Direct Density Derivative Estimation.
Neural Computation(2016)
摘要
Estimating the derivatives of probability density functions is an essential step in statistical data analysis. A naive approach to estimate the derivatives is to first perform density estimation and then compute its derivatives. However, this approach can be unreliable because a good density estimator does not necessarily mean a good density derivative estimator. To cope with this problem, in this letter, we propose a novel method that directly estimates density derivatives without going through density estimation. The proposed method provides computationally efficient estimation for the derivatives of any order on multidimensional data with a hyperparameter tuning method and achieves the optimal parametric convergence rate. We further discuss an extension of the proposed method by applying regularized multitask learning and a general framework for density derivative estimation based on Bregman divergences. Applications of the proposed method to nonparametric Kullback-Leibler divergence approximation and bandwidth matrix selection in kernel density estimation are also explored.
更多查看译文
AI 理解论文
溯源树
样例
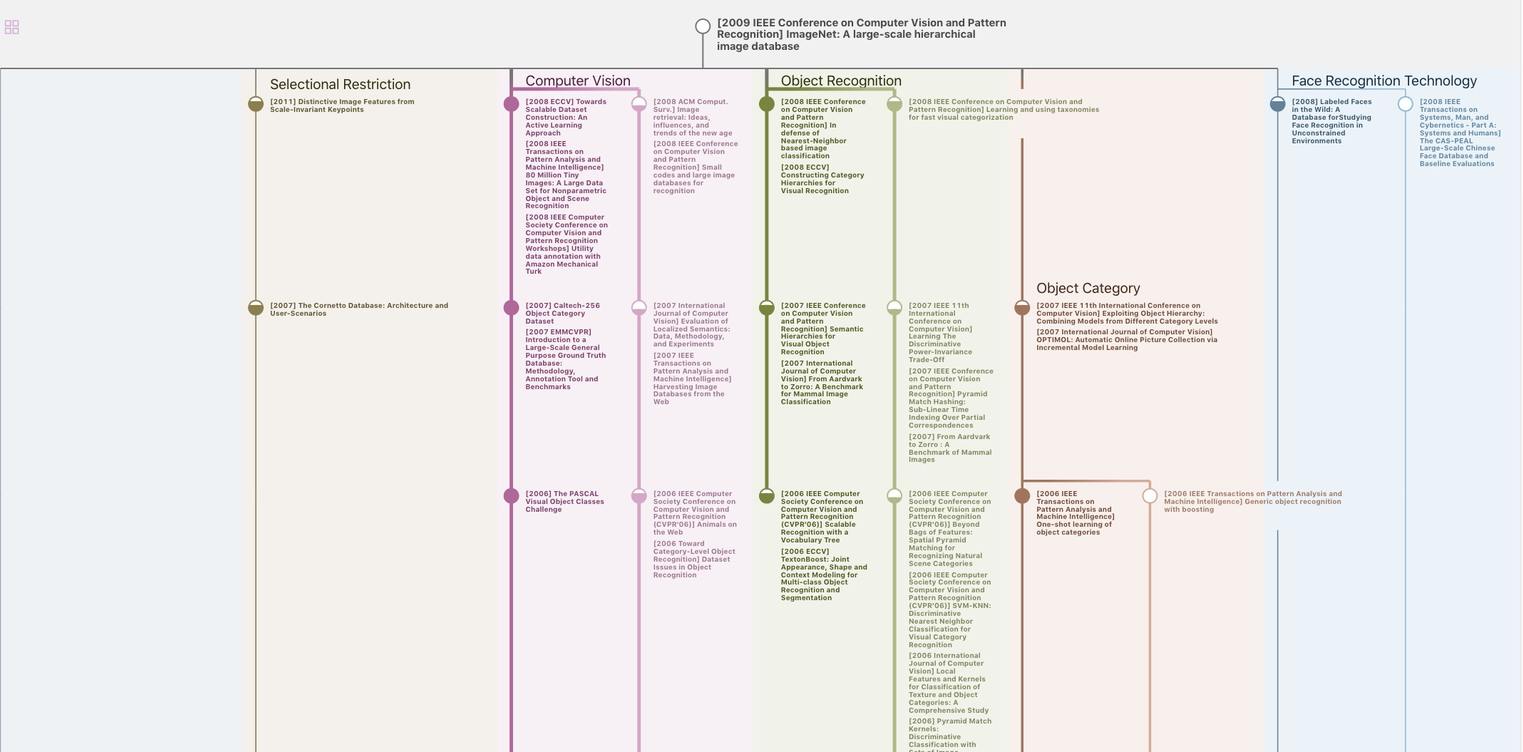
生成溯源树,研究论文发展脉络
Chat Paper
正在生成论文摘要