Empowering one-vs-one decomposition with ensemble learning for multi-class imbalanced data.
Knowl.-Based Syst.(2016)
摘要
Extending binary ensemble techniques to multi-class imbalanced data.OVO scheme enhancement for multi-class imbalanced data by ensemble learning.A complete experimental study of comparison of the ensemble learning techniques with OVO.Study of the impact of base classifiers used in the proposed scenario. Multi-class imbalance classification problems occur in many real-world applications, which suffer from the quite different distribution of classes. Decomposition strategies are well-known techniques to address the classification problems involving multiple classes. Among them binary approaches using one-vs-one and one-vs-all has gained a significant attention from the research community. They allow to divide multi-class problems into several easier-to-solve two-class sub-problems. In this study we develop an exhaustive empirical analysis to explore the possibility of empowering the one-vs-one scheme for multi-class imbalance classification problems with applying binary ensemble learning approaches. We examine several state-of-the-art ensemble learning methods proposed for addressing the imbalance problems to solve the pairwise tasks derived from the multi-class data set. Then the aggregation strategy is employed to combine the binary ensemble outputs to reconstruct the original multi-class task. We present a detailed experimental study of the proposed approach, supported by the statistical analysis. The results indicate the high effectiveness of ensemble learning with one-vs-one scheme in dealing with the multi-class imbalance classification problems.
更多查看译文
关键词
Multi-class classification,Imbalanced data,Ensemble learning,Binary decomposition,Classifier combination
AI 理解论文
溯源树
样例
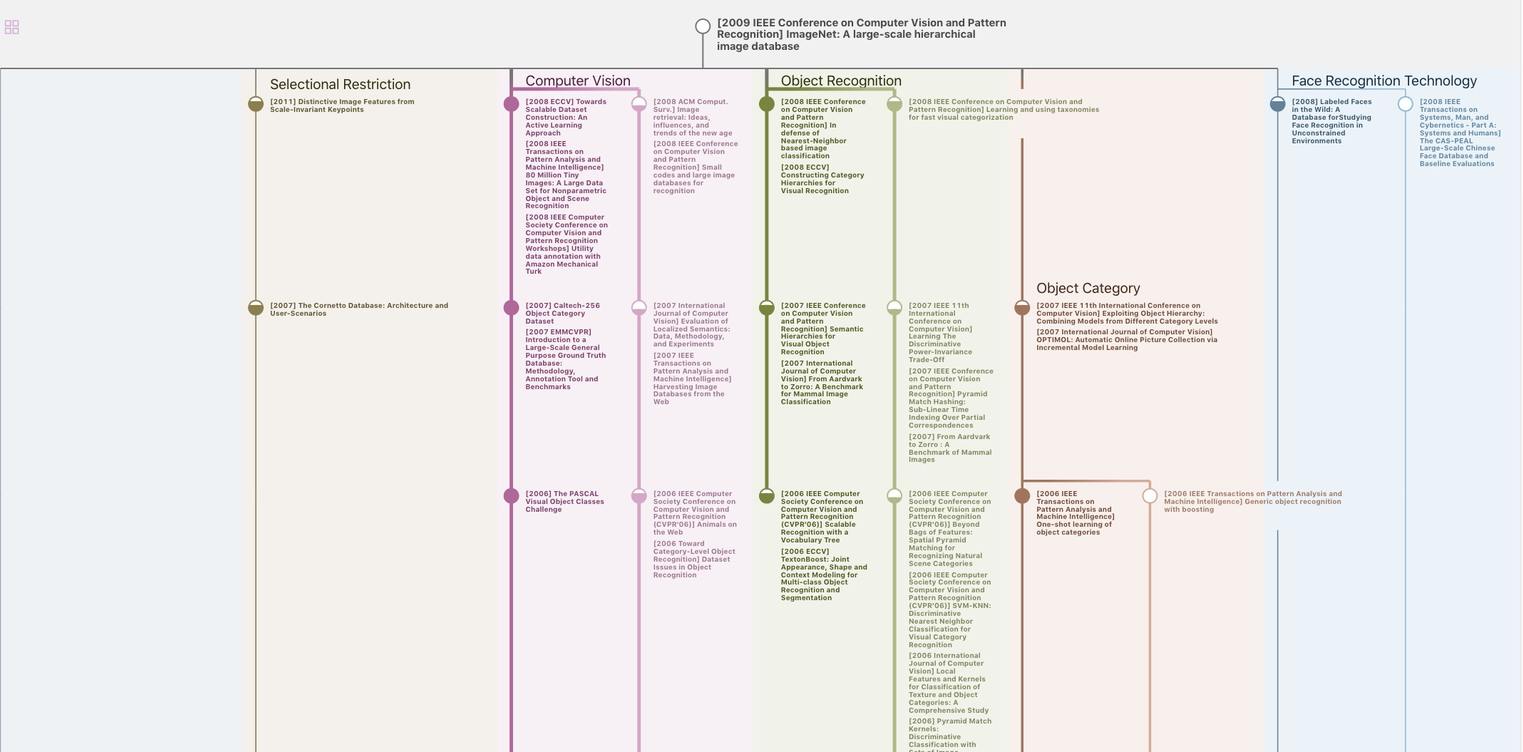
生成溯源树,研究论文发展脉络
Chat Paper
正在生成论文摘要