A Gaussian mixture model based discretization algorithm for associative classification of medical data.
Expert Syst. Appl.(2016)
摘要
A new supervised discretization algorithm is proposed.Multi-modal distributed numerical variables/features are particularly handled.The proposed approach outperforms existing algorithms in rule-based classification. Knowledge-based systems such as expert systems are of particular interest in medical applications as extracted if-then rules can provide interpretable results. Various rule induction algorithms have been proposed to effectively extract knowledge from data, and they can be combined with classification methods to form rule-based classifiers. However, most of the rule-based classifiers can not directly handle numerical data such as blood pressure. A data preprocessing step called discretization is required to convert such numerical data into a categorical format. Existing discretization algorithms do not take into account the multimodal class densities of numerical variables in datasets, which may degrade the performance of rule-based classifiers. In this paper, a new Gaussian Mixture Model based Discretization Algorithm (GMBD) is proposed that preserve the most frequent patterns of the original dataset by taking into account the multimodal distribution of the numerical variables. The effectiveness of GMBD algorithm was verified using six publicly available medical datasets. According to the experimental results, the GMBD algorithm outperformed five other static discretization methods in terms of the number of generated rules and classification accuracy in the associative classification algorithm. Consequently, our proposed approach has a potential to enhance the performance of rule-based classifiers used in clinical expert systems.
更多查看译文
关键词
Discretization,Gaussian mixture model,Associative classification,Medical informatics,Clinical decision support system
AI 理解论文
溯源树
样例
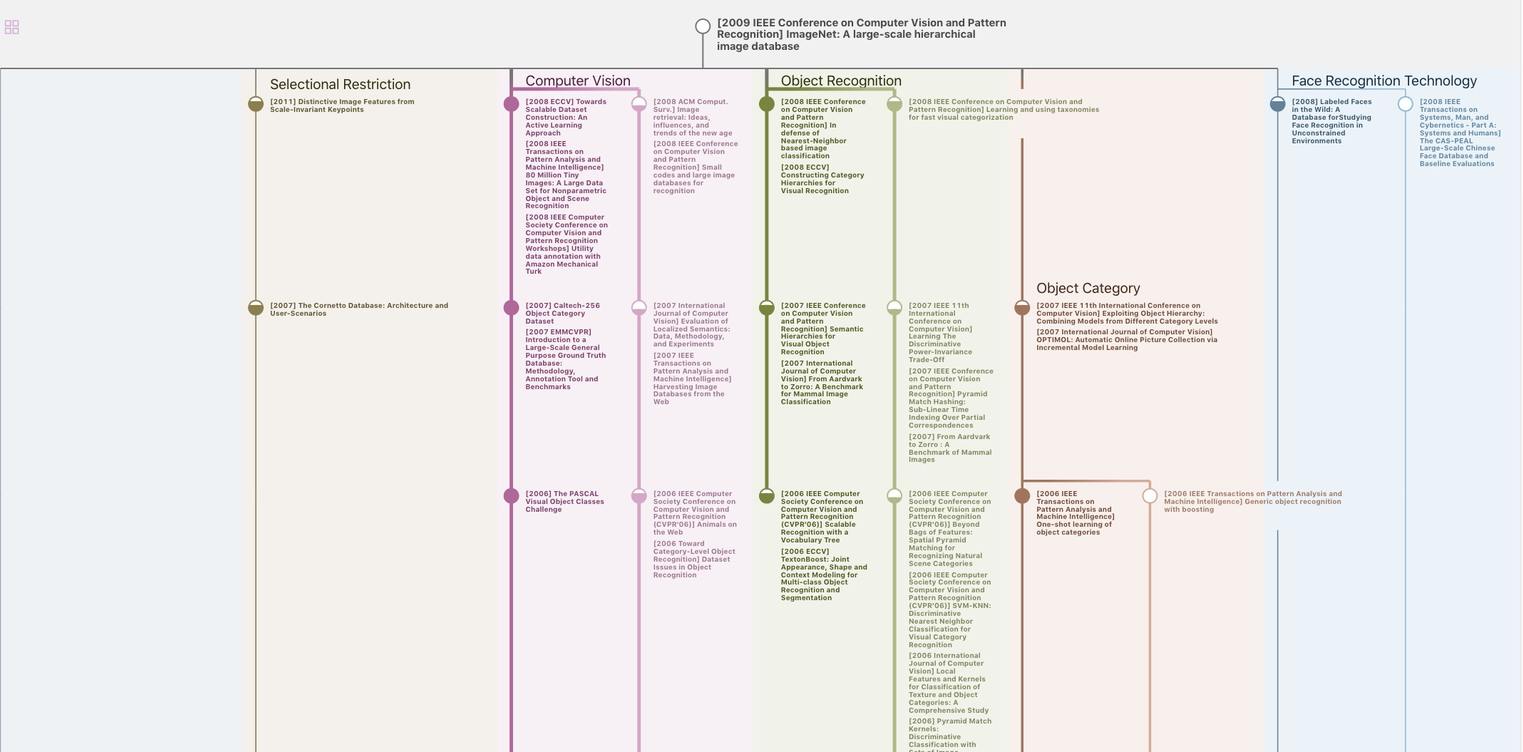
生成溯源树,研究论文发展脉络
Chat Paper
正在生成论文摘要