Prediction of radioprotectors targeting p53 for suppression of acute effect of cancer radiotherapy using machine learning
IEEE International Conference on Bioinformatics and Biomedicine(2015)
摘要
Radiation therapy and some chemotherapeutic agents mainly target the DNA of growing cancer cells, whereas these therapies have adverse side effects, including p53-induced apoptosis of normal tissues and cells. It is considered that p53 would be a target for therapeutic and mitigative radioprotection to escape from the apoptotic fate. So far, only three radioprotective p53 inhibitors have been reported, namely, pifithrin-α (PFTα), pifithrin-μ (PFTμ), and sodium orthovanadate (vanadate), which protect mice from acute lethality due to hematopoietic syndrome, indicating that pharmacologically temporary suppression of p53 effectively minimize the radiation damage. In this study, we examined the inhibitory activity of some zinc(II) chelators against radiation-induced apoptosis of MOLT-4 cells, based on the assumption that the binding of these compounds to zinc(II) in p53 proteins or removal of zinc(II) from the protein would temporally inhibit the function of p53. However, we have had some problems. The development of drug has been slow, due to the time required and the high cost of screening candidate compounds. It is possible to efficiently search for drugs by using machine learning. So we predict compounds that radioprotectors using Random Forest to study compound futures and using other machine learning methods for comparison with Random Forest. Procedure of learning is as follows: First, compounds were divided into several groups based on the toxicity and protection capability. Next, it was performed classification using machine learning. These results may contribute to discover of new radioprotectors.
更多查看译文
关键词
Machine Learning, Random Forest, Sepport Vector Machinem, Radioprotector
AI 理解论文
溯源树
样例
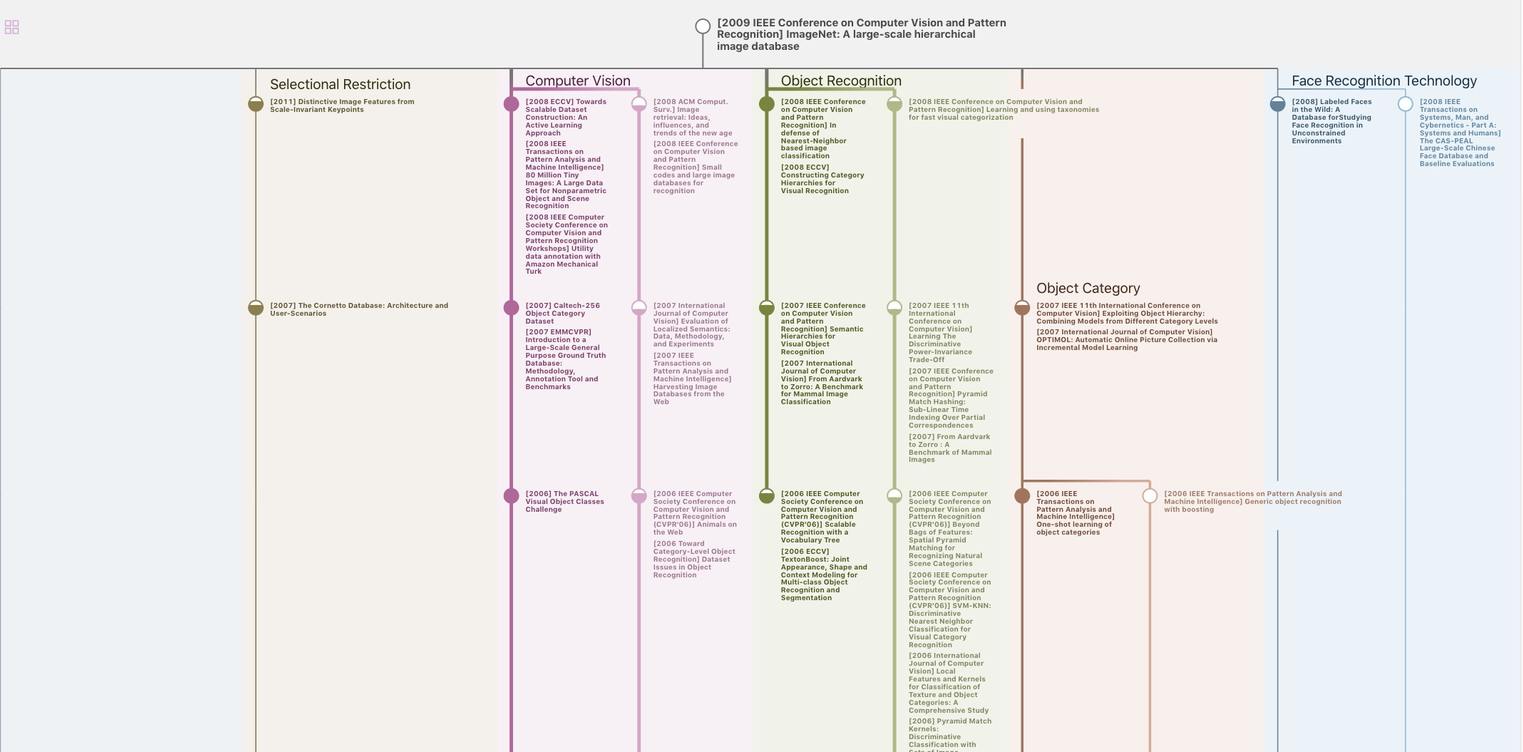
生成溯源树,研究论文发展脉络
Chat Paper
正在生成论文摘要