Computational Methods For Solving Nonconvex Block-Separable Constrained Quadratic Problems
SIAM Journal on Optimization(2016)
摘要
Nonconvex quadratically constrained quadratic programs (QCQPs) with block-separable convex constraints are generally NP-hard. These kinds of problems appear in many applications such as estimation and control, complex unimodular programming, and MAX-CUT type problems. Semidefinite relaxation is the best known upper bound approximation for QCQP with block-separable constraints. We suggest the block optimal descent (BOD) algorithm to obtain a lower approximation. We show that this algorithm utilizes block hidden convexity to apply block alternating minimization and has a sublinear rate of convergence. An improved approximation is obtained by using a novel approach, Lagrange guided descent (LGD), which finds a "good" initial point based on the semidefinite programming (SDP) relaxation solution. A quantitative study shows the LGD has superior performance over BOD.
更多查看译文
关键词
quadratic programming,nonconvex optimization,semidefinite programming,approximation methods and heuristics
AI 理解论文
溯源树
样例
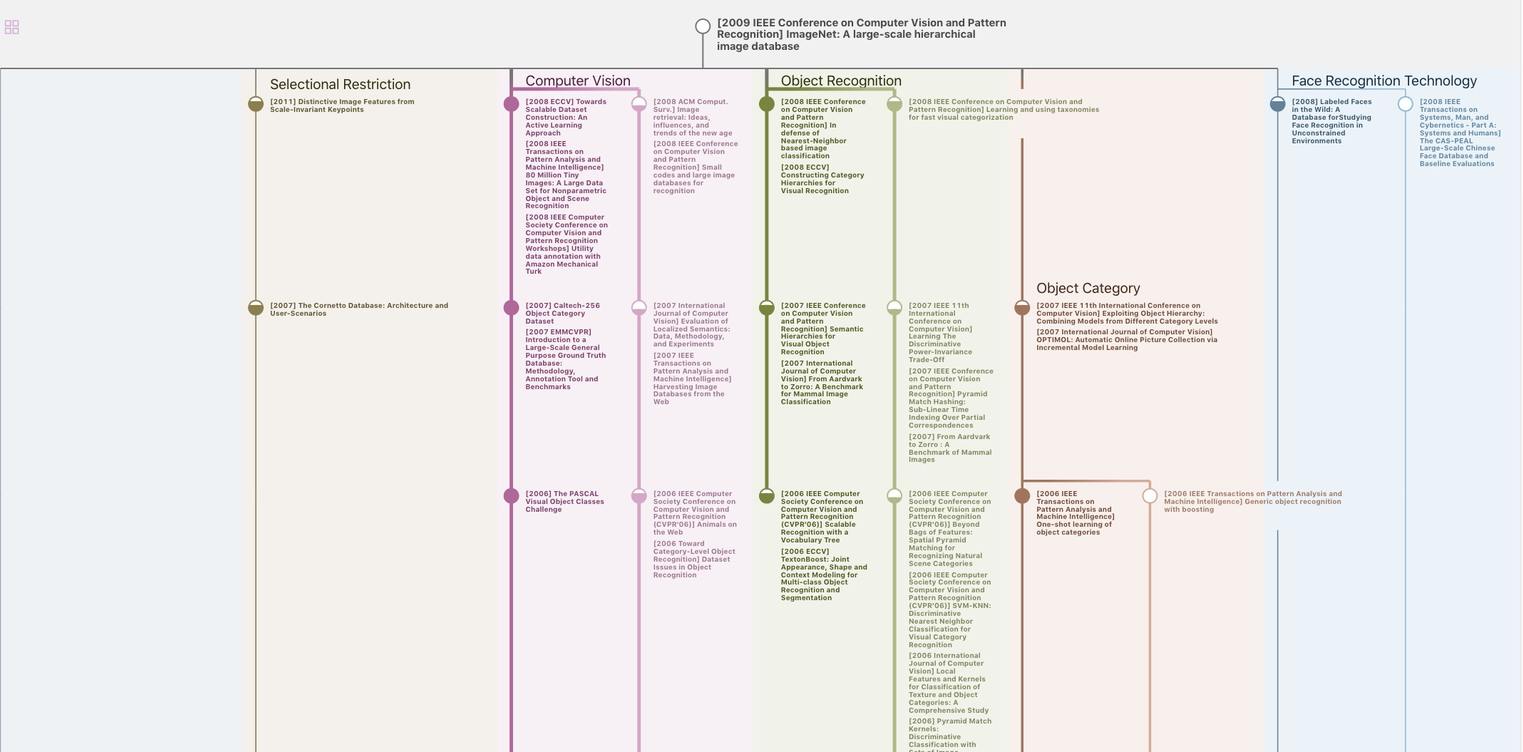
生成溯源树,研究论文发展脉络
Chat Paper
正在生成论文摘要