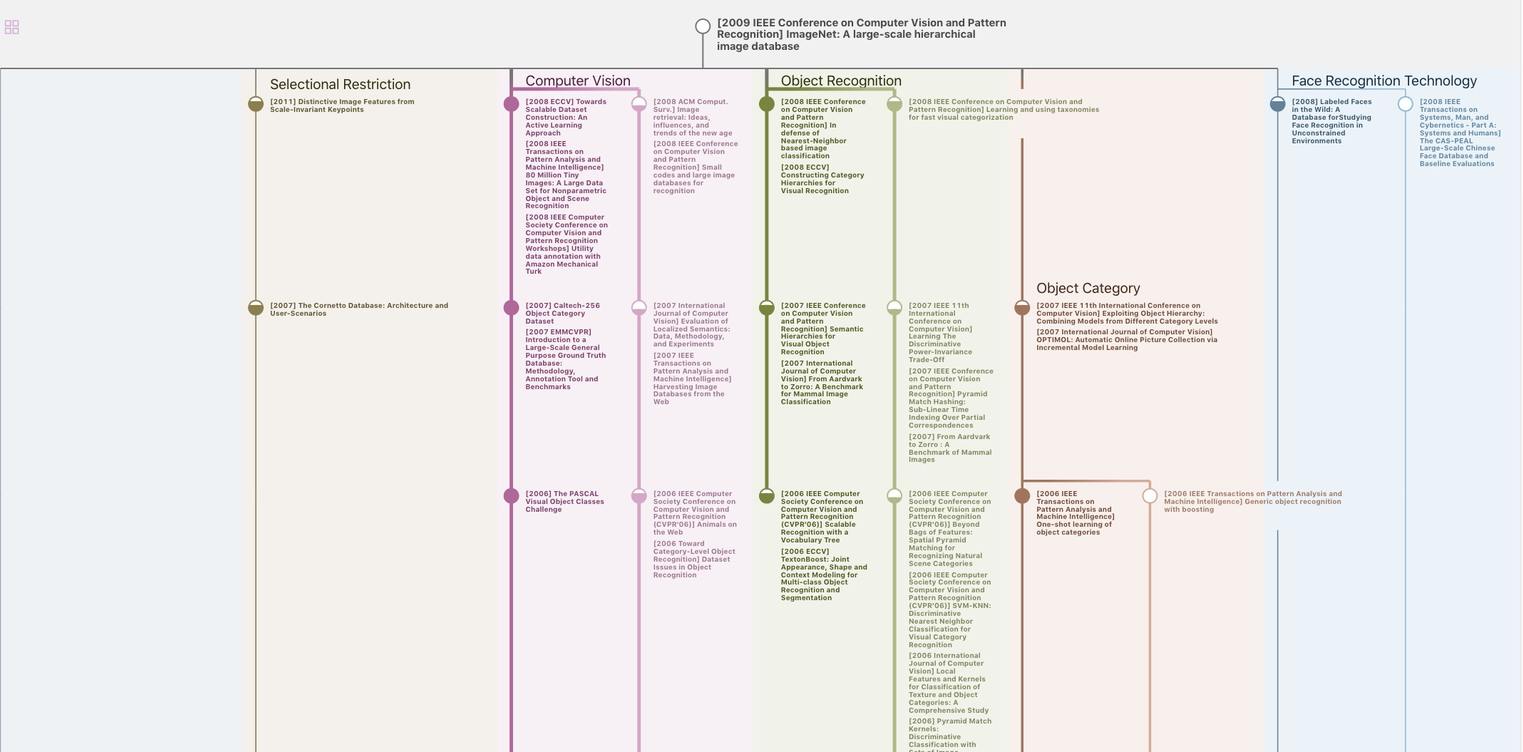
生成溯源树,研究论文发展脉络
Chat Paper
正在生成论文摘要