Deep Appearance Models: A Deep Boltzmann Machine Approach for Face Modeling
International Journal of Computer Vision(2018)
摘要
The “interpretation through synthesis” approach to analyze face images, particularly Active Appearance Models (AAMs) method, has become one of the most successful face modeling approaches over the last two decades. AAM models have ability to represent face images through synthesis using a controllable parameterized Principal Component Analysis (PCA) model. However, the accuracy and robustness of the synthesized faces of AAMs are highly depended on the training sets and inherently on the generalizability of PCA subspaces. This paper presents a novel Deep Appearance Models (DAMs) approach, an efficient replacement for AAMs, to accurately capture both shape and texture of face images under large variations. In this approach, three crucial components represented in hierarchical layers are modeled using the Deep Boltzmann Machines (DBM) to robustly capture the variations of facial shapes and appearances. DAMs are therefore superior to AAMs in inferencing a representation for new face images under various challenging conditions. The proposed approach is evaluated in various applications to demonstrate its robustness and capabilities, i.e. facial super-resolution reconstruction, facial off-angle reconstruction or face frontalization, facial occlusion removal and age estimation using challenging face databases, i.e. Labeled Face Parts in the Wild, Helen and FG-NET. Comparing to AAMs and other deep learning based approaches, the proposed DAMs achieve competitive results in those applications, thus this showed their advantages in handling occlusions, facial representation, and reconstruction.
更多查看译文
关键词
Deep Boltzmann machines,Deep appearance models,Active appearance models,Principal component analysis,Facial super-resolution reconstruction,Facial off-angle reconstruction,Face frontalization,Age estimation
AI 理解论文
溯源树
样例
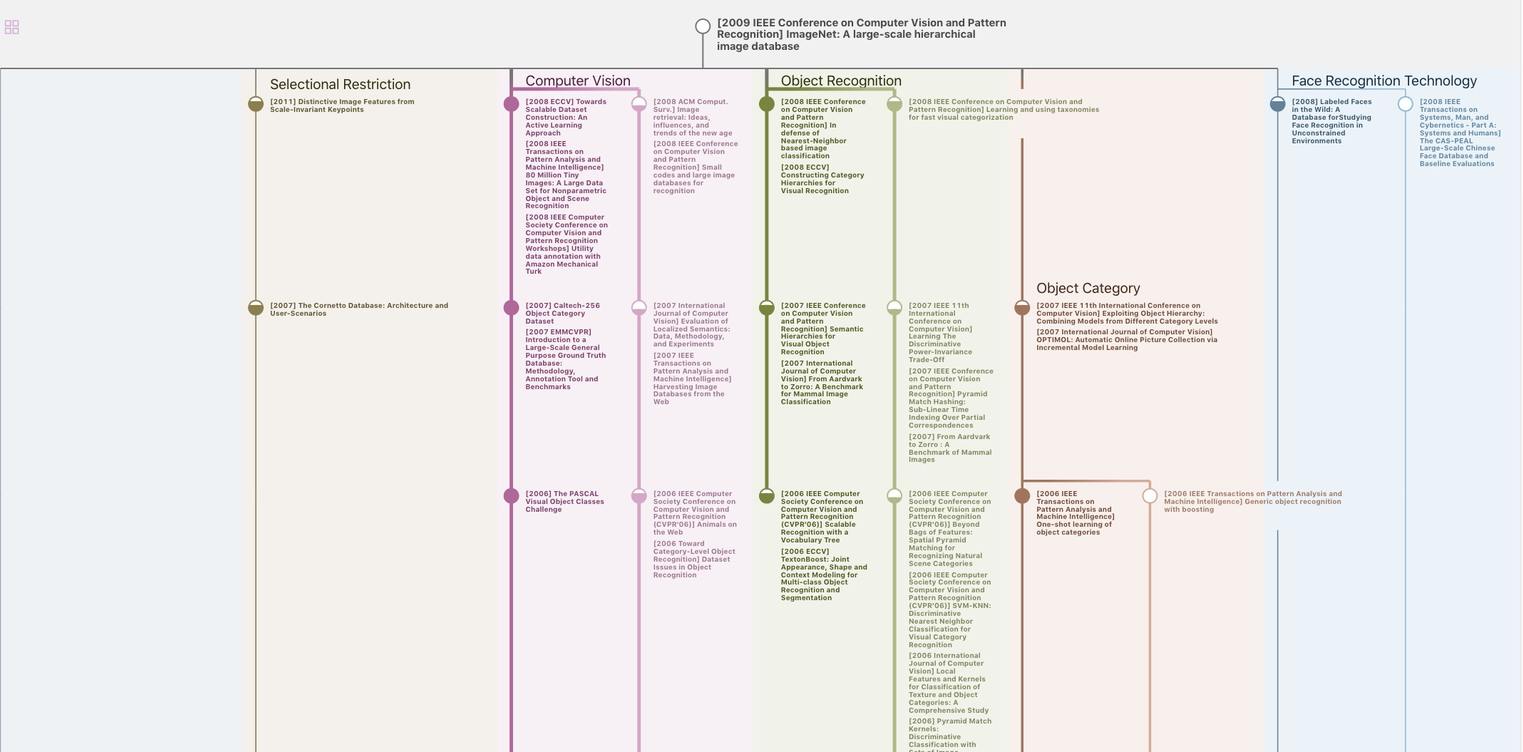
生成溯源树,研究论文发展脉络
Chat Paper
正在生成论文摘要