Scalable motif-aware graph clustering
WWW(2016)
摘要
We develop new methods based on graph motifs for graph clustering, allowing more efficient detection of communities within networks. We focus on triangles within graphs, but our techniques extend to other motifs as well. Our intuition, which has been suggested but not formalized similarly in previous works, is that triangles are a better signature of community than edges. We therefore generalize the notion of conductance for a graph to {\em triangle conductance}, where the edges are weighted according to the number of triangles containing the edge. This methodology allows us to develop variations of several existing clustering techniques, including spectral clustering, that minimize triangles split by the cluster instead of edges cut by the cluster. We provide theoretical results in a planted partition model to demonstrate the potential for triangle conductance in clustering problems. We then show experimentally the effectiveness of our methods to multiple applications in machine learning and graph mining.
更多查看译文
关键词
Graph Clustering,Large-scale Graph Mining,Expanders,Community detection
AI 理解论文
溯源树
样例
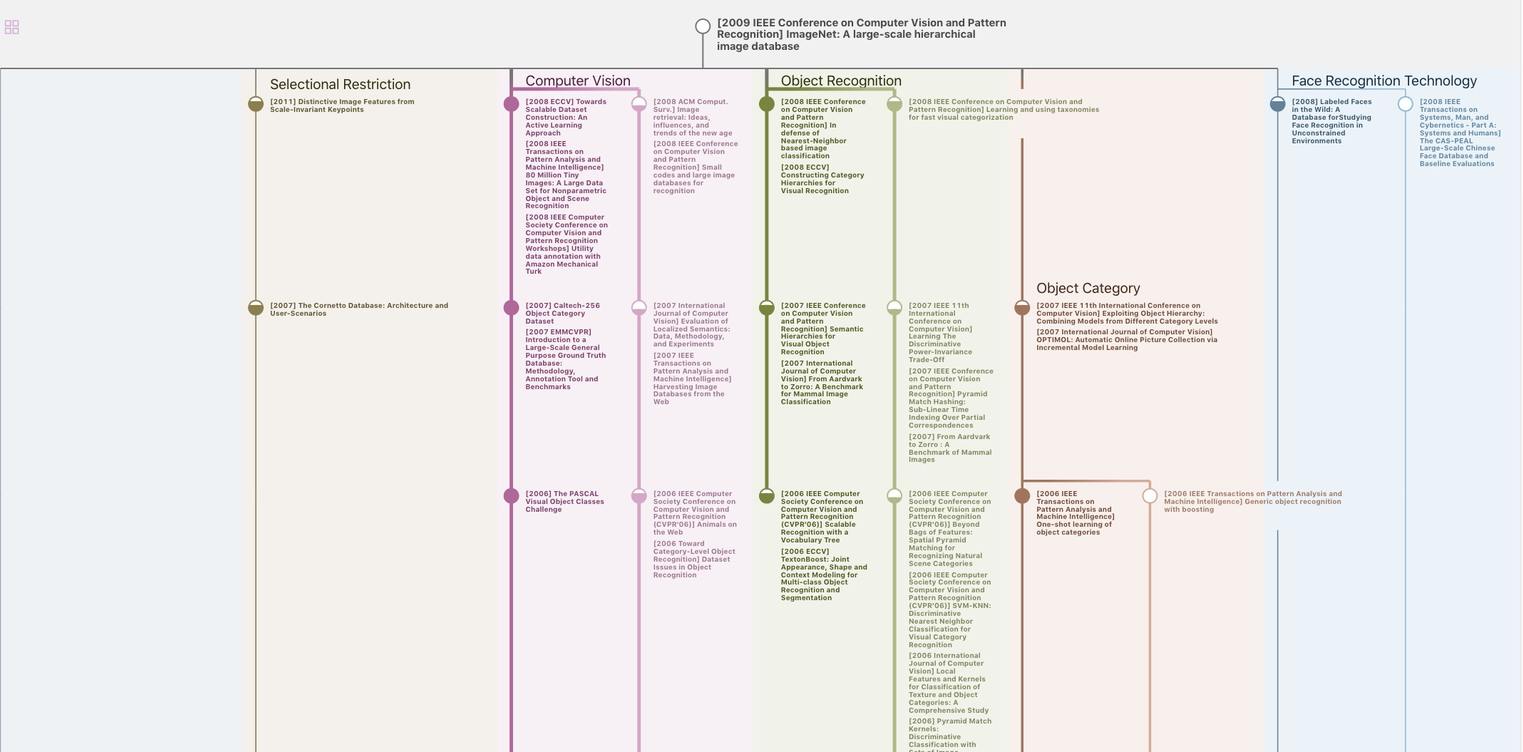
生成溯源树,研究论文发展脉络
Chat Paper
正在生成论文摘要