Developing Cancer Prediction Model Based on Stepwise Selection by Auc Measure for Proteomics Data
IEEE International Conference on Bioinformatics and Biomedicine(2015)
摘要
Since most of the cancer markers that have been reported are obtained directly from cancer tissues, it is difficult to use them for early diagnosis of cancer without surgery. Thus, development of markers that can be detected by blood is crucial for making early diagnosis of cancer easier. One of the most feasible types of markers that can be detected by blood is a protein marker. Here, we focus on building prediction methods using the protein markers for early diagnosis of cancer. To develop a prediction model with high prediction ability, it is critical to choose appropriate markers first. Here, we consider a stepwise selection method using area under the receiver operating characteristic curve (Step-AUC) in order to construct a multi-protein prediction model. We showed that the performance of Step-AUC highly depends on the tuning parameter. We compared our proposed Step-AUC method to stepwise selection using information criteria and support vector machine recursive feature extraction (SVM-RFE). We observed that Step-AUC and stepwise selection using Bayesian information criteria (Step-BIC) perform better than other methods. The importance of each marker can be chosen using a new stepwise selection consistency (SSC) measure. The final models include the markers with high SSC measures. We applied our stepwise procedure to pancreatic cancer data and found two markers of interest.
更多查看译文
关键词
early diagnosis of cancer,protein marker,stepwise selection,Receiver operating characteristic (ROC) curve,area under the curve (AUC),nmltiple reaction monitoring (MRM),support vector machine
AI 理解论文
溯源树
样例
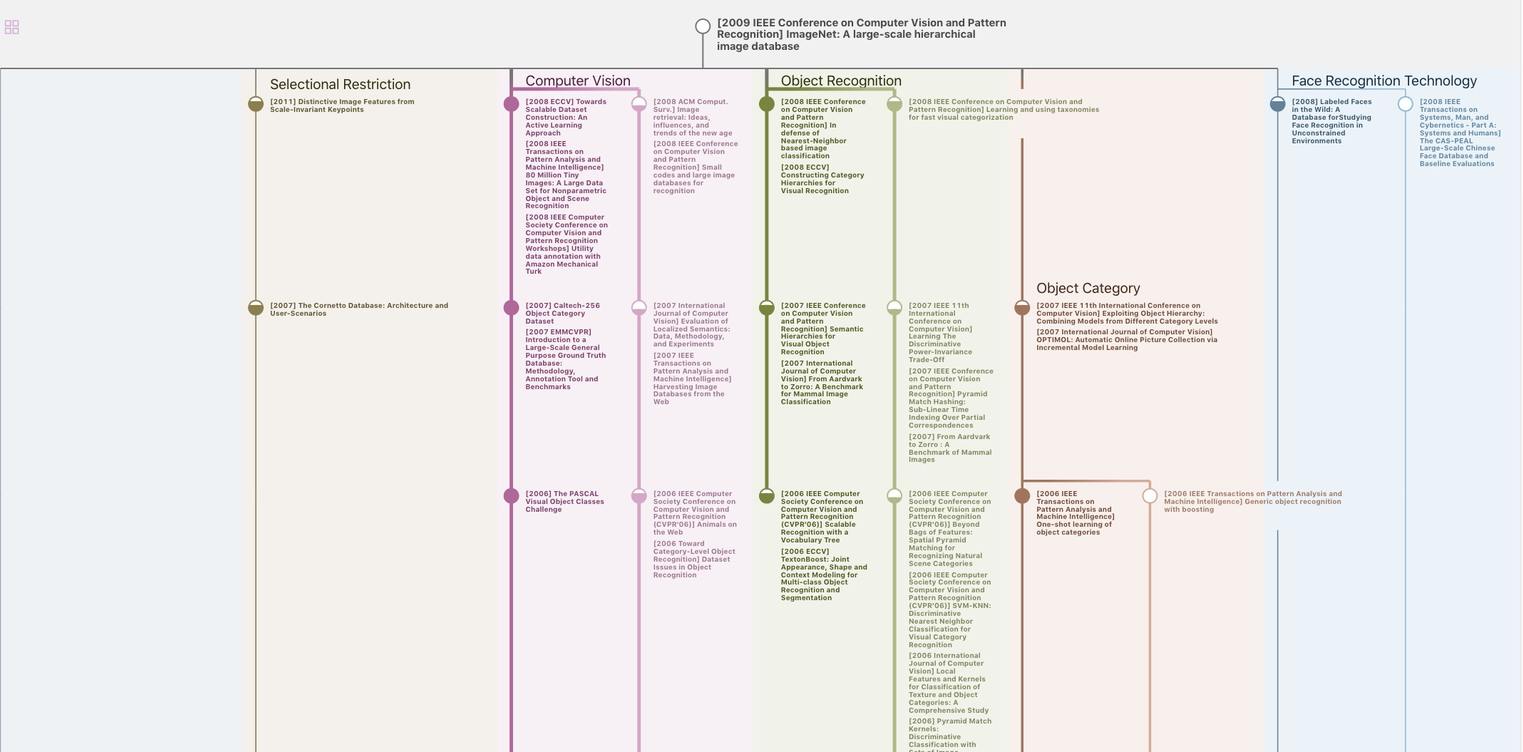
生成溯源树,研究论文发展脉络
Chat Paper
正在生成论文摘要