Learning Sparse Additive Models with Interactions in High Dimensions.
JMLR Workshop and Conference Proceedings(2016)
摘要
A function f : R-d -> R is referred to as a Sparse Additive Model (SPAM), if it is of the form f(x) = Sigma(l is an element of S) phi(l)(x(l)), where S subset of [d], |S| << d. Assuming phi(l)'s and S to be unknown, the problem of estimating f from its samples has been studied extensively. In this work, we consider a generalized SPAM, allowing for second order interaction terms. For some S-1 subset of [d], S-2 subset of (([d])(2)) , the function f is assumed to be of the form: f(x) = Sigma(p is an element of S1) phi(p)(x(p)) + Sigma((l,l')subset of S2) phi((l,l')) (x(l), x(l')). Assuming phi(p), phi((l,l')), S-1 and, S-2 to be unknown, we provide a randomized algorithm that queries f and exactly recovers S-1, S-2. Consequently, this also enables us to estimate the underlying phi(p), phi((l,l')). We derive sample complexity bounds for our scheme and also extend our analysis to include the situation where the queries are corrupted with noise - either stochastic, or arbitrary but bounded. Lastly, we provide simulation results on synthetic data, that validate our theoretical findings.
更多查看译文
AI 理解论文
溯源树
样例
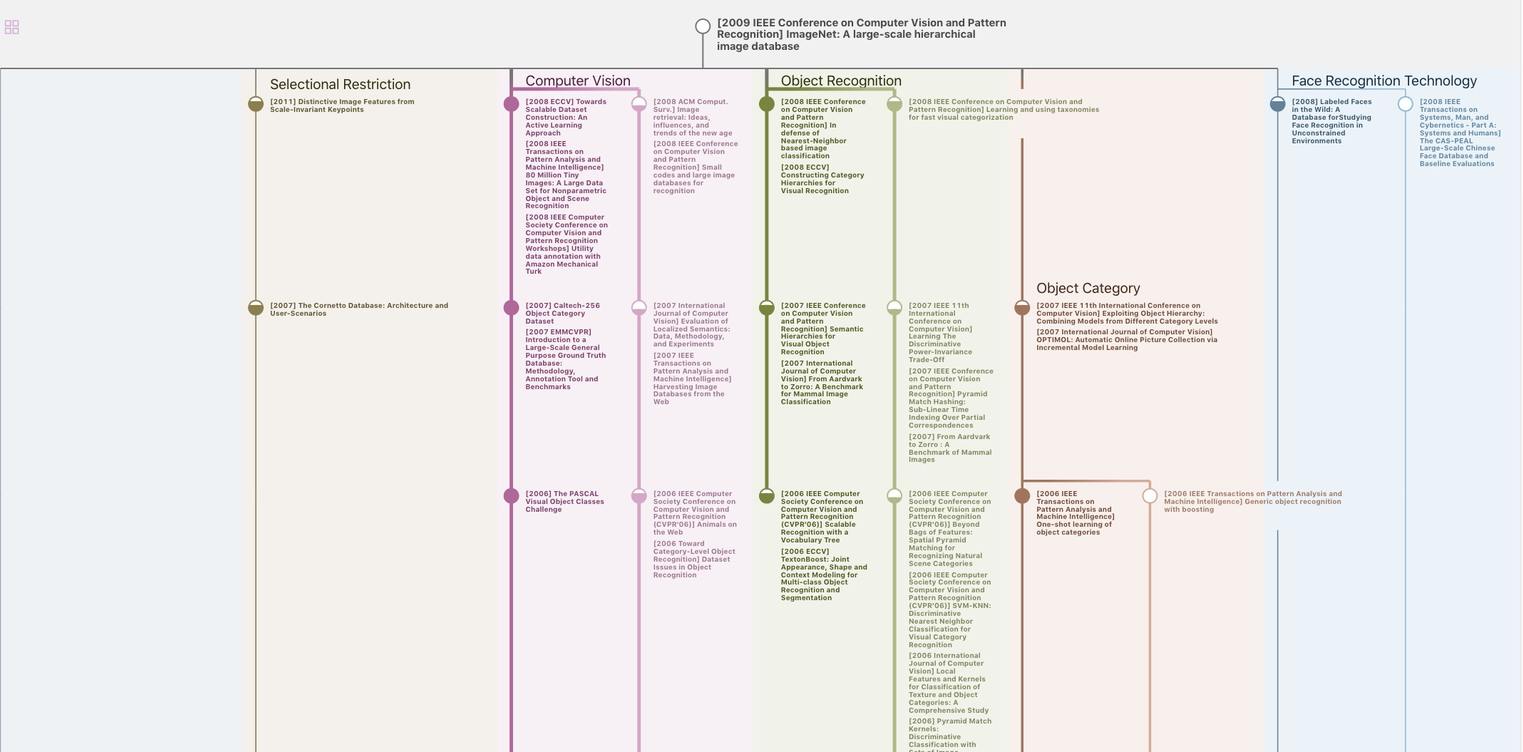
生成溯源树,研究论文发展脉络
Chat Paper
正在生成论文摘要