Normalized filter pool for prior modeling of nature images
Machine Vision and Applications(2016)
摘要
Markov random field (MRF), as one of special undirected graphs, is widely used in modeling priors of natural images. Targeting to learn better prior models from a given database, we explore the natural image statistics at different scales and build normalized filter pool, a kind of high-order MRF, for prior learning of nature images. The main contribution of the proposed model is that we construct a multi-scale MRF model through constraining the norms of filters in kernel space and integrate all the filtering responses in a unified framework. We formulate both learning and inference as constrained optimization problems and solve them using augmented Lagrange method. The experiment results demonstrate that the normalization of filters at different scales helps to achieve fast convergence in learning stage and obtain superior performance in image restoration, e.g., image denoising and image inpainting.
更多查看译文
关键词
High-order Markov random field,Multi-scale,Normalized filter pool,Image denoising,Image inpainting
AI 理解论文
溯源树
样例
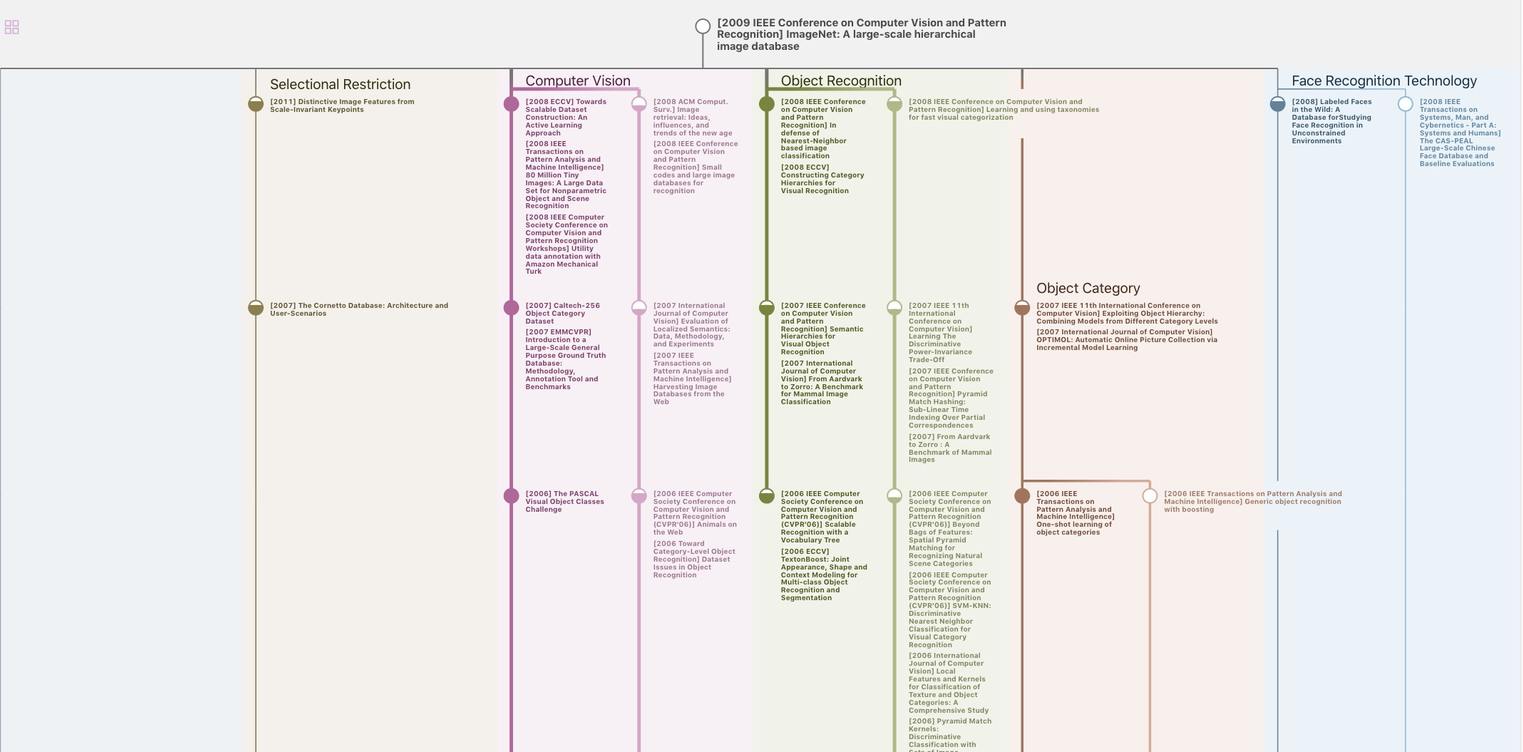
生成溯源树,研究论文发展脉络
Chat Paper
正在生成论文摘要