The Wisdom of Multiple Guesses.
EC(2015)
摘要
ABSTRACTThe "wisdom of crowds" dictates that aggregate predictions from a large crowd can be surprisingly accurate, rivaling predictions by experts. Crowds, meanwhile, are highly heterogeneous in their expertise. In this work, we study how the heterogeneous uncertainty of a crowd can be directly elicited and harnessed to produce more efficient aggregations from a crowd, or provide the same efficiency from smaller crowds. We present and evaluate a novel strategy for eliciting sufficient information about an individual's uncertainty: allow individuals to make multiple simultaneous guesses, and reward them based on the accuracy of their closest guess. We show that our multiple guesses scoring rule is an incentive-compatible elicitation strategy for aggregations across populations under the reasonable technical assumption that the individuals all hold symmetric log-concave belief distributions that come from the same location-scale family. We first show that our multiple guesses scoring rule is strictly proper for a fixed set of quantiles of any log-concave belief distribution. With properly elicited quantiles in hand, we show that when the belief distributions are also symmetric and all belong to a single location-scale family, we can use interquantile ranges to furnish weights for certainty-weighted crowd aggregation. We evaluate our multiple guesses framework empirically through a series of incentivized guessing experiments on Amazon Mechanical Turk, and find that certainty-weighted crowd aggregations using multiple guesses outperform aggregations using single guesses without certainty weights.
更多查看译文
AI 理解论文
溯源树
样例
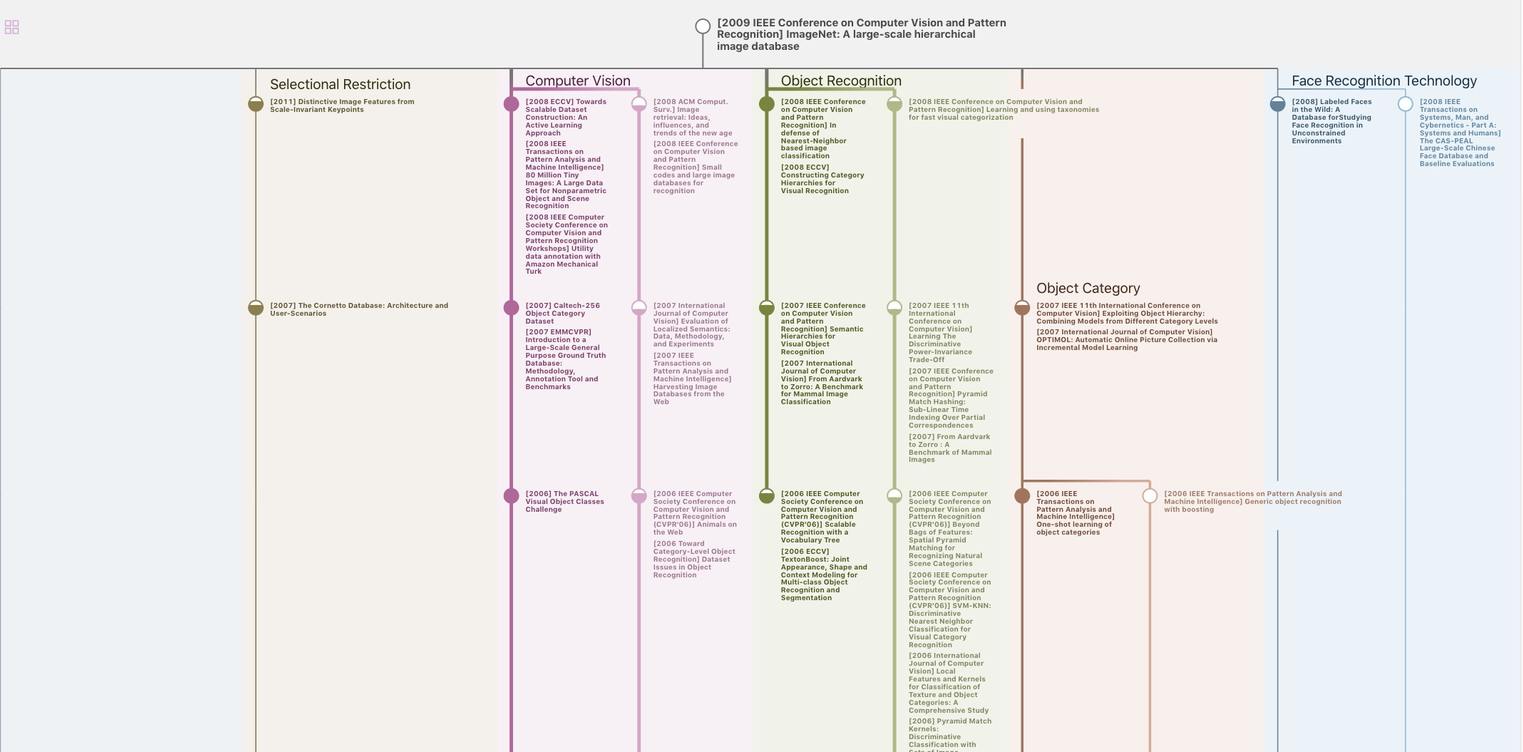
生成溯源树,研究论文发展脉络
Chat Paper
正在生成论文摘要