Accelerated Stochastic Block Coordinate Descent with Optimal Sampling
KDD(2016)
摘要
We study the composite minimization problem where the objective function is the sum of two convex functions: one is the sum of a finite number of strongly convex and smooth functions, and the other is a general convex function that is non-differentiable. Specifically, we consider the case where the non-differentiable function is block separable and admits a simple proximal mapping for each block. This type of composite optimization is common in many data mining and machine learning problems, and can be solved by block coordinate descent algorithms. We propose an accelerated stochastic block coordinate descent (ASBCD) algorithm, which incorporates the incrementally averaged partial derivative into the stochastic partial derivative and exploits optimal sampling. We prove that ASBCD attains a linear rate of convergence. In contrast to uniform sampling, we reveal that the optimal non-uniform sampling can be employed to achieve a lower iteration complexity. Experimental results on different large-scale real data sets support our theory.
更多查看译文
关键词
Stochastic block coordinate descent,Sampling
AI 理解论文
溯源树
样例
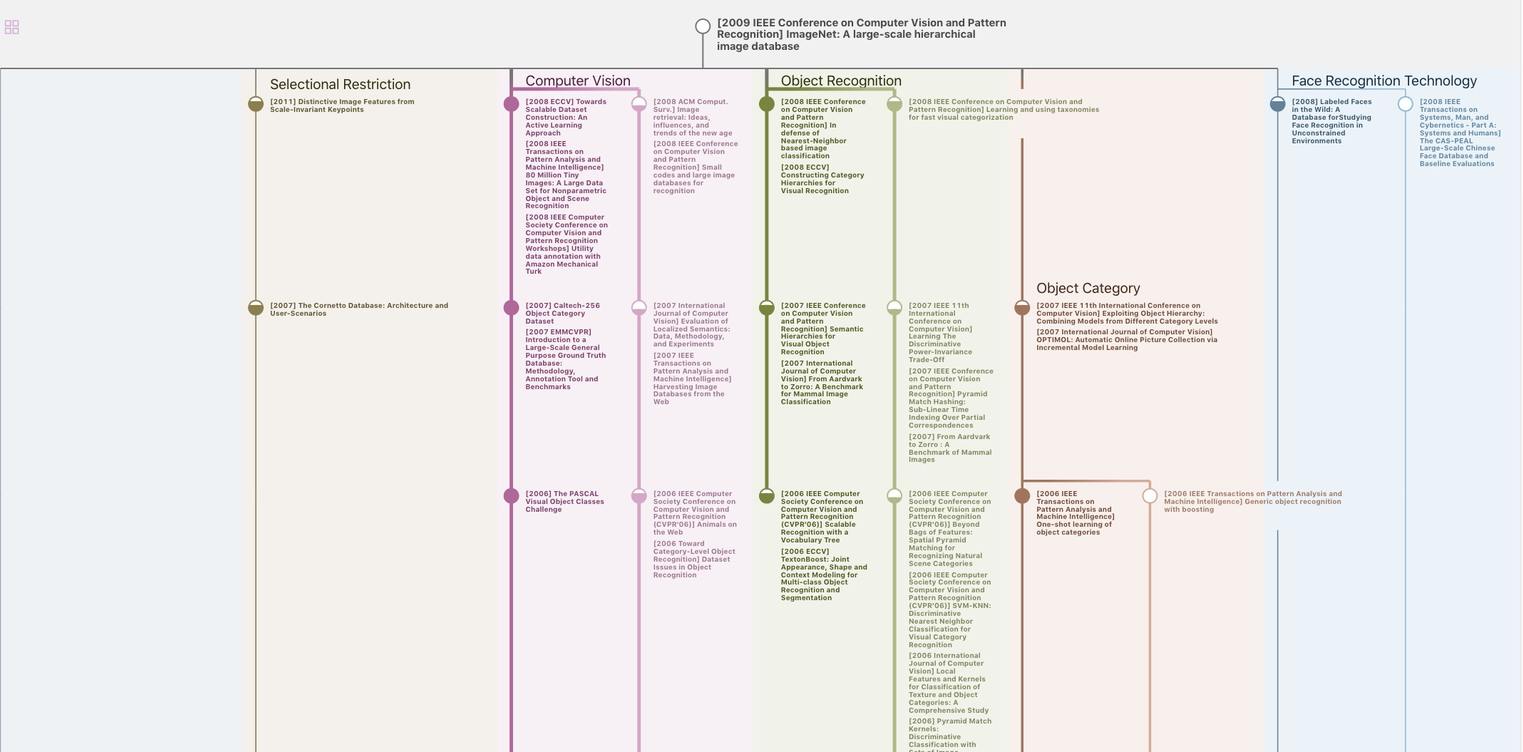
生成溯源树,研究论文发展脉络
Chat Paper
正在生成论文摘要