Constrained Local Model With Independent Component Analysis And Kernel Density Estimation: Application To Down Syndrome Detection
2015 IEEE 12th International Symposium on Biomedical Imaging (ISBI)(2015)
摘要
Statistical shape models generally characterize shape variations linearly by principal component analysis (PCA), which assumes that the non-rigid shape parameters are drawn from a Gaussian distribution. This practical assumption is often not valid. Instead, we propose a constrained local model based on independent component analysis (ICA) and use kernel density estimation (KDE) for non-parametrically modeling the distribution of the shape parameters. The model fitting is achieved by maximum a posteriori via the expectation-maximization algorithm and results in a mean shift-like update optimizer. The proposed approach is capable of modeling non-Gaussian shape priors and significantly outperformed the PCA-based model (p=0.03) and ICA-based model with Gaussian shape prior (p=0.01) in experiments to detect facial landmarks. Moreover, we applied the model to Down syndrome detection from frontal facial photographs and obtained higher accuracy than the best results reported in literature.
更多查看译文
关键词
Constrained Local Model,Non-Parametric Shape Prior,Kernel Density Estimation,Independent Component Analysis,Down syndrome
AI 理解论文
溯源树
样例
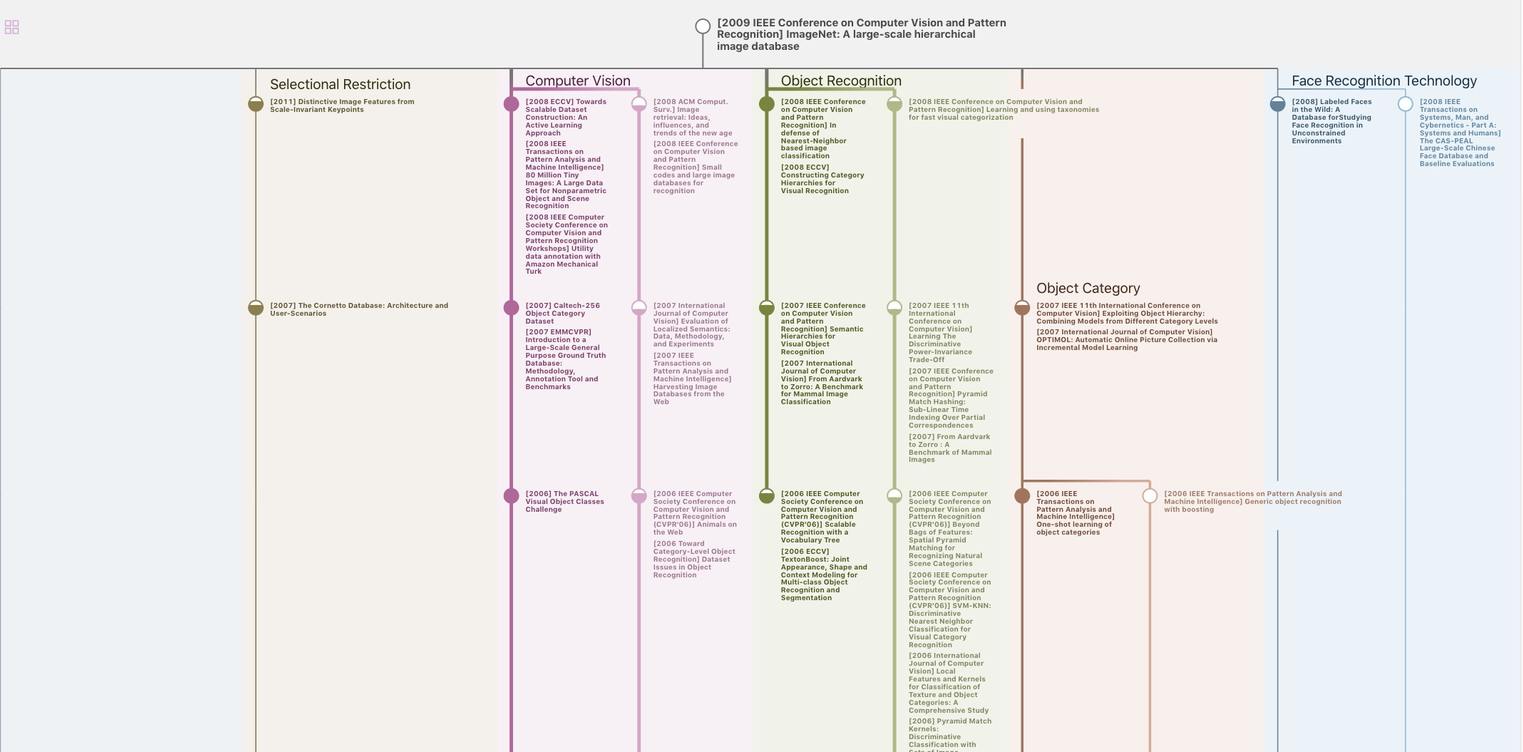
生成溯源树,研究论文发展脉络
Chat Paper
正在生成论文摘要