Joint inference of tissue-specific networks with a scale free topology
IEEE International Conference on Bioinformatics and Biomedicine(2015)
摘要
High-throughput experimental techniques have produced an enormous number of gene expression profiles for various tissues of the human body. Tissue-specificity is a key component in reflecting the potentially different roles of proteins in diverse cell lineages. One way of understanding the tissue specificity is by reconstructing the tissue-specific co-expression networks (CENs) to analyze the correlation between genes. A few methods have been developed for estimating CENs, but it still remains challenging in terms of both accuracy and efficiency. In this paper we propose a new method, JointNet, for predicting tissue-specific co-expression networks. JointNet is exploiting the observation that, functionally related tissues have similar expression patterns and thus, similar networks. It uses different node penalties for hubs and non-hub nodes to accurately estimate the scale-free networks. Our experimental results show that the resulting tissue-specific CENs are accurate and that our method outperforms the current state of the art.
更多查看译文
关键词
nonhub nodes,node penalties,similar expression patterns,JointNet,tissue-specific coexpression networks,cell lineages,human body,gene expression profiles,high-throughput experimental techniques,scale free topology,tissue-specific networks joint inference
AI 理解论文
溯源树
样例
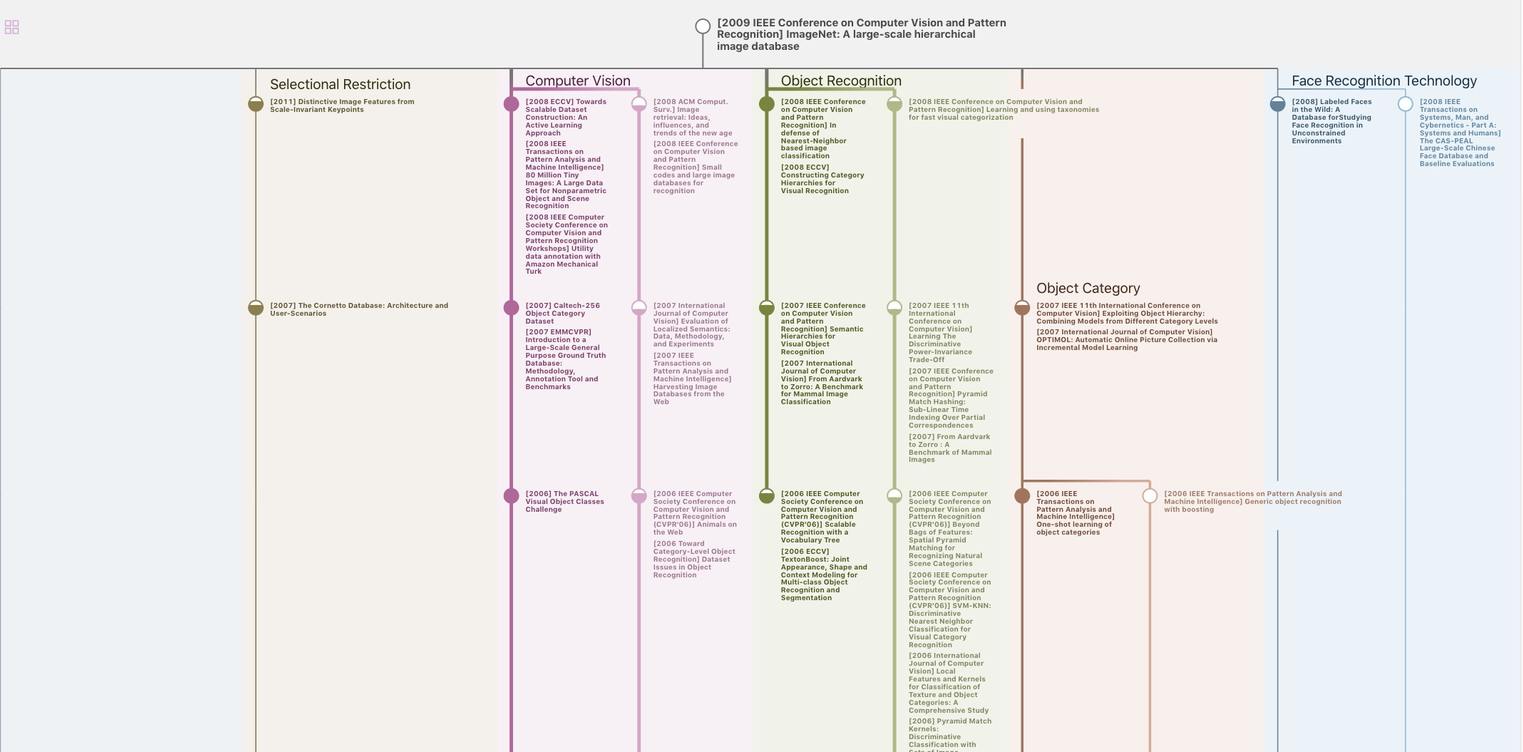
生成溯源树,研究论文发展脉络
Chat Paper
正在生成论文摘要