Schatten p-norm based principal component analysis.
Neurocomputing(2016)
摘要
Structured sparse PCA (SSPCA) is a new emerging method regularized by structured sparsity-inducing norms. However, these regularization terms are not necessarily optimal because of the noisy and irrelevant features embedded in predefined patterns. This paper presents a method called Schatten p-norm based principal component analysis (S(p)PCA) to learn interpretable and structured elements (or factors). In S(p)PCA, a low-rank assumption is used to characterize structured elements in a two-dimensional matrix form. Compared to SSPCA, the low-rank assumption of S(p)PCA is more intuitive and effective for describing object parts of an image. Moreover, S(p)PCA can deal with some scenarios, where the dictionary element matrixes have complex structures. We also propose an efficient and simple optimization procedure to solve the problem. Extensive experiments on denoising of sparse structured signals and face recognition on different databases (e.g. AR, Extend Yale B and Multi-PIE) demonstrate the superior performance over some recently proposed methods. (C) 2016 Elsevier B.V. All rights reserved.
更多查看译文
关键词
SPARSE REPRESENTATION,MATRIX DECOMPOSITION,RECOVERY,MINIMIZATION,RECOGNITION,COMPLETION
AI 理解论文
溯源树
样例
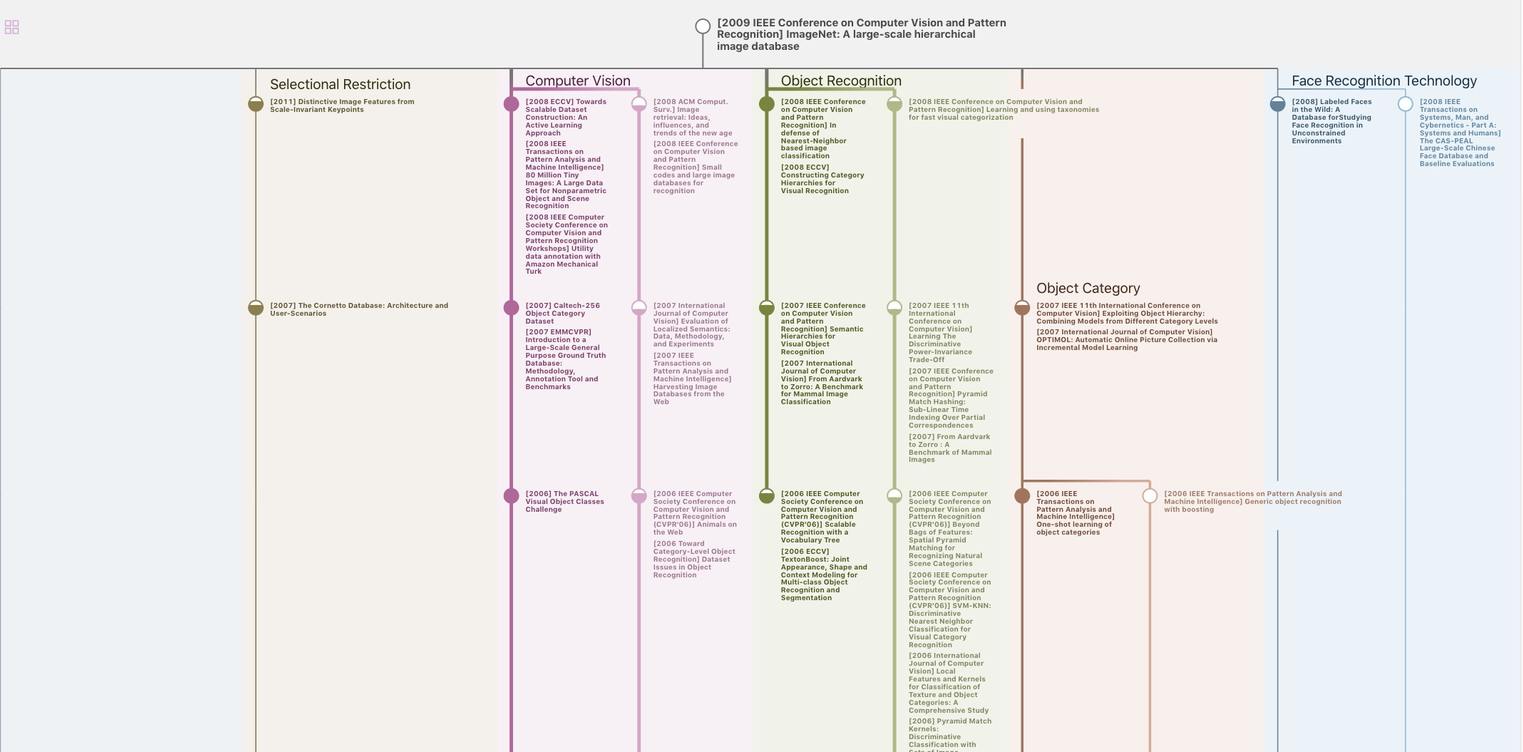
生成溯源树,研究论文发展脉络
Chat Paper
正在生成论文摘要