Double Articulation Analyzer with Deep Sparse Autoencoder for Unsupervised Word Discovery from Speech Signals
Advanced robotics(2016)
摘要
Direct word discovery from audio speech signals is a very difficult and challenging problem for a developmental robot. Human infants are able to discover words directly from speech signals, and, to understand human infants' developmental capability using a constructive approach, it is very important to build a machine learning system that can acquire knowledge about words and phonemes, i.e. a language model and an acoustic model, autonomously in an unsupervised manner. To achieve this, the nonparametric Bayesian double articulation analyzer (NPB-DAA) with the deep sparse autoencoder (DSAE) is proposed in this paper. The NPB-DAA has been proposed to achieve totally unsupervised direct word discovery from speech signals. However, the performance was still unsatisfactory, although it outperformed pre-existing unsupervised learning methods. In this paper, we integrate the NPB-DAA with the DSAE, which is a neural network model that can be trained in an unsupervised manner, and demonstrate its performance through an experiment about direct word discovery from auditory speech signals. The experiment shows that the combined method, the NPB-DAA with the DSAE, outperforms pre-existing unsupervised learning methods, and shows state-of-the-art performance. It is also shown that the proposed method outperforms several standard speech recognizer-based methods with true word dictionaries.
更多查看译文
关键词
Bayesian nonparametrics,deep learning,speech recognition,unsupervised learning,word discovery
AI 理解论文
溯源树
样例
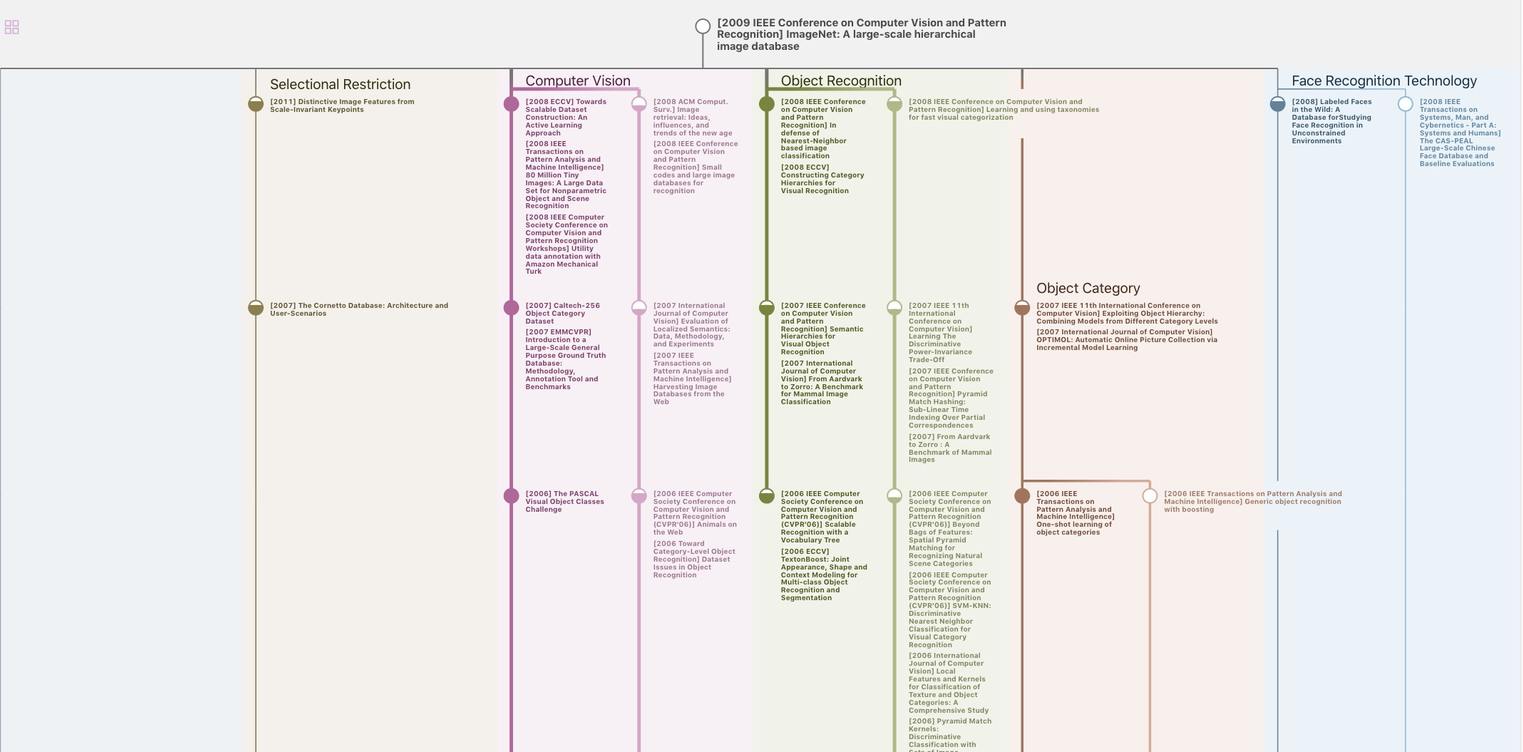
生成溯源树,研究论文发展脉络
Chat Paper
正在生成论文摘要