Atom Decomposition with Adaptive Basis Selection Strategy for Matrix Completion.
TOMCCAP(2016)
摘要
Estimating missing entries in matrices has attracted much attention due to its wide range of applications like image inpainting and video denoising, which are usually considered as low-rank matrix completion problems theoretically. It is common to consider nuclear norm as a surrogate of the rank operator since it is the tightest convex lower bound of the rank operator under certain conditions. However, most approaches based on nuclear norm minimization involve a number of singular value decomposition (SVD) operations. Given a matrix X ∈ Rm×n, the time complexity of the SVD operation is O(mn2), which brings prohibitive computational burden on large-scale matrices, limiting the further usage of these methods in real applications. Motivated by this observation, a series of atom-decomposition-based matrix completion methods have been studied. The key to these methods is to reconstruct the target matrix by pursuit methods in a greedy way, which only involves the computation of the top SVD and has great advantages in efficiency compared with the SVD-based matrix completion methods. However, due to gradually serious accumulation errors, atom-decomposition-based methods usually result in unsatisfactory reconstruction accuracy. In this article, we propose a new efficient and scalable atom decomposition algorithm for matrix completion called Adaptive Basis Selection Strategy (ABSS). Different from traditional greedy atom decomposition methods, a two-phase strategy is conducted to generate the basis separately via different strategies according to their different nature. At first, we globally prune the basis space to eliminate the unimportant basis as much as possible and locate the probable subspace containing the most informative basis. Then, another group of basis spaces are learned to improve the recovery accuracy based on local information. In this way, our proposed algorithm breaks through the accuracy bottleneck of traditional atom-decomposition-based matrix completion methods; meanwhile, it reserves the innate efficiency advantages over SVD-based matrix completion methods. We empirically evaluate the proposed algorithm ABSS on real visual image data and large-scale recommendation datasets. Results have shown that ABSS has much better reconstruction accuracy with comparable cost to atom-decomposition-based methods. At the same time, it outperforms the state-of-the-art SVD-based matrix completion algorithms by similar or better reconstruction accuracy with enormous advantages on efficiency.
更多查看译文
关键词
Algorithms,Experimentation,Matrix completion,atom decomposition,basis selection
AI 理解论文
溯源树
样例
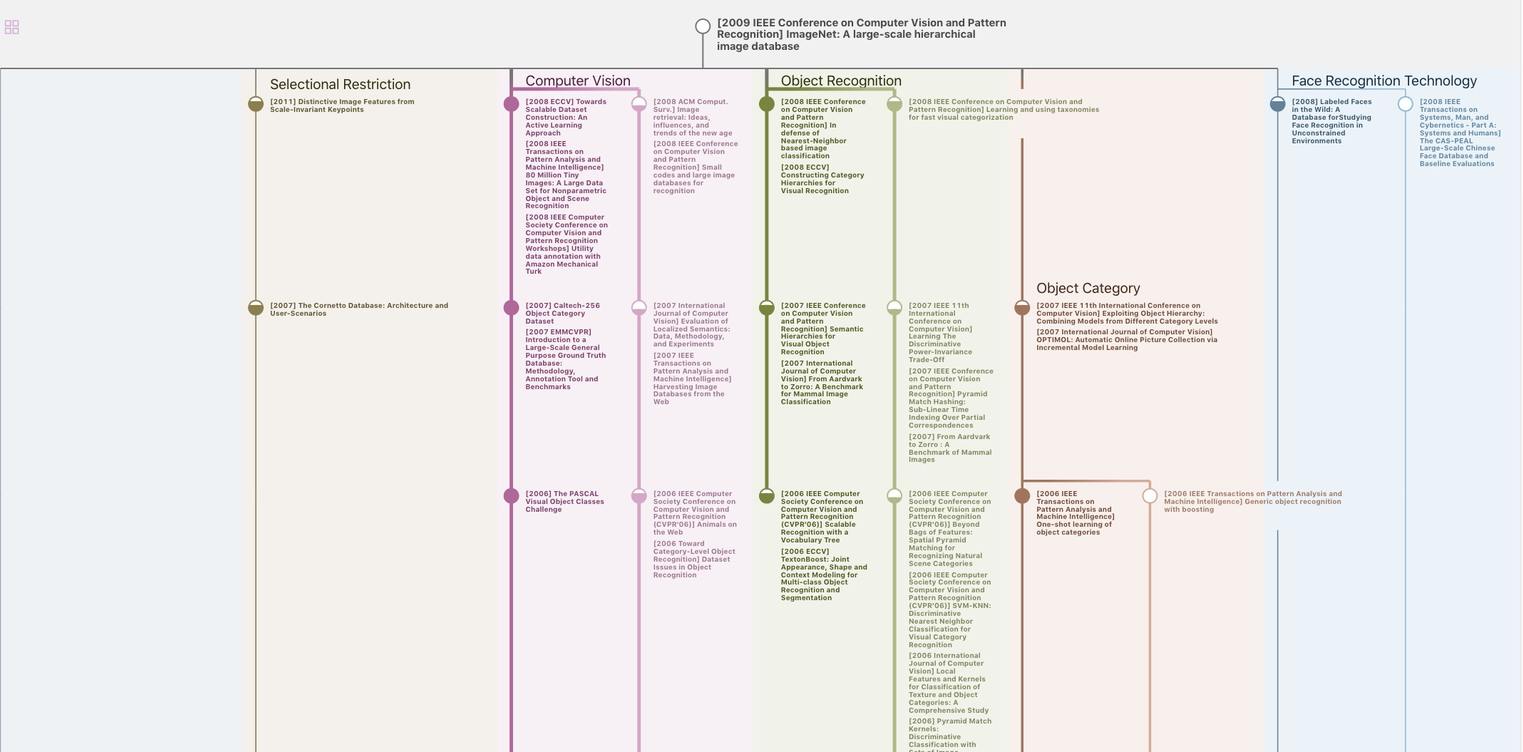
生成溯源树,研究论文发展脉络
Chat Paper
正在生成论文摘要