Hybrid analysis for indicating patients with breast cancer using temperature time series
Computer Methods and Programs in Biomedicine(2016)
摘要
HighlightsWe have proposed a hybrid automatic method for identifying patients with breast cancer, supporting human experts.We have applied learning techniques supervised and unsupervised learning techniques.We have applied various validity indexes of clusters.In the evaluation of the proposed method, a data mining tool was used to solve the combined algorithm selection and hyperparameter optimization (CASH) problem.Among the classification algorithms which have been applied, K-Star and Bayes Net has shown the best result, 100% accuracy. Breast cancer is the most common cancer among women worldwide. Diagnosis and treatment in early stages increase cure chances. The temperature of cancerous tissue is generally higher than that of healthy surrounding tissues, making thermography an option to be considered in screening strategies of this cancer type. This paper proposes a hybrid methodology for analyzing dynamic infrared thermography in order to indicate patients with risk of breast cancer, using unsupervised and supervised machine learning techniques, which characterizes the methodology as hybrid. The dynamic infrared thermography monitors or quantitatively measures temperature changes on the examined surface, after a thermal stress. In the dynamic infrared thermography execution, a sequence of breast thermograms is generated. In the proposed methodology, this sequence is processed and analyzed by several techniques. First, the region of the breasts is segmented and the thermograms of the sequence are registered. Then, temperature time series are built and the k-means algorithm is applied on these series using various values of k. Clustering formed by k-means algorithm, for each k value, is evaluated using clustering validation indices, generating values treated as features in the classification model construction step. A data mining tool was used to solve the combined algorithm selection and hyperparameter optimization (CASH) problem in classification tasks. Besides the classification algorithm recommended by the data mining tool, classifiers based on Bayesian networks, neural networks, decision rules and decision tree were executed on the data set used for evaluation. Test results support that the proposed analysis methodology is able to indicate patients with breast cancer. Among 39 tested classification algorithms, K-Star and Bayes Net presented 100% classification accuracy. Furthermore, among the Bayes Net, multi-layer perceptron, decision table and random forest classification algorithms, an average accuracy of 95.38% was obtained.
更多查看译文
关键词
Breast cancer,Dynamic infrared thermography,Temperature time series,Machine learning,Classification
AI 理解论文
溯源树
样例
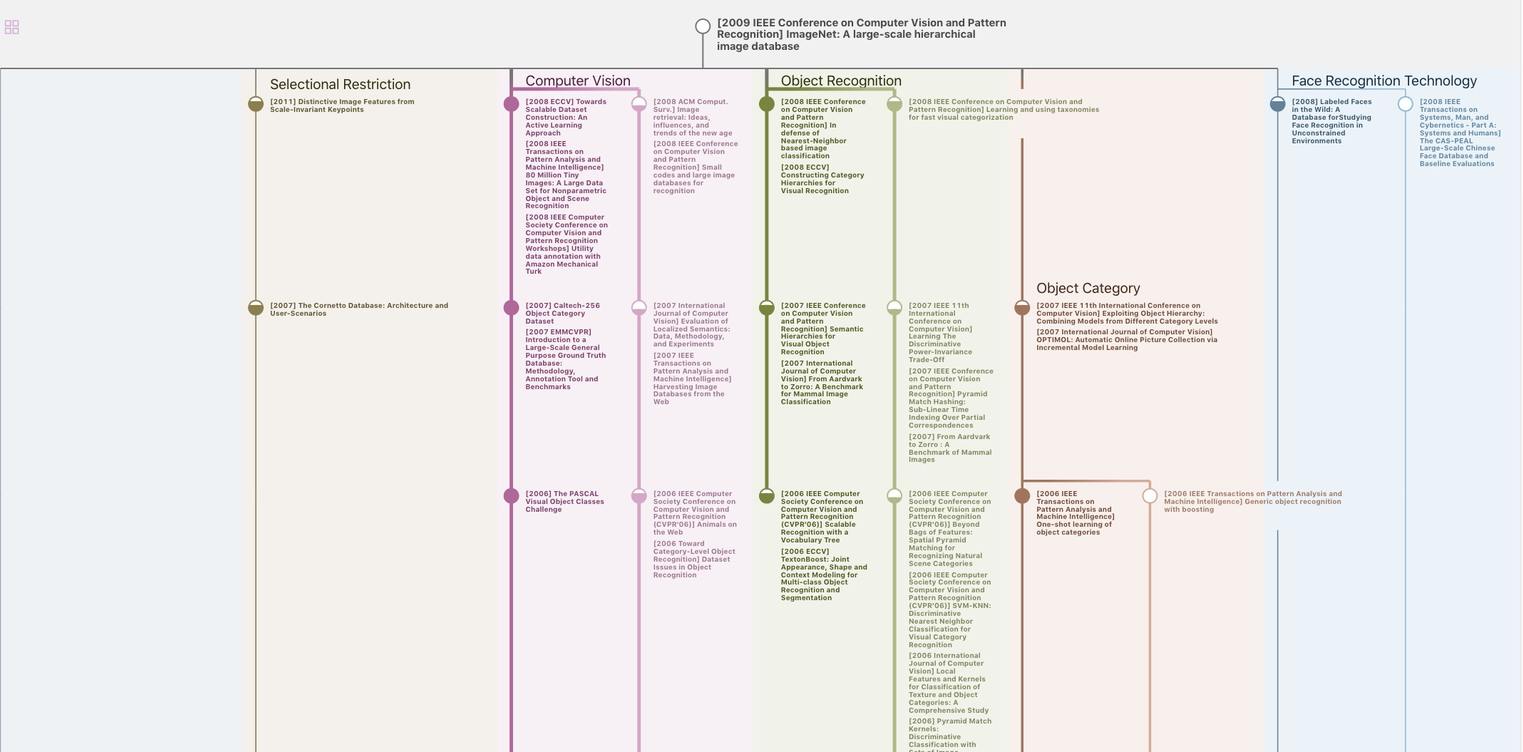
生成溯源树,研究论文发展脉络
Chat Paper
正在生成论文摘要