Proof-reading guidance in cell tracking by sampling from tracking-by-assignment models
IEEE International Symposium on Biomedical Imaging(2015)
摘要
Automated cell tracking methods are still error-prone. On very large data sets, uncertainty measures are thus needed to guide the expert to the most ambiguous events so these can be corrected with minimal effort. We present two easy-to-use methods to sample multiple proposal solutions from a tracking-by-assignment graphical model and experimentally evaluate the benefits of the uncertainty measures derived. Expert time for proof-reading is reduced greatly compared to random selection of predicted events.
更多查看译文
关键词
Cell tracking, uncertainty, machine learning, probabilistic graphical models
AI 理解论文
溯源树
样例
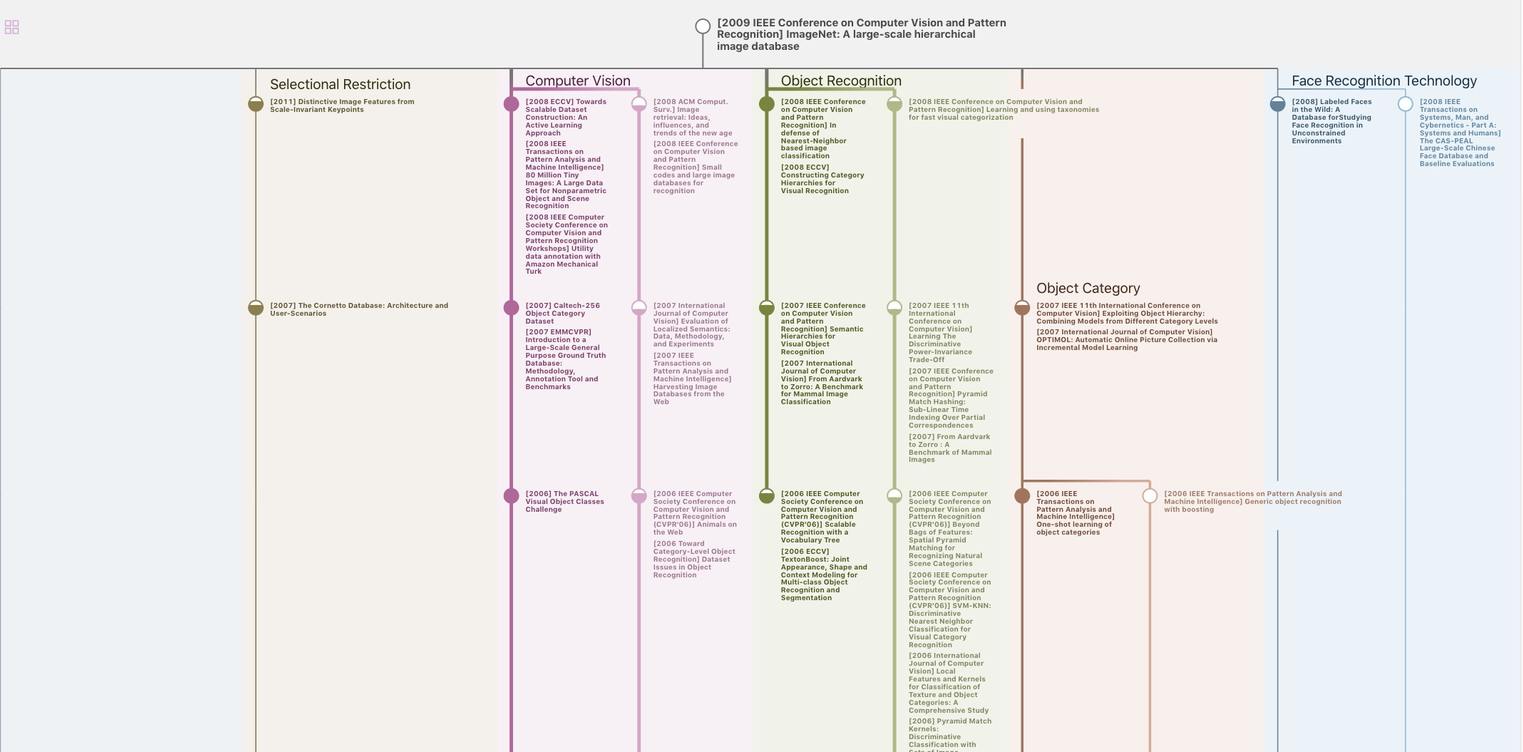
生成溯源树,研究论文发展脉络
Chat Paper
正在生成论文摘要