LOMo: Latent Ordinal Model for Facial Analysis in Videos
2016 IEEE Conference on Computer Vision and Pattern Recognition (CVPR)(2016)
摘要
We study the problem of facial analysis in videos. We propose a novel weakly supervised learning method that models the video event (expression, pain etc.) as a sequence of automatically mined, discriminative sub-events (eg. onset and offset phase for smile, brow lower and cheek raise for pain). The proposed model is inspired by the recent works on Multiple Instance Learning and latent SVM/HCRF- it extends such frameworks to model the ordinal or temporal aspect in the videos, approximately. We obtain consistent improvements over relevant competitive baselines on four challenging and publicly available video based facial analysis datasets for prediction of expression, clinical pain and intent in dyadic conversations. In combination with complimentary features, we report state-of-the-art results on these datasets.
更多查看译文
关键词
LOMo,latent ordinal model,weakly supervised learning,video event modeling,automatically mined discriminative subevents,multiple instance learning,latent SVM/HCRF,video based facial analysis datasets,facial expression prediction,clinical pain,dyadic conversations
AI 理解论文
溯源树
样例
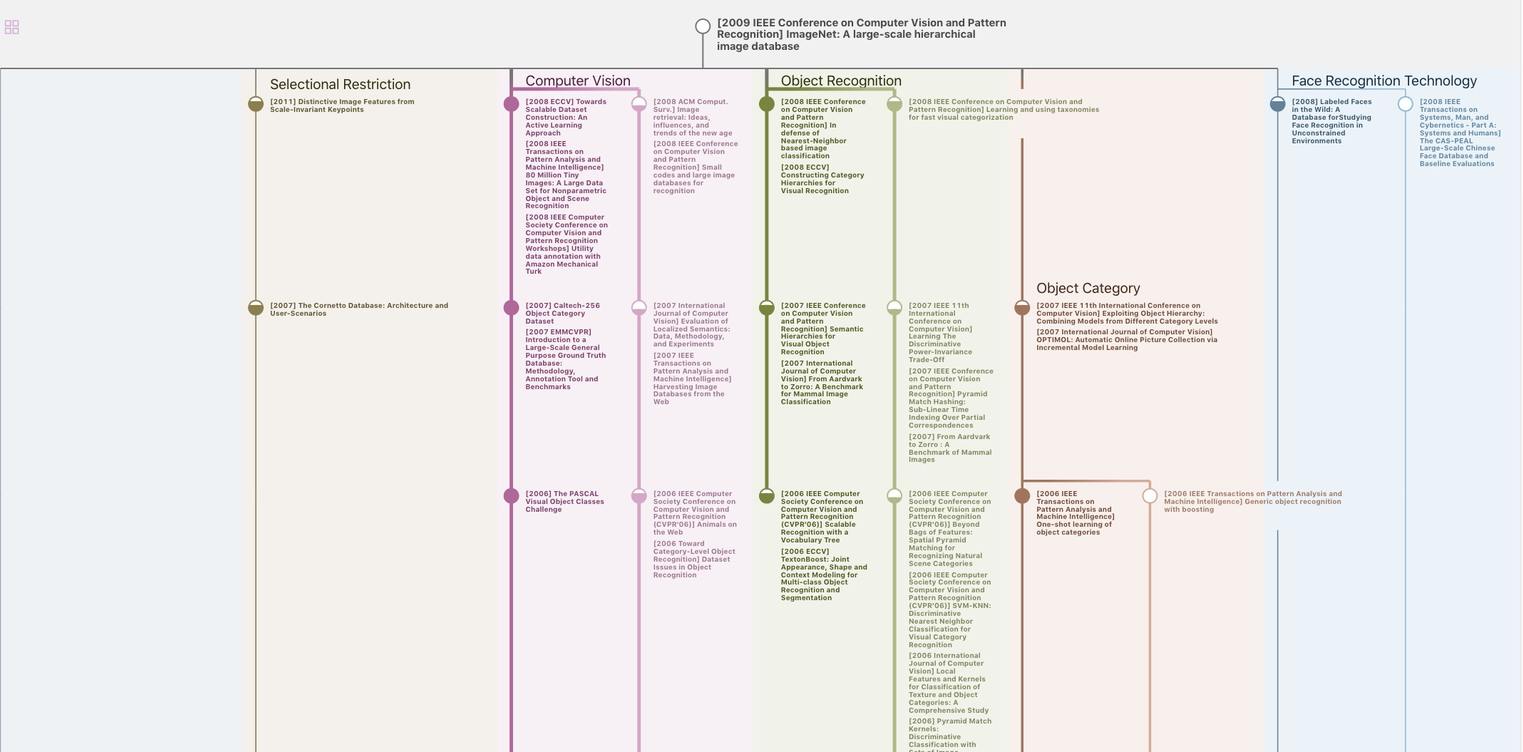
生成溯源树,研究论文发展脉络
Chat Paper
正在生成论文摘要