Sketching with Test Scores and Submodular Maximization.
arXiv: Data Structures and Algorithms(2016)
摘要
We consider the problem of maximizing a submodular set function that can be expressed as the expected value of a function of an $n$-size collection of independent random variables with given prior distributions. This is a combinatorial optimization problem that arises in many applications, including the team selection problem that arises in the context of online labour platforms. We consider a class of approximation algorithms that are restricted to use some statistics of the prior distributions to estimate the outputs of value oracle calls, which can be interpreted as test scores. We establish that the existence of test scores that provide an approximation guarantee is equivalent to that a special type of test scores, so called replication test scores, provide this approximation guarantee. We also identify sufficient conditions for replication test scores to provide this approximation guarantee. These sufficient conditions are shown to hold for a large number of models of production, including a monotone concave function of total production, best-shot, and constant elasticity of substitution production function. We also study a more general submodular welfare maximization problem, which accommodates the case of multiple projects, and derive an $Omega(1/log(k))$-approximation, algorithm where $k$ is the maximum number of participants in a project. We evaluate the quality of approximation provided by test scores in a case study using data from a popular online labour platform for software development.
更多查看译文
AI 理解论文
溯源树
样例
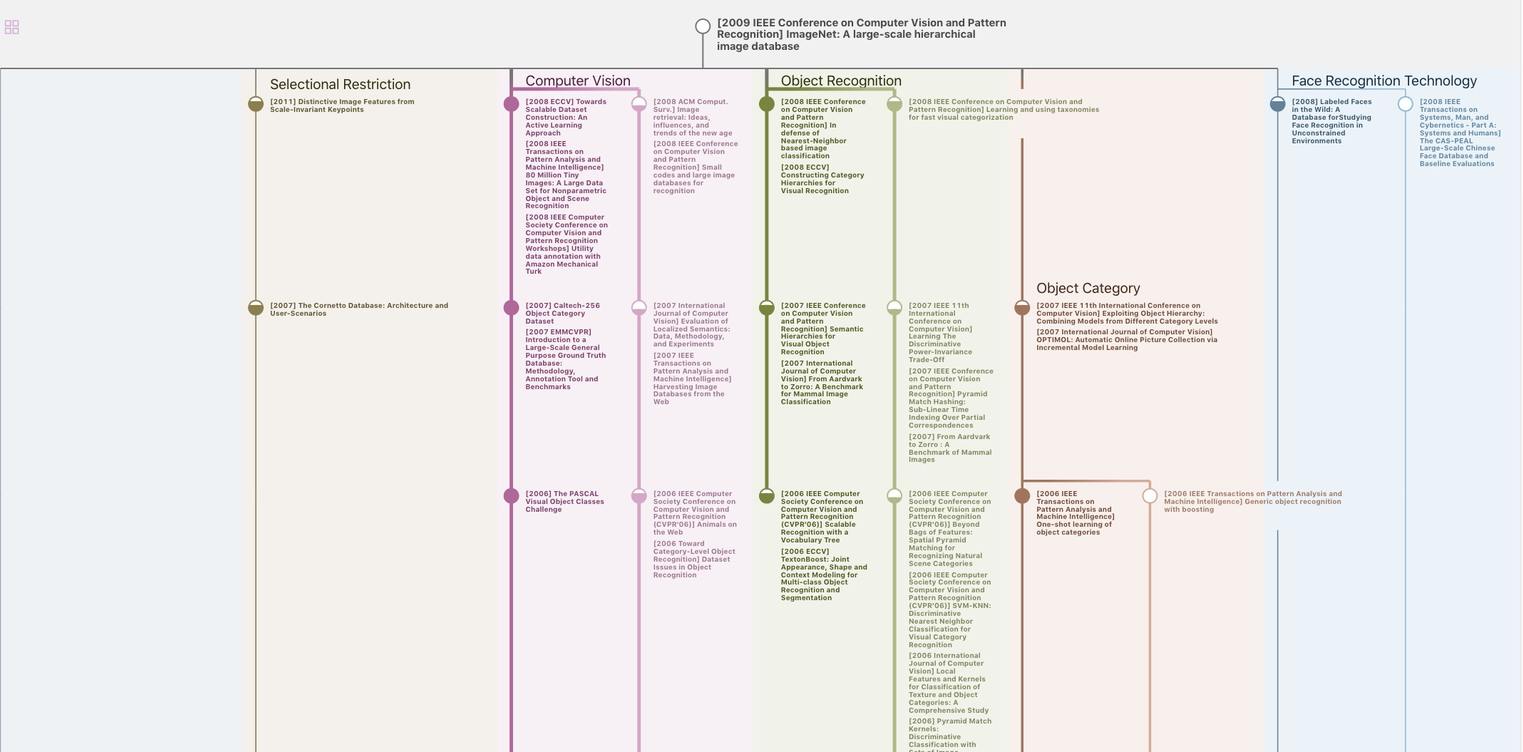
生成溯源树,研究论文发展脉络
Chat Paper
正在生成论文摘要