Minimizing hallucination in histogram of Oriented Gradients
2015 12th IEEE International Conference on Advanced Video and Signal Based Surveillance (AVSS)(2015)
摘要
Histogram of Oriented Gradients is one of the most extensively used image descriptors in computer vision. It has successfully been applied to various vision tasks such as localization, classification and recognition. As it mainly captures gradient strengths in an image, it is sensitive to local variations in illumination and contrast. In the result, a normalization of this descriptor turns out to be essential for good performance [3, 4]. Although different normalization schemes have been investigated, all of them usually employ L1 or L2-norm. In this paper we show that an incautious application of L-like norms to the HOG descriptor might produce a hallucination effect. To overcome this issue, we propose a new normalization scheme that effectively minimizes hallucinations. This scheme is built upon a detailed analysis of the gradient distribution resulting in adding an extra bin with a specific value that increases HOG distinctiveness. We validated our approach on person re-identification and action recognition, demonstrating significant boost in the performance.
更多查看译文
关键词
oriented gradient histogram,hallucination minimization,image descriptor,computer vision,L2-norm,L1-norm,gradient distribution,action recognition,person reidentification,HOG descriptor
AI 理解论文
溯源树
样例
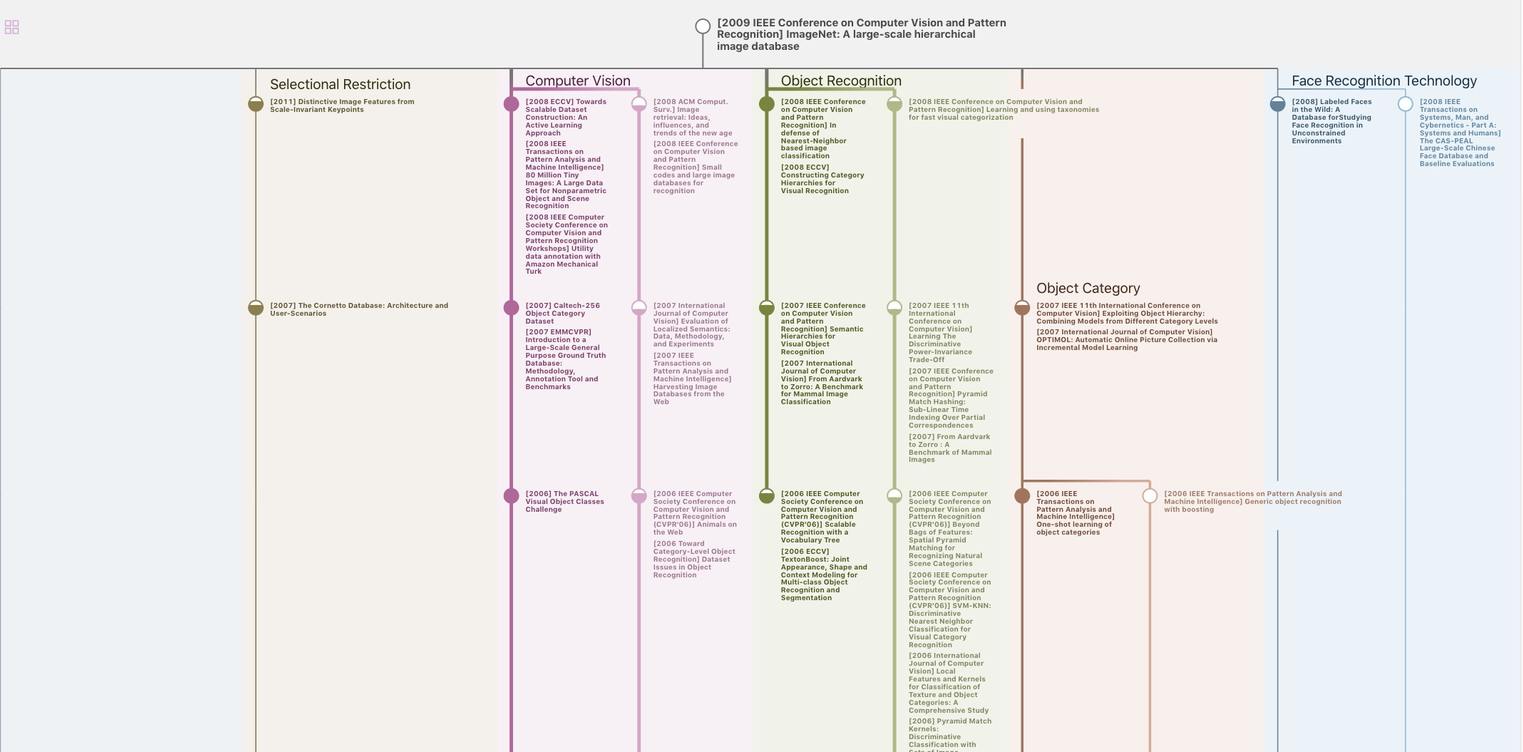
生成溯源树,研究论文发展脉络
Chat Paper
正在生成论文摘要