A Similarity Study Of Interactive Content-Based Image Retrieval Scheme For Classification Of Breast Lesions
IEICE TRANSACTIONS ON INFORMATION AND SYSTEMS(2016)
摘要
To study the similarity between queries and retrieved masses, we design an interactive CBIR ( Content-based Image Retrieval) CADx ( Computer-aided Diagnosis) system using relevance feedback for the characterization of breast masses in ultrasound ( US) images based on radiologists' visual similarity assessment. The CADx system retrieves masses that are similar to query masses from a reference library based on six computer-extracted features that describe the texture, width-to-height, and posterior shadowing of the mass. The k-NN retrieval with Euclidean distance similarity measure and the Rocchio relevance feedback algorithm ( RRF) are used. To train the RRF parameters, the similarities of 1891 image pairs from 62 ( 31 malignant and 31 benign) masses are rated by 3 MQSA ( Mammography Quality Standards Act) radiologists using a 9-point scale ( 9= most similar). The best RRF parameters are chosen based on 3 observer experiments. For testing, 100 independent query masses ( 49 malignant and 51 benign) and 121 reference masses on 230 ( 79 malignant and 151 benign) images were collected. Three radiologists rated the similarity between the query masses and the computer-retrieved masses. Average similarity ratings without and with RRF were 5.39 and 5.64 for the training set and 5.78 and 6.02 for the test set, respectively. Average AUC values without and with RRF were, respectively, 0.86+/- 0.03 and 0.87+/- 0.03 for the training set and 0.91+/- 0.03 and 0.90+/- 0.03 for the test set. On average, masses retrieved using the CBIR system were moderately similar to the query masses based on radiologists' similarity assessments. RRF improved the similarity of the retrieved masses.
更多查看译文
关键词
computer-aided diagnosis, relevance feedback, content-based image retrieval, breast lesion characterization
AI 理解论文
溯源树
样例
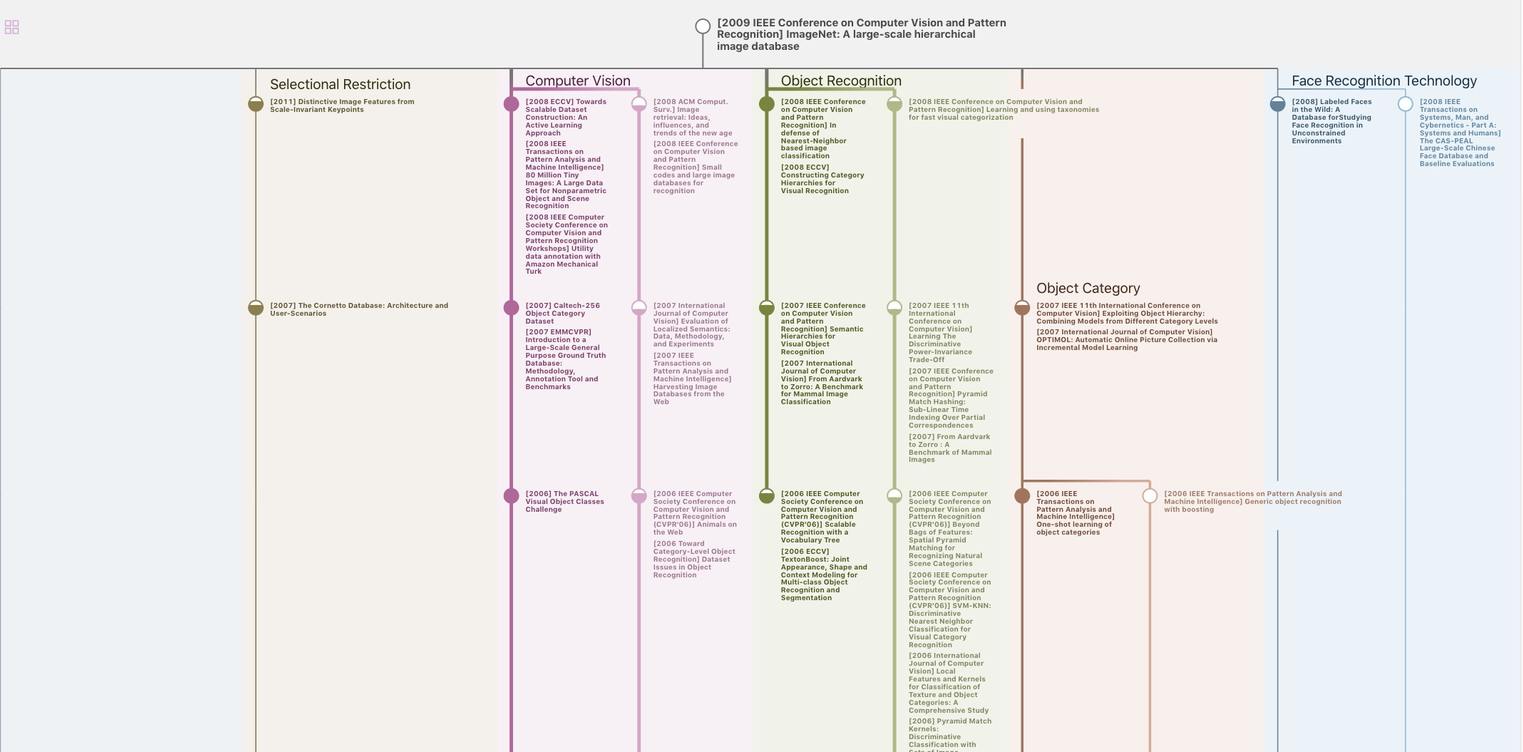
生成溯源树,研究论文发展脉络
Chat Paper
正在生成论文摘要