The Bayesian Linear Information Filtering Problem
2016 IEEE 28th International Conference on Tools with Artificial Intelligence (ICTAI)(2016)
摘要
We present a Bayesian sequential decision-making formulation of the information filtering problem, in which an algorithm presents items (news articles, scientific papers, tweets) arriving in a stream, and learns relevance from user feedback on presented items. We model user preferences using a Bayesian linear model, similar in spirit to a Bayesian linear bandit. We compute a computational upper bound on the value of the optimal policy, which allows computing an optimality gap for implementable policies. We then use this analysis as motivation in introducing a pair of new Decompose-Then-Decide (DTD) heuristic policies, DTD-Dynamic-Programming(DTD-DP) and DTD-Upper-Confidence-Bound (DTD-UCB). We compare DTD-DP and DTD-UCB against several benchmarks on real and simulated data, demonstrating significant improvement, and show that the achieved performance is close to the upper bound.
更多查看译文
关键词
exploration vs. exploitation,information filtering,Bayesian statistics,dynamic programming,linear bandit
AI 理解论文
溯源树
样例
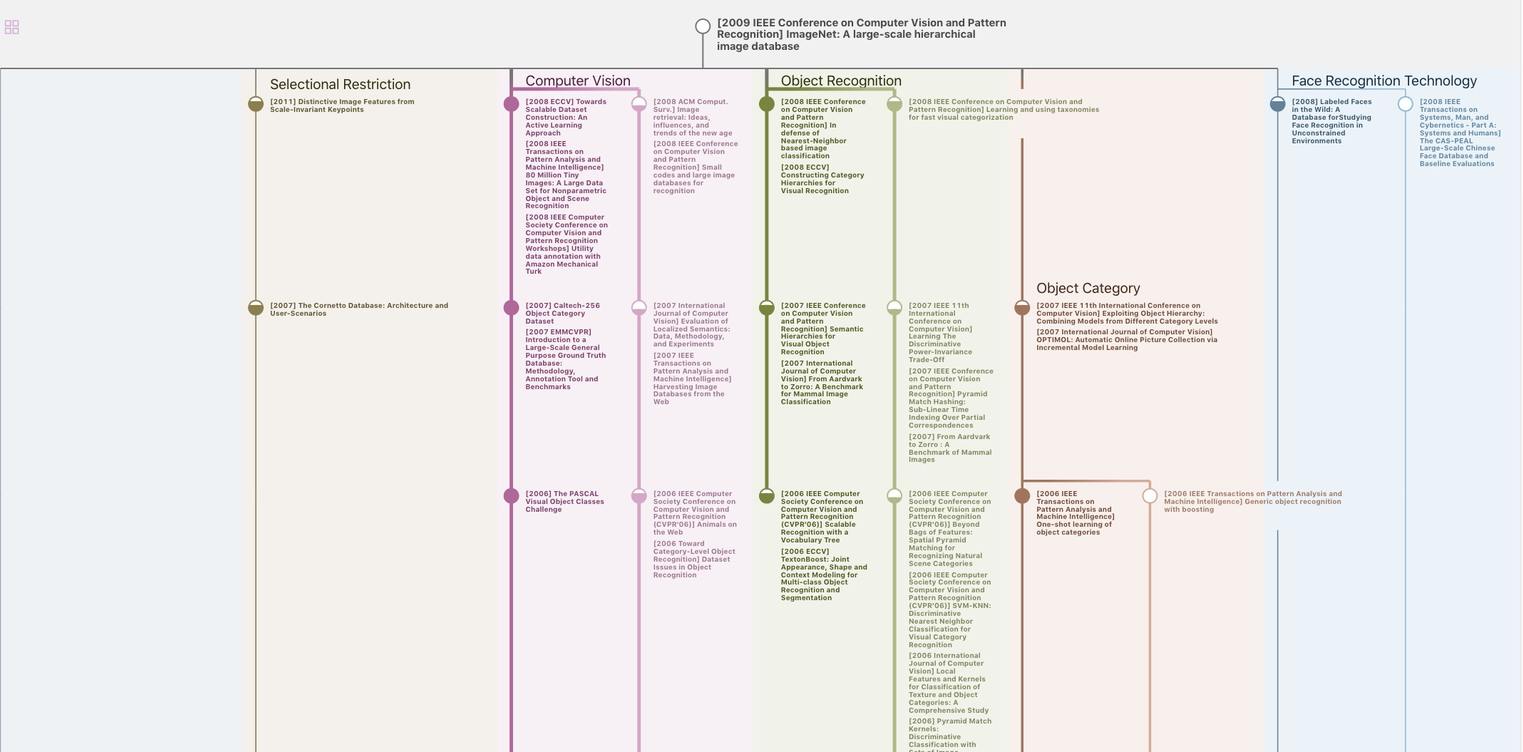
生成溯源树,研究论文发展脉络
Chat Paper
正在生成论文摘要